科技业下一波浪潮:当大数据遇上生物学
去年12月,CVS提出以690亿美元收购安进保险公司。今年1月,又有三大巨头——亚马逊、摩根大通和伯克谢尔哈撒韦表示要成立合资公司,降低共100万左右员工的医疗成本并提高效果。之后3月,信诺保险表示将出资超过500亿美元收购医药福利管理机构Express Scripts。 为何近来医疗领域交易如此热闹?猛一看你可能以为是为了追求量级。也就是管理学中常说的“规模”。但实际上有个强大的催化剂,一种无限大又无限小的东西似乎能回答所有问题。答案就是数据。 更确切地说,是你的数据:个体生物信息、病史、健康不断波动的情况,以及去过何处、消费习惯、睡眠状况,饮食和排泄等等。日常生活中产生的数据,实验室检测结果、医学影像、基因档案、液体活检、心电图……这些都只是一小部分,但已涵盖大量数据。再加上医疗索赔、临床实验、处方和学术研究等等,每天产生的数据约750万亿字节,约占全世界数据量的30%。 海量数据已存在许久,多亏现在层出不穷的新科技,先进的测量仪器,无处不在的连接和云技术,再加上人工智能,企业终于可以利用这些数据。“收集数据只是一方面。”斯克利普斯研究所主任埃里克·托普尔表示。“但更重要的是分析。就在三五年前,数据还只能闲置,现在已经可以分析解读。这是医疗领域最大的变化。” |
It Began in December, with CVS’s proposed $69 billion buyout of insurer Aetna. In January, three more corporate behemoths—Amazon JPMorgan Chase , and Berkshire Hathaway —said they were forming a joint venture aimed at reducing health care costs and improving outcomes for their combined 1 million or so employees. Then, in March, Cigna said it would buy pharmacy benefits manager Express Scripts for more than $50 billion. What’s driving this frenzy of health care–related dealmaking? On first glance you might think it’s merely the pursuit of mass itself. Of “scale,” as management types like to say. But in truth, there’s a more powerful catalyst—one so gargantuan and infinitesimal at the same time that it sounds like the answer to a riddle. And that’s data. More specifically, it’s your data: your individual biology, your health history and ever-fluctuating state of well-being, where you go, what you spend, how you sleep, what you put in your body and what comes out. The amount of data you slough off everyday—in lab tests, medical images, genetic profiles, liquid biopsies, electrocardiograms, to name just a few—is overwhelming by itself. Throw in the stuff from medical claims, clinical trials, prescriptions, academic research, and more, and the yield is something on the order of 750 quadrillion bytes every day—or some 30% of the world’s data production. These massive storehouses of information have always been there. But now, thanks to a slew of novel technologies, sophisticated measuring devices, ubiquitous connectivity and the cloud, and yes, artificial intelligence, companies can harness and make sense of this data as never before. “It’s not the data,” says Eric Topol, director of the Scripps Translational Science Institute. “It’s the analytics. Up until three-to-five years ago, all that data was just sitting there. Now it’s being analyzed and interpreted. It’s the most radical change happening in health care.” |
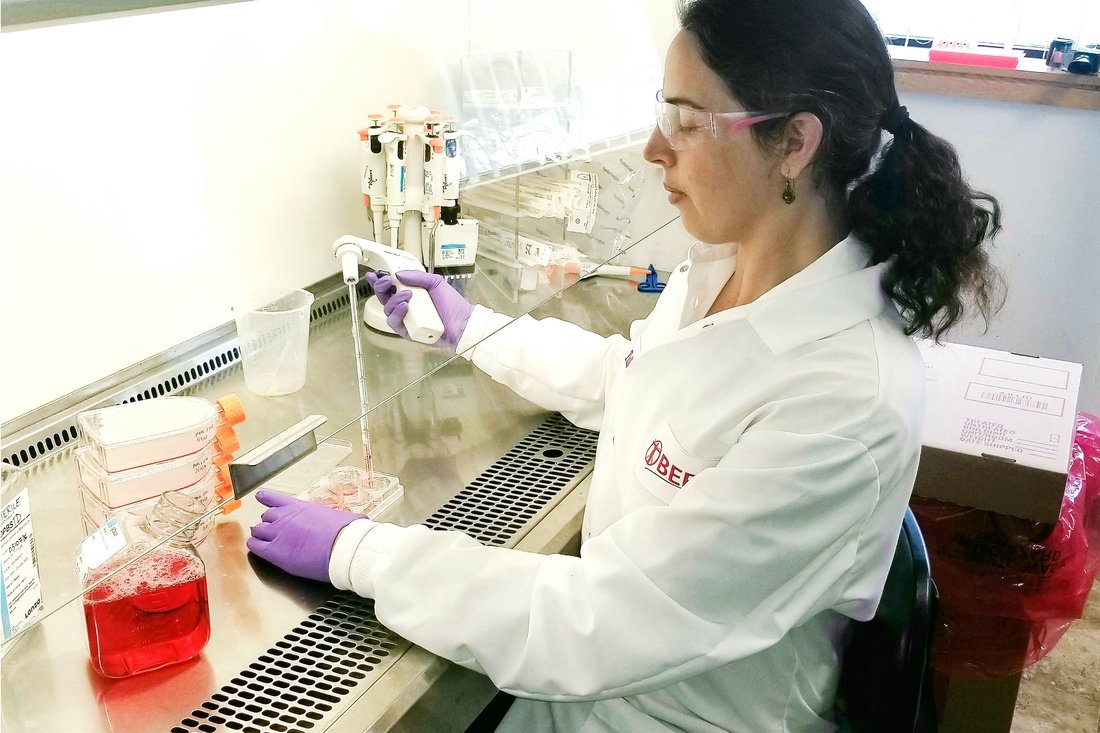
因此,获取、分析并利用数据已成为新的淘金潮。一群科技大鳄,还有一帮热门初创公司都在积极试水。 谷歌母公司Alphabet旗下的生物科技公司Verily就在追踪10000名志愿者提供的生物特征信息,努力制定人类健康“基准”(流言称其对医疗保险行业也有兴趣)。苹果刚宣布了iPhone上的新功能,可以立即对接几家大医疗系统,上传医疗数据,此外苹果还与斯坦福大学进行心脏领域研究,测试可穿戴设备是否能检测严重的心脏疾病。 根据医疗保险和医疗补助服务中心的数据,合理利用数据最终可以提升患者健康水平并降低医疗成本,仅2018年医疗成本就预计增长5.3%。 当然,这是远期理想。但最起码能带动潜在的相关业务。BDO董事总经理大卫·弗兰德指出,坐享海量数据的Facebook和谷歌通过广告赚钱,他估计相关业务价值2000亿美元。“医疗行业规模要大15倍,”他表示。“医疗支出达3万亿美元。理论上说,如果路走对了就能打造15个Facebook和15个谷歌。这就是竞争激烈的原因。” 也正因如此,从医院到保险公司到福利管理机构,再到医疗和仪器制造商,如此多传统医疗公司都在急切地重组创新,要知道相关行业体量可达经济总量五分之一。行业重组不仅意味着企业层面重新划定医疗行业版图,也会影响到每个人。 埃森哲首席技术和创新官保罗·多尔蒂比较乐观,他估计由于“信息不对称”存在,掌握自身生物数据的病人会从中受益,享受更多红利。 为了更好地了解未来如何实现权力均衡,也为了更深入探究目前的情况,《财富》杂志采访了三十几位涉及医疗行业各细分领域的高管,以及企业家、医生、病人和其他专家。以下就是大数据革命在促进医药行业方面的各种说法。 数据药丸:病患诊断新范式 雅各比八岁生日前后,林德赛·阿莫斯开始注意儿子的异常。雅各比很活泼,经常打冰球和长曲棍球,但突然变得有些懒洋洋,而且总想去洗手间。医生测量雅各比的血糖后,通知家人赶紧送孩子去急诊。路上孩子开始意识模糊。阿莫斯后来才知道,当时雅各比的血糖水平高达735毫克/分升,而健康范围是70-140毫克/分升。幸运的是,雅各比病情没有发展到糖尿病酮酸血症,简称DKA。DKA是一种可能致命的并发症,由于血糖水平持续升高,血液变酸性,导致器官衰竭。 阿莫斯一家住在丹佛郊区,可怕事件发生后医生给出的应对方案却极为随意,也不明确。她的家人上了速成课程学习1型糖尿病,了解高血糖和低血糖如何危及生命。他们得知要计算雅各比的糖分摄入,一天里要多次检查血糖,刺破手指用糖尿病测试条,然后手写记录在日志中。 压力,训练,胰岛素,各种食物……再加上各项的影响因素相互作用,导致血糖水平出了名的难掌控,要把雅各比的血糖水平保持在健康范围内让人精疲力尽也很可怕。阿莫斯处处小心计算,但效果不佳,雅各比的血糖就像坐过山车,时而飙升到极高水平(他会感觉疲劳),时而又会降到极低(他会头晕)。 但雅各比的生死就看这些数字。阿莫斯希望能时刻了解血糖水平,雅各比诊断后几个星期,她用尽一切努力测试儿子的血糖,一天能测20多次,远远超过保险覆盖的次数。 |
