AI技术被大公司垄断,这家公司能否打破旧有格局?
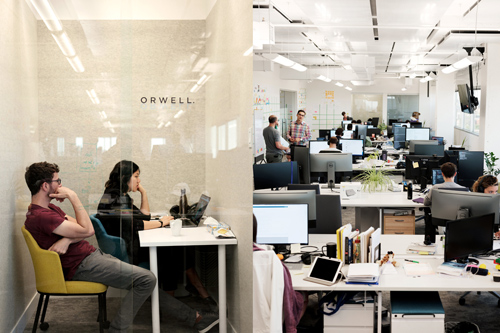
Element AI蒙特利尔办公室的Orwell静思室(左1);员工们在总部努力工作。Guillaume Simoneau for Fortune
在当今的人工智能领域,所有技术似乎都与加拿大不同大学的三位研究员有关。第一位是杰夫·欣顿,这位70岁高龄的英国人在多伦多大学任教,是深度学习这个子领域的先驱,而这一领域业已成为人工智能的代名词。第二位是一位名为杨立昆的法国人,57岁,上个世纪80年代曾在欣顿的实验室工作,如今在纽约大学执教。第三位是54岁的约书亚·本吉奥,出生于巴黎,成长于蒙特利尔,如今在蒙特利尔大学执教。这三位人士是十分要好的朋友和合作伙伴,正因为如此,业界人士将他们三位称之为加拿大黑手党。 然而在2013年,谷歌将欣顿收入麾下,Facebook聘请了杨立昆。这两位人士依然保留着其学术职务,并继续任教,但本吉奥并不是一位天生的实业家。他的举止十分谦虚,近乎谦卑,略微勾着腰,每天花大量的时间坐在电脑显示器旁。尽管他曾为多家公司提供过咨询服务,而且会经常性地获邀加入某一公司,但约书亚坚持追求他所热衷的项目,而不是那些最有可能获利的项目。他的朋友、人工智能初创企业Imagia联合创始人亚历山大·鲍斯利尔对我说:“人们应意识到,他的志向异常远大,其价值观也是高高在上。一些科技行业的工作者已经忘却了人性,但约书亚并没有。他真的希望这一科学突破能够对社会有所助益。” 科罗拉多大学波尔得分校的人工智能教授迈克·莫泽说的更为直率:“约书亚并没有背叛自己。” 然而,不背叛自己已经成为了一种少数派行为。大型科技公司,例如亚马逊、Facebook、谷歌和微软等,都在大肆收购创新初创企业,并挖走了大学的精英,为的是获取顶级人工智能人才。华盛顿大学人工智能教授佩德罗·多明戈斯称,他每年都会询问学术界的熟人,想了解是否有学生希望读取博士后学位。他对我说,上次他问本吉奥时,“本吉奥表示,‘他们还没毕业,我就已经留不住他们了。’”本吉奥受够了当前的这种态势,希望阻止大学的人才流失。他坚信,实现这一目标最好的方式就是以其人之道还治其人之身:资本主义重拳。 |
IN THE MODERN FIELD OF ARTIFICIAL INTELLIGENCE, all roads seem to lead to three researchers with ties to Canadian universities. The first, Geoffrey Hinton, a 70-year-old Brit who teaches at the University of Toronto, pioneered the subfield called deep learning that has become synonymous with A.I. The second, a 57-year-old Frenchman named Yann LeCun, worked in Hinton’s lab in the 1980s and now teaches at New York University. The third, 54-year-old Yoshua Bengio, was born in Paris, raised in Montreal, and now teaches at the University of Montreal. The three men are close friends and collaborators, so much so that people in the A.I. community call them the Canadian Mafia. In 2013, though, Google recruited Hinton, and Facebook hired LeCun. Both men kept their academic positions and continued teaching, but Bengio, who had built one of the world’s best A.I. programs at the University of Montreal, came to be seen as the last academic purist standing. Bengio is not a natural industrialist. He has a humble, almost apologetic, manner, with the slightly stooped bearing of a man who spends a great deal of time in front