The quest to retrieve, analyze, and leverage that data has become the new gold rush. And a vanguard of tech titans—not to mention a bevy of hot startups—are on the hunt for it. Alphabet life sciences arm Verily is aiming to create a “baseline” of human health by tracking all kinds of biometric information from 10,000 volunteers (and is rumored to have an interest in the health insurance business). Apple just released an iPhone feature offering users in several big health systems instant access to their own medical record—an effort that joins its ongoing heart study with Stanford, testing if wearables can detect serious cardiac conditions. Tapping this reservoir, say many, will ultimately improve patient health and decrease medical costs, which are projected to rise 5.3% in 2018 alone, according to the Centers for Medicare & Medicaid Services. That’s a noble aspiration, certainly. But not lost on anyone is that it’s sure to make for a potentially blockbuster business too. David Friend, managing director at BDO, points out that data-rich Facebook and Google make their money on advertising—a business worth $200 billion, he estimates. “Health care is 15 times bigger than that,” he says. “We spend $3 trillion. In theory, if this is done right, you’ll have 15 Facebooks and 15 Googles. That’s what’s up for grabs.” Which is why so many old-guard health care companies, from hospitals and insurers to benefits managers and drug and device makers—which together account for one-fifth of the economy—are hastily recombining and reinventing themselves. The realignment promises not only to drastically reshape the health care landscape for companies overall, but for you as well. Optimists, like Accenture’s chief technology and innovation officer Paul Daugherty, predict that the “information asymmetry” will soon favor patients whose ownership of their own biological data will give them new power. To see what the new balance of power will look like in the coming years—and what it looks like right now—Fortune interviewed more than three dozen executives at companies across the health care continuum, along with entrepreneurs, doctors, patients, and other experts. Here’s how the big-data revolution is—and isn’t—transforming medicine. The Data Pill: A New Paradigm for Patients Right around his eighth birthday, Lindsay Amos noticed her son Jacoby seemed not quite himself. The usually active boy who played hockey and lacrosse was sluggish, and he seemed to be going to the bathroom a lot. When the boy’s doctor took a reading of Jacoby’s blood sugar, the family was told to rush to the ER. On the drive there, the eight-year-old drifted in and out of consciousness. Jacoby’s blood sugar, Amos later learned, was 735 mg/dL, compared with a healthy range of 70–140 mg/dL. The boy was lucky not to have developed diabetic ketoacidosis, or DKA, a sometimes fatal complication in which, owing to prolonged elevated glucose levels, the blood becomes acidic, and organs begin to shut down. The resolution to those terrifying events struck Amos, who lives in the Denver suburbs, as startlingly casual and vague. Her family received a crash course on Type 1 diabetes, and the life-threatening consequences of both dangerously high and low blood sugar. They were told to count Jacoby’s carbohydrates and check his blood sugar throughout the day—a process that involves pricking a finger and using a diabetes test strip—and to record this all manually in a logbook. The dance between stress, exercise, insulin, various kinds of food—and what impact all those factors and more have on blood sugar—is notoriously hard to master, and trying to keep Jacoby in a healthy range was both exhausting and frightening. She’d dutifully do the math, but it didn’t seem to matter. Jacoby was on a roller coaster: His glucose level swinging unpredictably to alarming highs (when he’d feel fatigued) to dangerous lows (when he’d feel dizzy). His life depended on this number. Amos wanted to have an idea of where it stood at all times and for the first few weeks after Jacoby’s diagnosis, she did her best—testing her son’s glucose levels 20 or so times a day, far exceeding the number of test strips their insurance provider covered. |
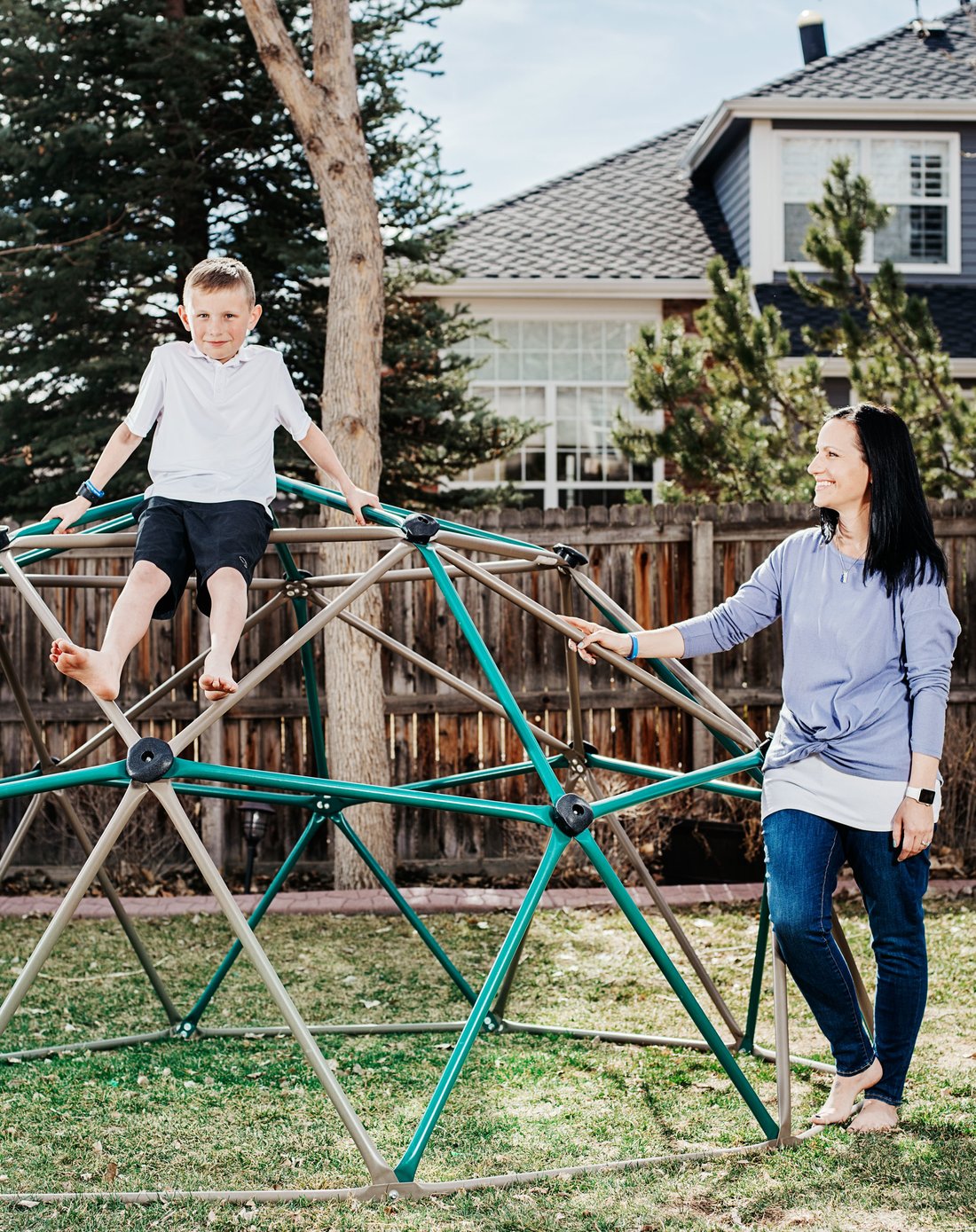
每年都有数百万美国家庭遇到类似疾病冲击;但2015年开始,新出现的智能手机技术部分缓解了担忧。加州一家名叫Dexcom的公司将血糖持续监测仪(已经问世十多年)与智能手机(或智能手表)无线连接,用户可以监测,绘制并分享血糖数据,每隔五分钟即可获得最新数据,全天待命,血糖达到危险水平时还能发出警报。 一些专家认为,随时在线的设备会告诉病人太多信息,但阿莫斯半开玩笑地介绍自己如何“跟踪”儿子血糖水平时说,这种仪器堪称救生员。她说,雅各比现在可以正常地读小学三年级,只要注意看看Apple Watch上的血糖数据就可以,不用再一天跑很多趟校医院。(阿摩斯也在iPhone上随时盯着。)而且现在雅各比可以安心睡整晚,不用隔几个小时就起床扎手指测血糖。 设备提供的还不仅仅是安心,其收集的数据确实可以帮阿莫斯和雅各比了解糖尿病情况以及如何控制。他们能发现哪些食物导致血糖飙升,以及何时注射胰岛素能更好地控制比萨之类复杂碳水化合物。是的,他的糖尿病没有治愈。但现在至少可以预测,很少出现意外。很明显,这项技术已经完全融入他们的日常生活。“跟汽车安全带和自行车头盔没有区别。”阿莫斯表示。 这只是智能手机和联网设备改变病人与健康数据关系的方式之一,过程中也能帮助病人改善健康状况。 Virta和Omada Health之类数字糖尿病预防和治疗平台还连接起社区和健康教练,教练可以远程监控体重、血糖、饮食和服药等情况。现在还有Proteus Digital Health可食入传感器,都值得《黑镜》拍一集了,这种传感器可以帮助患者(以及医生和家属)随时查看是否服药。Froedtert医院及威斯康星医疗网络医学院首席创新和数字官迈克尔·安德烈斯介绍,每粒药片接触胃酸时都会启动应用程序,传感器把整个过程显示得像游戏一样。根据Froedtert测试,丙肝患者服用Gilead Harvoni等昂贵药物期间,配合传感器按时服药比例达98.6%。按时服药不仅有利于治愈疾病,还能省下不少钱,因为多吃一个月药费用会多出数万美元。这就是Froedtert(而不是患者)支付传感器费用的原因。 |