of computer screens. While he advised several companies and was forever being asked to join one, Bengio insisted on pursuing passion projects, not the ones likeliest to turn a profit. “You must realize how big his heart is and how well-placed his values are,” his friend Alexandre Le Bouthillier, a cofounder of an A.I. startup called Imagia, tells me. “Some people on the tech side forget about the human side. Yoshua does not. He really wants this scientific breakthrough to help society.” Michael Mozer, an A.I. professor at the University of Colorado at Boulder, is more blunt: “Yoshua hasn’t sold out.” Not selling out, however, had become a lonesome endeavor. Big tech companies—Amazon, Facebook, Google, and Microsoft, among others—were vacuuming up innovative startups and draining universities of their best minds in a bid to secure top A.I. talent. Pedro Domingos, an A.I. professor at the University of Washington, says he asks academic contacts each year if they know students seeking postdoc positions; he tells me the last time he asked Bengio, “he said, ‘I can’t even hold on to them before they graduate.’ ” Bengio, fed up by this state of affairs, wanted to stop the brain drain. He had become convinced that his best bet for accomplishing this was to use one of Big Tech’s own tools: the blunt force of capitalism. |
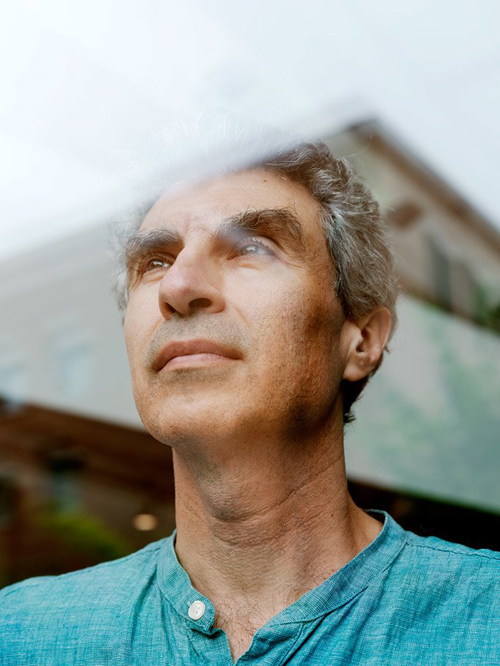
开发人工智能的一些大型科技公司已经实现了对资源的控制,然而约书亚·本吉奥却是行业内为数不多抵制商业化运作的人士。他的公司Element AI改变了这一情况。Guillaume Simoneau for Fortune
2015年9月的一个暖意融融的下午,本吉奥和四位最为要好的同事齐聚鲍斯利尔在蒙特利尔的家中。这次聚会实际上是一次策略讨论会,主题是一家技术转让公司(由本吉奥数年前与他人创建)。然而,对其专业领域的未来感到忧心忡忡的本吉奥也借此机会提出了一些自己一直在思考的问题:是否有可能创建一家企业,能够帮助初创企业和大学这个庞大的生态系统,而不是伤害这一系统,而且这家企业对于整个社会也能有所助益?如果可行的话,这家企业是否能够在大型科技企业主宰的世界中与其他企业竞争。 本吉奥尤其希望听到他的朋友让-弗朗西斯·加格内的意见,后者是一位充满活力的多产创业家,比本吉奥小15岁多。加格内此前曾向如今名为JDA Software的公司出售了由自己联合创建的初创企业。在该公司工作了三年之后,加格内离开了该公司,成为了加拿大风投公司Real Ventures的常驻创业者。如果加格内的下一个项目与自身的目标相一致,本吉奥非常希望参与其中。凑巧的是,加格内也在琢磨如何在这个由大科技公司主宰的世界中生存。在经过了三小时的会面之后,也就是在太阳开始落山之时,加格内对本吉奥和其他人说:“行了,我准备把这个商业计划再充实一下。” 那年冬天,加格内和同事尼古拉斯·查帕多斯到访了本吉奥在蒙特利尔大学不大的办公室。加格内在本吉奥学术行头——教科书、一沓沓的论文、写满了密密麻麻公式的白板——的环绕下宣布,在Real Ventures的支持下,他已经拿出了一个商业计划。他建议联合创建一家初创企业,后者将致力于为初创企业和其他缺乏资源的机构打造人工智能技术,这些机构没钱自行研发,但有可能对非大型科技公司之类的供应商感兴趣。这家初创企业的主要卖点就是,公司所拥有的人才可能是地球上最有才干的团队之一。它可以向来自于本吉奥的实验室以及其他大学的研究人员支付薪资,让他们每个月来公司工作几个小时,同时保留其学术职务。通过这种方式,公司便能以较低廉的价格获得顶级人才,而大学又可以保留其研究人员,同时主流客户便有机会与财大气粗的竞争对手开展竞争。各方面都是赢家,可能唯一吃亏的就是那些大型科技公司。 谷歌首席执行官桑达尔·皮查伊在今年早些时候宣布:“人工智能是人类正在攻克的最为重要的事情之一。它可能比电或火更加复杂。”谷歌和其他公司构成了本吉奥所担心的大型科技公司威胁,他们已将自己标榜为普及人工智能的生力军,其实现这一目标的方式便是让消费者和不同规模的公司都能用得起人工智能技术,而且用该技术来改善世界。谷歌云首席科学家李飞飞向我透露:“人工智能将让世界发生翻天覆地的变化。它是一种能够让工作、生活和社会更加美好的力量。” 在本吉奥和加格内讨论成立初创企业之时,这些大型科技公司还未卷入那些引人注目的人工智能伦理困境——关于出售人工智能并将其用于军事、可预测监控,以及在产品中不慎融入种族歧视和其他偏见,而且它们很快因此而尝到了苦果。但即便是在那个时候,知情人士都清楚地知道,大型科技公司正在部署人工智能来巩固其巨大的权力和财富。要理解这一现象,我们得知道人工智能与其他软件的区别。首先全球的人工智能专家相对较少,这意味着他们可以轻松拿到6位数的薪资,而这一点就会使组建大型工智能专家团队异常昂贵,只有那些最有钱的公司才负担得起。第二,相对于传统软件,人工智能对计算能力的要求更高,但也因此大幅提高了成本,同时还需要更多的优质数据,可是这些数据很难获得,除非你刚好是一家大型科技公司,能够无限制地获得上述两种资源。 本吉奥说:“如今,人工智能的发展方式出现了一种新的特征……专长、财富和权力集中在少数几家公司手中。”更好的资源能够吸引到更好的研究人员,后者会带来更好的创新,为公司创造更多收入,从而购买更多的资源。“有点类似于自给自足。”他补充道。 本吉奥最早与人工智能结缘时恰逢大型科技公司的崛起。本吉奥于上个世纪70年代在蒙特利尔长大,他尤其热爱科幻类书籍,例如菲利普·迪克的小说《Do Androids Dream of Electric Sheep?》。在这本书中,由大型公司制造的有感情机器人失去了控制。在大学,本吉奥读的是计算机工程专业,并在麦吉尔大学攻读硕士学位,当时他看到了由杰夫·欣顿撰写的一篇论文,与儿童时代那篇他异常喜爱的故事发生了共鸣,然后他就像着了魔一样。他随后回忆道:“我当时觉得,‘天哪,这就是我想要从事的事情。’” 随着时间的推移,本吉奥与欣顿和杨立昆成为了深度学习这一领域的重量级人物,后者涉及名为神经网络的计算机模型。但他们的研究出师不利,不仅找错了方向,而且目标也很模糊。深度学习在理论上十分诱人,但没有人能够让它在实践中有效运行。科罗拉多大学教授莫泽回忆道:“多年来,在机器学习会议上,神经网络并不怎么受欢迎,而约书亚会在会上大谈特谈其神经网络。而我的感受是,‘可怜的约书亚,真的是执迷不悟。’” 在00年代末期,研究人员明白了为什么深度学习未能发挥其效用。对神经网络进行高水平的培训需要更高的计算能力,但当时无法提供这样的计算能力。此外,神经网络需要优质的数字信息才能进行学习,在消费互联网崛起之前,没有足够的信息可供神经网络来学习。在00年代末,所有一切都发生了变化,而且大型科技公司很快便应用了本吉奥和其同事的技术,实现了诸多的商业里程碑:翻译语言、理解发言、面部识别等。 在那之前,本吉奥的弟弟萨米在谷歌工作,他也是一名人工智能研究员。有人力邀本吉奥与他的弟弟和同事一道,前往硅谷发展,然而,在2016年10月,他与加格内和查帕多斯携手Real Ventures创建了其自己的初创企业:Element AI。Element AI的投资公司DCVC的执行合伙人马特·欧克说:“除了Element AI之外,约书亚在任何人工智能平台都没有实质性的所有权,然而在过去五年中,有不少人都劝他这样做。他在公司完全靠声誉说话。” 为了赢得客户,Element利用了其研究人员的明星效应,其出资方的声誉光环以及提供比大型科技公司更个性化服务的承诺。但公司的高管也在从另一个角度发力:在当今这个年代,谷歌曾竞相将人工智能技术卖给军队,Facebook曾接待了一位影响了美国大选的激进演员,而亚马逊则在贪婪地吞食全球经济,但Element可以将自己确立为一家并不是很贪婪、更注重伦理的公司。 今春,我到访了Element位于蒙特利尔Plateau District的总部。公司的人数有了大幅的增长,达到了300人,而且根据墙上张贴的五颜六色的报事贴数量,工作量亦有了大幅增长。