This everyday trauma affected millions of American families each year; then, in 2015, a bit of smartphone technology took away some of the worry. A California company called Dexcom connected a continuous glucose monitor (a device that had been around for more than a decade) wirelessly to a smartphone (or smart watch), allowing the user to read, plot, and share blood sugar levels with anyone, at five-minute intervals, all day long—and sending an alert when patients were at risk. While some experts believe such always-on devices can leave patients with too much information, Amos—who half-jokingly speaks of “stalking” her son’s glucose levels—says it’s a lifesaver. Jacoby is now just another normal third-grader, she says. Rather than leaving class to go to the nurse’s office multiple times a day, he discreetly monitors his blood sugar on his Apple Watch. (Amos watches too, on her iPhone.) And instead of waking every few hours to have his finger pricked, he sleeps through the night. But the device offers more than just peace of mind. The data it generates has actually helped Amos and Jacoby understand his diabetes and how to manage it. It lets them see what foods make his blood sugar soar and how best to time his insulin shots around complex carbs like pizza. No, his diabetes isn’t cured. But his blood sugar is now predictable and rarely triggers an alarm. It’s remarkable how unremarkable the technology now is in their lives. “It’s no different than a seat belt or a bicycle helmet,” she says. This is but one way in which smartphones and connected devices are changing the relationship between patients and their health data—and enabling them to improve their health in the process. Digital diabetes prevention and treatment platforms such as Virta and Omada Health connect users with support communities and health coaches—who can remotely monitor things like weight, blood sugar, diet, and medicine intake. Then there’s Proteus Digital Health’s ingestible sensor, which—with a technology worthy of an episode of Black Mirror—helps patients (and, if they want, their doctors and family members) keep tabs on whether or not they’re taking their meds. By pinging an app every time a pill hits stomach acid, the sensor gamifies the prescription process, as Michael Anderes, the chief innovation and digital officer at Froedtert and the Medical College of Wisconsin Health Network, puts it. Hepatitis C patients on expensive drugs like Gilead’s Harvoni were 98.6% compliant in taking the medicine at the right time when using the sensors, in Froedtert’s experience. That’s not just critical for curing the disease, it’s also a major money saver because an extra month of medication would cost tens of thousands of dollars. Which is why Froedtert (not its patients) foots the bill for the sensor. |
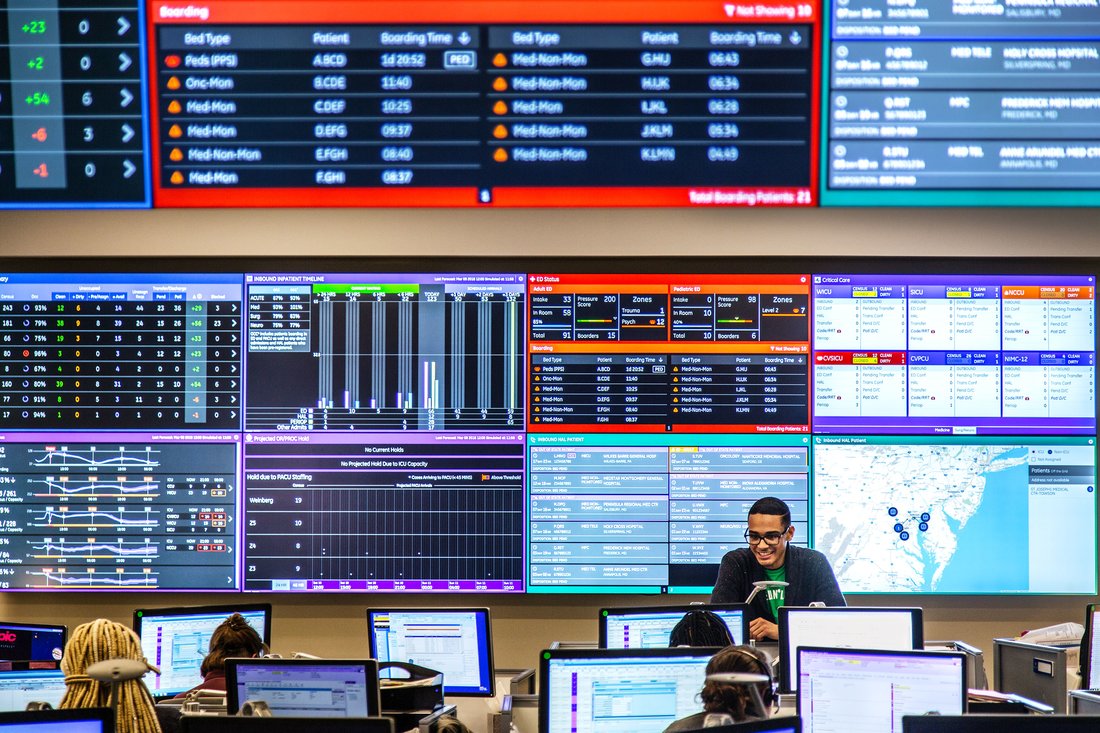
流行的Apple Watch或Android系统可穿戴健康设备已经普及,提醒各种健康事项,从睡眠呼吸暂停到高血压,甚至是严重心律失常。人们越发关注自己的基因组,希望预测患上某些疾病(如癌症和阿尔茨海默病)的风险,有些暂时还无法实现,有些技术尚不完备(并且有争议)。消费者开始利用Colour Genomics和23andMe等公司非常便宜又方便的基因检测试剂盒,检测结果会提示罹患某些疾病几率较高。支持者认为,了解风险有时可以帮人们采取相应预防措施,从而降低风险。 突然之间,类似家庭基因测试变成必需品:最近的黑色星期五,23andMe的标准DNA测试销量跻身亚马逊前五,差点赶上亚马逊自家出品的智能音箱Echo Dot和多功能高压锅“Instant Pot”。 所有革新技术的卖点都很简单:消费者主导。 一些大型保险公司甚至发现,让患者参与数据有助于改善结果和控制成本。这也是安泰保险首席执行官个马克·贝尔托里尼下的赌注。贝尔托里尼正争取与CVS达成合作,他认为,如果企业能明确告知消费者享受的益处,消费者就可能甚至会积极共享数据。“我们制定了各种规则保护数据,”贝尔托里尼表示,“但如果换个角度告诉客户,‘如果我们能掌握有关你的信息,服务起来更方便,’他或她就会愿意提供数据。这也是社交媒体作用很大的原因。” 掌握个性化数据后,公司可以与患者共同制定健康计划,贝尔托里尼说:“我们会告诉患者,如果一起制定计划就可以免除共同支付金额,也不必再授权,就因为计划是一起制定的。” 指挥中心:通过数据实现更好决策 疼痛来袭时,罗德尼·M正在给妻子挑选生日礼物。这位52岁的公关公司首席执行官抓紧胸口,挣扎着做在椅子上,腰部以上都失去了知觉,只感到麻木。他感觉就像“被卡车撞了一样”。 两年前罗德尼刚治疗过癌症,又患上一种新重病——为身体输送血液的关键动脉撕裂导致的“主动脉夹层”。 他还算幸运,急救车及时赶到把他送到马里兰州哥伦比亚市的霍华德县总医院,五分之一罹患动脉夹层的病人送到医院之前就去世了。这还没折腾完,几分钟内罗德尼又被飞机送到巴尔的摩的约翰霍普金斯医院,经过七个小时的复杂手术终于活了下来。 当时他并不知道,其实是霍普金斯医院人工智能驱动的数据“指挥中心”救了他的命。“41分钟。从监控系统报警到飞机从医院起飞只用了41分钟。”首席行政官吉姆·舒伦表示,他负责医疗中心不断扩大的急诊部门。 指挥中心从十几个数据流中实时提取信息,包括病历、紧急调度服务更新、实验检测结果,以及在特定时间医院有多少张病床。然后通过人工改进的算法,系统可以瞬间完成患者分级并根据需要进行安排,例如在罗德尼的案例中提前安排好外科手术团队。 对于医院来说,数据管理产生的经济效益无可争议。“霍普金斯医院指挥中心2016年启动以来,收治复杂癌症患者的能力增加了约60%,急诊室等床时间(等待住院病床)减少了25%以上,而手术室的等候时间减少了60%,“霍普金斯指挥中心设计方——通用电气医疗该项目负责人杰夫·特里说。霍普金斯医院的舒伦表示,应用该技术后,相当于医院不用实际投入,而收治能力增加15到16张病床。今年通用电气计划宣布新成立10个指挥中心,覆盖30家不同的医院。特里称,未来五年里医疗中心的投资回报率可达近4比1。 电子病历(EHR)也是近期大数据最新突破,可能没那么吸睛,但同样具有革新性。 电子病历是医疗圈少有人人讨厌的技术。医生抱怨称浪费的时间太多,跟其他医疗记录系统也没法顺利协作,并且其中大部分患者都读不懂。2015年以来,至少有593篇学术论文和一条说唱视频吐槽过该技术导致医生耗尽精力。如果大数据真能革新行业,机会可能就在这,应该将电子病历从耗时工作转变为可行的研究工具。 密歇根州西南部一个非盈利社区卫生系统Lakeland Health里,大数据确实发挥了作用。2012年,Lakeland开始启用电子病历系统,但基本由纸和笔组成。护士先在图表里记录每个病人的生命体征,回到电脑边再手动重新输入医院的电子系统,这一转录过程需要15到20分钟,并且经常出现错误。2016年中,医院启动全新流程,通过患者的腕带自动上传,或护士利用手持设备的床边输入数据。 Lakeland首席护理信息官阿瑟·巴拉奇表示,从一开始就有个明显变化:护士们花在输入数据上的时间减少,有更多时间照顾患者。但更彻底也令人惊讶的变化是“蓝色警报”下降:即患者心脏和呼吸停止。自英荷健康巨头飞利浦开发的新系统于2016年6月推出后,蓝色警报已下降56%。为什么?部分原因是警报系统嵌入了人工智能技术,不仅能检测生命体征的细微变化,还能根据病情打出风险评分,方便护士优先照顾病情更危急的病人。 “重要的是诚实地认识现状。”飞利浦战略与创新首席医疗官罗伊·史麦斯表示。许多新数字和数据工具关注的重点不是护理,而是如何使护理更高效、更智能、更精确地实现。 史麦斯跟《财富》杂志采访的许多位专家观点一致,即使是对数字健康极感兴趣的人。他们都警告不要过度使用新医疗技术。 “我们过度承诺,真正实现的不多。”西达斯西奈医学中心医生兼健康服务研究主任布伦南·斯皮格尔表示。“我认为自己是对技术保持怀疑的技术狂。但硅谷这个“回声室”里有太多人从来没接触过病人,也不理解数字医疗多么困难。“斯皮格尔还在加州洛杉矶分校担任医学和公共健康教授,他举了亲身经历的失败案例为证,包括2015年西达斯西奈想通过Fitbit、Apple Watch、Withings等可穿戴设备将病人与电子病历连接起来,结果一败涂地。“我们没能给病人适合的信息,也没诚挚邀请他们参与项目。”潜在参与者没什么理由参与连接和项目,因为项目没有明确的价值主张。“数字健康不是计算机科学或工程科学,而是社会科学和行为科学。” 斯科利普斯研究所的埃里克·托普尔同时也是一位著名的心脏病专家,他也提出了类似警告。“承诺很多,但大部分没实现。”他表示原因是存在各种系统性障碍。挑战之一便是美国僵化又“长期存在”的医疗机构。美国医生有半数超过50岁,他们抗拒改变,“除非获得更多补偿。” 对抗“故障问题” 按照安进公司的说法,大数据已然颠覆加州生物医药研发过程,还大大影响了研发阶段新药。事情是2011年开始的,时任研发主管的肖恩·哈珀前往冰岛旅行。他的目的是解决公司面临的“故障问题”,也是行业的问题。简单来说,90%的研发新药都没法进入市场。 研发新药非常昂贵而且低效。企业经常投资数十亿美元,花很多年努力验证可能有希望的科学假设。药品科学家渴望化学试验时突降好运,实际上并没完全理解努力追求的生物复杂性,也不明白为何某些药在白鼠身上作用明显,对人类却不起作用。 对哈珀来说,冰岛似乎可以提供一些独特的医疗数据。数据由冰岛政府提供,包括16万公民的基因排序信息,还有医疗和家谱,数据由总部位于雷克雅未克的人类基因设备公司deCode存储分析。该公司1996年成立,一直在苦苦经营。 尽管偿付能力存在问题,但deCode在基因探索领域成果丰硕。其拥有的大量数据可以用来挖掘遗传变异人群,并将变异与癌症到精神分裂症等疾病的临床结果联系起来。随着计算机处理能力提升,测序成本大幅下降,哈珀发现该公司对药物研发来说是价值低估的资产,于是2012年安进公司以4.15亿美元将其收入囊中。 此次收购彻底改变了安进的研发过程。收购之前,安进候选分子中只有15%针对特定的遗传目标进行了验证。收购完成后,安进开始利用deCode的数据库评估所有候选药物。审核发现了一些明显不起作用的药物。证据显示,有5%的候选分子没有疗效。管理层立刻关停相关项目(包括一种非常受期待的冠状动脉药物,而且即将进入人体试验阶段),转而优先考虑基因靶点明确的药物。经deCode基因数据库确认后,安进也通过了十多种药物。 哈珀表示,现在安进公司四分之三的研发产品都会参考基因数据,大部分都来自deCode数据库,如今收购成本早已收回。 尽管对药物靶点进行基因验证并不能保证最后成功,但科学家们还是得弄清楚如何安全有效地给药,应对大量生物学上的挑战,确实开了个好头。哈珀表示,“如果回报率能提高50%,变化已经很大。” 生物科技公司再生元也曾想与deCode合作,跟安进公司收购deCode时间差不多,其策略也跟安进类似。最终再生元没有寻求收购,而是以再生元遗传学中心(RGC)的形式建立自己的研究中心,花费四年给大量外显组完成测序(蛋白质编码部分的基因组)尽可能与病历配对,并加速药物研发。 再生元基因组生物信息学负责人杰夫·里德表示,很多人在讨论药品研发时利用基因技术,“却没有提前设计样本流。”简单来说:他们没有数据。后来里德得知再生元与盖辛格公司有合作,便加入了再生元(参见《基础护理》),盖辛格是位于宾夕法尼亚州的健康管理系统,计划从10万名病历完整的患者中收集样本并对完成测序。“他们希望获得数据后能切实改善对患者的护理。”再生元遗传学中心负责人阿里斯·巴拉斯说。 如今再生元合作方已超过60个,包括已招募50万名参与者的英国生物银行。巴拉斯和里德说,其掌握的数据规模和多样性都不断发展,内部研究数据能力也不断增强,这点至关重要。迄今为止已启动50个靶点生物学项目。 隐藏的数字:病历未尽之用 肿瘤科医生和前杜克大学教授艾米·阿伯内西认为,杂乱的健康信息没什么意义,除非符合两个关键标准:质量和背景。“不了解医学实践核心的人都不知道到底多么混乱。”四年前担任Flatiron Health首席医疗官的阿伯内西表示,这家创业公司由谷歌风投(GV)投资。 以Flatiron专长的癌症病历为例。肿瘤学电子病历中的许多重要信息(实际上约一半)可能都在医生的笔记中,但笔记没法组成特定的数据字段。各种观察结果没法按类整理在表格中。 “过去电子病历只是收费和收藏工具,医生按规矩撰写才能保住工作。”田纳西肿瘤中心的医生兼首席执行官杰弗里·巴顿解释说。田纳西肿瘤中心是基于社区的医疗机构,收治了该州大部分癌症患者,也是数百个使用Flatiron系统的社区癌症中心之一。 讽刺的是,Flatiron的真正卖点是人类。遇到此类数据,人类往往能发现计算机系统可能错过的细节。阿伯内西说,真正的挑战不是收集数据,而是要“清理干净”。“如果不理解相关背景真的很难做到。” Flatiron现在掌握美国20%主动治疗的癌症患者数据,“数据结构很清晰。”罗氏制药公司首席执行官丹尼尔·奥蒂表示,今年2月罗氏出资19亿美元收购了Flatiron公司。“Flatiron特别之处在于能整理出符合监管要求的真实数据。”奥蒂告诉《财富》。他表示,Flatiron的数据非常完备,“理论上可以取代罗氏为癌症免疫治疗药物Tecentriq设置的临床试验‘对照组’之一”。 理论上,Flatiron的数据系统可能对临床试验招募产生更广泛的影响。数十年来,癌症药物研究中病人招募都是最大挑战之一。举例来说,如果招募顺利就更容易根据病人情况匹配适合的药物。 IBM沃森在该领域初见成效。梅奥诊所今年3月报告称,使用IBM先进的认知计算系统后,乳腺癌临床试验参与人数增加了80%。 “沃森帮我们更快更准确地匹配患者与潜在的临床试验,以往肿瘤医生很难做到。”梅奥诊所首席信息官克里斯托弗·罗斯接受贸易出版物MobiHealthNews采访时表示。 即使是以前很少有机会接触患者的机构,例如药房福利管理结构,由于掌握海量数据,也可能为改善人类健康和降低成本贡献力量。 以密苏里州的药房福利管理结构Express Scripts为例,今年 3月刚刚由信诺保险收购。Express Scripts每年为1亿美国人管理14亿个处方,人们什么时候不按时吃药都知道。不遵医嘱服药每年导致的成本达1000亿美元至3000亿美元,数字差异是由于测算方式不同。成本出现的原因是患者不遵医嘱出现并发症,引起后续治疗。 Express Scripts首席数据官汤姆·亨利表示,已经发现300种可能让病人放弃照处方配药的因素。各项因素包括从基本的人口统计数据(收入水平和邮政编码)到行为数据(根据患者不取处方药后的调查问卷,判断患者健忘程度和拖延倾向)再到直观性较低的因素,如开药者和患者的性别(接受女性医生诊治的男性不遵从医嘱可能性更大)。该公司称,算法准确率达到94%,可以用算法为患者风险评分,并采取不同的提醒服务。亨利称提醒服药的方式“比较温和,不会强迫”。总之,Express Scripts称不遵医嘱服药现象减少了37%,为客户节省了1.8亿美元。 与其他领域的革命一样,许多人没考虑清楚下一步,也没准备好承担相应后果便纷纷涌入。在医疗大数据社交实验中问题也有多方面,从病人隐私到警告人们有些风险无法避免时的道德困境等。对许多数字医疗支持者来说,大数据仿佛是苦苦等待的一粒“魔术子弹”。问题是这颗子弹究竟往哪里打。(财富中文) 本文另一版本发表于2018年4月《财富》杂志,标题为《当大数据遇上生物学》。 译者:Pessy 审校:夏林 |
Wearable health trackers like the popular Apple Watch or Android-based devices are now alerting their owners to everything from sleep apnea to hypertension to even serious cardiac arrhythmias. And increasingly, that self-awareness is drilling into our own genomes, helping people—if for now, imperfectly (and controversially)—gauge their risk of developing certain diseases, such as cancer and Alzheimer’s. Consumers are turning to ever-cheaper, spit-and-send genetic test kits offered by companies like Color Genomics and 23andMe, to forewarn them of specific genetic susceptibilities—an awareness that, boosters say, can sometimes enable individuals to take preventive action that may mitigate those risks. Out of the blue, such at-home gene tests have become consumer must-haves: On the most recent Black Friday, 23andMe’s standard DNA test was one of Amazon’s top five sellers—barely trailing Amazon’s own Echo Dot and the “Instant Pot.” The selling point for all of these transformative technologies is a simple one: The consumer is in the driver’s seat. Some big insurers are even discovering that engaging patients with their data is a good way to improve outcomes and control costs. That’s what Mark Bertolini is betting on. Bertolini, the CEO of Aetna, which is looking to combine forces with CVS , believes that consumers can be—and would actively want to be—data-sharing partners, if companies can demonstrate how consumers can benefit from that cooperation. “We have all these rules about protecting data,” says Bertolini. “But if you turn it around and say to the customer, ‘If we have this information about you, we can make this a lot more convenient for you,’ he or she will give you the data. That’s why social media works the way it does.” With that personalized data, then, the company can build a health plan in concert with those patients, says Bertolini: “We want to say to them, if we build a plan together, there are no copays and there are no authorizations because we built it together.” Command Central: Better Decisions Through Data Rodney M. was picking up a birthday present for his wife when the pain hit. The 52-year-old CEO of a communications firm clutched his chest and scrambled to a seat. He felt numb, paralyzed from the waist down. It felt like he’d been “hit by a truck.” Already a cancer survivor in remission for two years, Rodney had just suffered a whole new kind of medical nightmare—an “aortic dissection,” caused by a tear in the critical artery that supplies the body’s lifeblood. He was lucky when the ambulance arrived quickly to take him to Howard County General Hospital, in Columbia, Md.—one in five aortic dissection patients dies before reaching a hospital. But his journey didn’t end there: Within minutes, Rodney was being airlifted to Johns Hopkins in Baltimore—and after a complex, seven-hour surgery, survived. He didn’t know it at the time, but an artificial intelligence-powered data “command center” at Hopkins helped save his life. “Forty-one minutes. That’s how long it took to get wheels up from the hospital,” says Jim Scheulen, the chief administrative officer tasked with overseeing the medical center’s sprawling emergency medicine unit. The command center pulls in information from more than a dozen data streams in real time, including patient health records, emergency dispatch service updates, lab results, and tabs on how many hospital beds are available at any given time. Then, through its human-trained algorithms, it makes split-second decisions on triaging patients and getting them where they need to go—prepping the surgical team ahead of time in cases like Rodney’s. For the hospital, the financial benefits of all this data management are unmistakable. “At Hopkins, there’s been about a 60% increase in the ability to accept complex cancer patients, an over 25% reduction in emergency room boarding [those waiting for an inpatient bed], and a 60% reduction in operating room holds with the command center since its launch in February 2016,” says Jeff Terry, who oversees such projects for GEHealthcare, which built the Hopkins command center. Hopkins’s Scheulen says the technology functionally expanded the hospital’s capacity by 15 or 16 beds without the need to add, well, actual beds. This year, GE plans to announce 10 new command centers covering 30 different hospitals—which, over five years, Terry claims, should yield those medical centers a roughly 4 to 1 return on their investment. Far less whizbang, but likely more transformative, are recent upgrades to last generation’s big-data breakthrough: the electronic health record (EHR). It’s hard to find a medical technology more universally hated than the EHR. Doctors complain that it consumes too much of their time, that they don’t work well with other medical records systems, and that they’re still largely indecipherable to patients. The technology’s role in physician burnout has been explored in no less than 593 scholarly articles and one rap video since 2015. But if the big-data mission has found a worthy calling, it is here—transforming electronic health records from a time suck to a viable research tool. That’s what happened at Lakeland Health, a not-for-profit community health system in southwest Michigan. Lakeland got an EHR system in 2012—but it might as well have been composed of paper and pen. Nurses there recorded vital signs of every patient on charts—and once back at their workstation, would manually reenter the stats into the hospital’s electronic system, a transcription process that ate up 15 to 20 minutes and often resulted in errors. Then, in mid-2016, they switched to a new process that enabled data to be automatically uploaded from patient wristbands or entered by nurses at their bedside on handheld devices. One change was obvious from the start: Nurses spent less time on data entry and more time tending to patients, says Arthur Bairagee, Lakeland’s chief nursing informatics officer. But more radical—and surprising—was the drop in “code blues”: the warnings that patients were in cardiac and respiratory arrest. They’ve dropped a mammoth 56% since the new system, developed by Anglo-Dutch health giant Philips, was introduced in June 2016. Why? In part, the A.I.-driven warning system built into the monitoring technology, which not only picks up on even subtle changes in vital signs, but also assigns patients risk scores that help nurses prioritize their attention. “It’s important to be honest and pragmatic about where we are now,” says Roy Smythe, chief medical officer for strategy and innovation at Philips. Many of the new digital and data tools aren’t so much about providing care, but rather about making that care more efficient, smarter, and precisely delivered. Like so many experts Fortune interviewed—even those who are gung ho about digital health—Smythe cautions against overhyping the new med tech. “We have overpromised and under–delivered,” says Brennan Spiegel, a physician and director of health services research at the Cedars-Sinai health system. “I consider myself a techno-skeptical techno-philiac. But there are way too many people in the Silicon Valley echo chamber who have never touched hands on a patient and don’t understand how hard digital health is.” Spiegel, who is also a professor of medicine and public health at UCLA, points to high-profile failures he’s personally experienced in the field—including a 2015 Cedars-Sinai project to connect patients, through wearables like Fitbit, Apple Watch, Withings, and others, to electronic health records, which flopped spectacularly. “We didn’t give patients the optimal messaging, and we didn’t invite them in the most compelling way.” So potential participants had little personal rationale to connect and stay engaged with the program because it didn’t present a tangible value proposition. “Digital health is not a computer science or an engineering science; it’s a social science and a behavioral science.” Eric Topol, at Scripps, who’s also a renowned cardiologist, sounds a similar cautionary note. “There’s a tremendous amount of promise, but so much is unfulfilled,” he says, owing to a variety of systemic roadblocks. Among the challenges, he says, are America’s rigid and “long in the tooth” medical establishment—half of U.S. doctors are over 50—which is resistant to changing its ways “unless it’s going to lead to higher compensation.” Fighting the “Failure Problem” To hear the folks at Amgen tell it, big data has upended the California biotech’s drug development process and significantly reshaped its pipeline. That story begins in 2011, when R&D chief Sean Harper, started making trips to Iceland. He was trying to solve his company’s—which is also the industry’s—“failure problem,” which is summed up by the fact that 90% of drug candidates fail to make it to market. Discovering new medicines is a wildly expensive and inefficient endeavor. Companies will often invest billions of dollars and many years chasing a promising scientific hypothesis. Pharmaceutical scientists hope for a moment of chemical serendipity, despite often not fully understanding the complexity of the biological mechanisms they’re targeting—or why something might fail in humans when it works so neatly in a mouse model. For Harper, Iceland seemed to offer an unparalleled pool of health-related data. The collection of that data—the genetic sequences of 160,000 citizens, along with their medical and genealogical records—was made possible by the Icelandic government, and the storage and analysis of that data was overseen by deCode, a Reykjavík-based human genetics outfit that, since its founding in 1996, had struggled to stay afloat financially. Despite its solvency issues, deCode had become a prolific publisher of genetic discovery. Its trove of data allowed the company to mine the population for genetic variants and connect those variants to clinical outcomes in diseases ranging from cancer to schizophrenia. As the cost of sequencing plummeted in sync with the rise of computer processing power, Harper saw an undervalued asset for drug discovery: Amgen bought the company in 2012 for $415 million. That purchase has utterly transformed Amgen’s R&D process. Prior to the deCode acquisition, only 15% of Amgen candidate molecules had been validated against specific genetic targets. After the purchase, Amgen began evaluating all of its drug candidates against deCode’s database. The review exposed some clear losers; in the case of 5% of its candidate molecules, there was evidence the agent wouldn’t work. Managers killed those programs (including one highly anticipated drug aimed at coronary disorders that was about to head into human trials) and prioritized others where there was a clear genetic target for the drug. Amgen also green-lighted more than a dozen drugs for which it found confirmation in deCode’s genetic data. Today, three-quarters of Amgen’s pipeline is based on genetic insights largely gleaned from the database, says Harper, and the company has more than earned its investment back. While having genetic validation for a target is no guarantee of success—scientists still have to figure out how to drug the target safely and effectively, and meet myriad other biological challenges—it does offer a head start. Says Harper, “If you can increase your rate of return by 50%, that’s enormous.” Regeneron, a biotech that was looking to partner with deCode around the same time Amgen bought it, has a similar strategy. But rather than buying up a genetic research outfit, it decided to build its own in the form of the Regeneron Genetics Center (RGC), an ambitious four-year old effort to sequence as many exomes (the protein-encoding part of the genome) as possible, pair them with medical records, and accelerate drug development. Lots of people talk about the promise of using genetics in pharma R&D, says Jeff Reid, Regeneron’s chief of genome bioinformatics, “but they don’t have a vision for sample flow.” Translation: They don’t have the data. Reid joined Regeneron when he learned the company had partnered with Geisinger (see “Keystone Care”), a Pennsylvania-based health care system with which it planned to collect and sequence samples from 100,000 consenting patients who also have comprehensive medical records. “They wanted to take this data and actually improve the care of patients,” says Aris Baras, who heads up the RGC. The company has since partnered with more than 60 other sources including the UK Biobank, which has recruited 500,000 participants. Baras and Reid say the scale and diversity of its growing data set, along with the ability to make all of these discoveries in-house, are critical. The work has so far spawned 50 target biology programs. Hidden Figures: The Untapped Value of Medical Records A random assortment of health information doesn’t mean much if it doesn’t meet at least two critical criteria, says oncologist and former Duke professor Amy Abernethy: quality and context. “Anyone who doesn’t understand the core aspects of practicing medicine can’t understand how messy it is,” says Abernethy, who four years ago became the chief medical officer at Flatiron Health, a startup backed by Google Ventures (GV). Take cancer records—Flatiron’s specialty—as an example. Many of the most useful nuggets in an oncology EHR (about half, in fact) may reside in doctors’ notes that aren’t structured into specific data fields. These are the sorts of observations that can’t be neatly packaged into categories on a form. “Historically, these electronic records are billing and collection tools, documentation we have to comply with to get paid,” explains Jeffrey Patton, a physician and CEO of Tennessee Oncology, a community-based health system that treats the largest number of cancer patients in the state and is one of hundreds of community cancer centers that now uses Flatiron’s system. Flatiron’s selling point, ironically, is humans. When it comes to this type of data, it seems, people can figure out things a purely computer-driven system might miss. The real challenge isn’t to gather the data, but to “clean it up,” says Abernethy. “And that’s really hard without an understanding of context.” Flatiron now has data from 20% of active cancer patients in the U.S., “and it’s extremely well structured,” says Daniel O’Day, CEO of Roche Pharmaceuticals, a unit of Roche Holding AG, which snapped up Flatiron in a $1.9 billion deal announced in February. “What set Flatiron apart was that it was able to create regulatory grade, real-world data,” O’Day tells Fortune—data that O’Day claims is so well curated, that it “could have theoretically replaced the ‘control’ arm” of one of Roche’s own clinical trials for the cancer immunotherapy drug Tecentriq. In theory, Flatiron’s system could have a broader impact on clinical trials accrual—for generations, one of the most stubborn challenges in cancer drug research—making it easier, for example, to match up specific patients with appropriate drug studies. That, indeed, is an area where IBM Watson has already found some success. In March, the Mayo Clinic reported that using Big Blue’s advanced cognitive computing system increased enrollment in clinical trials for breast cancer by 80%. “Watson is able to give us faster, better matching of patients to potential clinical trials that our oncologists wouldn’t have otherwise been able to see,” Mayo Clinic CIO Christopher Ross told the trade publication MobiHealthNews. Even organizations which previously only had distant relationships with patients—pharmacy benefit managers, for instance—are, because of the vast kingdoms of data they oversee, well positioned to draw insights that might improve population health and lower costs. Consider Express Scripts, the Missouri-based PBM that just announced its sale to insurer Cigna in March. Express Scripts administers 1.4 billion prescriptions for 100 million Americans each year—and it knows when you don’t take your meds. Such nonadherence costs between $100 billion and $300 billion a year, depending on which estimate one believes. That cost comes when patients suffer complications from not following the doctor’s orders. The company has identified 300 different factors that can help determine the likelihood that a patient will not fill a prescription says Express Scripts’ chief data officer Tom Henry. They range from that basic demographic data (income level and zip code) to behavioral data (one’s level of forgetfulness and tendency to procrastinate, gleaned from surveys the company does after patients fail to pick up their prescriptions) to less intuitive things like the genders of the prescriber and patient (men with a woman physician are more likely to not follow orders). The company uses the algorithm, which it says is validated and 94% accurate, to assign risk scores to patients and target them with varying modes of outreach—Henry says those efforts are “soft touch, nothing Orwellian.” Nonetheless, Express Scripts claims this work has reduced nonadherence by 37% and saved its clients more than $180 million. As with any revolution, many rush into action without considering weighty questions about what comes next and what unintended consequences may arise. In this social experiment, those questions cover everything from patient privacy to the ethical dilemma of warning someone about a risk they can’t avoid. To many digital health evangelists, big data is the “magic bullet” we’ve been waiting for. The question is, where exactly that bullet strikes. A version of this article appears in the April 2018 issue of Fortune with the headline “Big Data Meets Biology.” |