在一次会面中,公司的12位Elemental(Elemental人,公司员工称呼自己的方式)观看了一个正在开发中的产品的演示。其中,工作人员可以在类似于谷歌的界面上输入问题,例如“公司的招聘预测”,然后获得最新的答案。这些答案不仅基于现有的信息,而且还来源于人工智能根据业务目标的理解对未来做出的预测。我所见到的员工看起来既兴奋又疲惫不堪,这一现象在初创企业中十分常见。 Element所面临的一个持续挑战便是缺乏优质数据。培训人工智能模型最简单的方法就是在模型中录入带有详细标记的案例,例如数千张猫的图片或翻译后的文本。大型科技公司能够获得众多的消费导向型数据,这一优势在打造大规模消费产品时都是其他公司所无法比拟的。然而,企业、政府和其他机构拥有大量的隐私信息。即便公司使用了谷歌的邮件服务,或亚马逊的云计算服务,这些科技巨头也不会让这些供应商获取其有关设备故障或销售趋势或处理时间的内部数据库。而Element正是看到了这一点。如果公司能够获取多家公司的数据库,例如产品图片的数据库,那么公司便可以在客户允许的情况下,使用所有这些信息来打造一个更好的产品推荐引擎。大型科技公司也在向企业销售人工智能产品和服务,IBM正好也在专注于这项业务,但是没有人能够垄断这一市场。Element认为,如果它可以将自己融入这项机构,那么公司便可以建立企业数据方面的优势,类似于大型科技公司在消费品数据方面的优势。 公司在这一方面并非只是停留在纸面上。Element已与加拿大多家知名公司签订了协议,包括蒙特利尔港和加拿大广播电台,而且其客户有十几家都是全球1000强公司,但公司高管并没有透露具体数量,或任何非加拿大公司。产品也处于早期开发阶段。在问题回答产品的演示期间,该项目的经理弗朗西斯科·梅勒特(英语非母语)曾索取了有关“雇员在某个产品上耗费了多少时间”的信息。梅勒特承认这款产品的面世仍需很长的时间。但是他指出,Element希望它能够变得超级智能,甚至能够回答最有深度的策略问题。他给出了这样一个例子:“接下来应该怎么做?”这看起来似乎已经超越了策略的范畴,听起来有点近乎祈祷的意味。 谷歌雇员曾反对公司将人工智能技术提供给五角大楼的决定便是一个很好的例子。这一事实表明,科技公司在人工智能军事用途方面的立场已经成为了伦理的试金石。本吉奥和其他联合创始人在最初便发誓,绝不将人工智能用于攻击性军事用途。但是今年早些时候,韩国的一所科研大学——科学技术高级研究院宣布,该机构将与Element的主要投资方韩国国防部门韩华集团合作,打造军事系统。尽管这两家企业存在投资关系,但本吉奥签署了一封公开信,声讨韩国的这家机构,除非它承诺 “不开发缺乏有效人类控制的自主武器。”加格内则更为谨慎,他在给韩华集团的信件中强调,Element不会与制造自主武器的公司开展合作。不久后,加格内和科学家们得到了保证:科学技术高级研究院和韩华集团不会利用人工智能打造军事系统。 自主武器并非是人工智能所面临的唯一挑战,也不是其所面临的最严峻的挑战。研究人工智能社会影响的纽约大学教授凯特·克劳福德写道,人工智能领域所有“束手无策”的问题,例如从现有问题转而成为未来存在的威胁,以及“性别歧视、种族主义和其他形式的歧视”,已被写入机器学习算法。由于人工智能模型依靠工程师录入的数据来进行培训,数据中的任何偏见都会让获得这一数据的模型出现问题。 推特曾部署了由微软开发的人工智能聊天机器人Tay,以学习人类如何交谈,然而它很快便抛出了种族主义言论,例如“希特勒是正确的。”微软对此道歉,并将Tay下架,同时表示公司正在着手解决数据偏见的问题。谷歌的人工智能功能应用使用自拍来帮助用户寻找艺术作品中与自己长相相似的人物,非洲裔美籍人士的匹配对象基本上都是奴隶,而亚洲裔美籍人士的匹配对象基本上都是斜眼的日本歌舞姬,可能是因为数据过分依赖于西方艺术作品的缘故。我自己是印度裔美籍女性,当我使用这款应用时,谷歌给我发来的肖像是有着古铜色面孔、表情郁闷的印第安人首领。我对此也感到十分郁闷,谷歌这一点倒是没弄错。(一位发言人就此道歉,并表示“谷歌正在致力于减少人工智能中不公平的偏见”。) 像这类问题基本上源自于现实当中存在的偏见,然而雪上加霜的是,外界认为人工智能领域比广泛的计算机科学领域更加缺乏多元化,而后者是白人和亚洲人的天下。研究员提姆尼特·格布鲁是一名埃塞俄比亚裔美籍女性,曾在微软等公司供职,她说:“该领域的同质性催生了这些巨大的问题。这些人生活在自己的世界中,并认为自己异常开明而且先知先觉,但是他们没有意识到,他们让这个问题愈发严重。” 女性占Element员工总数的33%,占领导层的35%,占技术员工数量的23%,比很多大型科技公司的比例都要高。公司的雇员来自于超过25个国家:我遇到了一位来自塞内加尔的研究人员,他加入公司的部分原因在于,尽管他拿到了富布莱特奖学金来到美国深造,但无法获得留在美国的签证。但是公司并没有按照种族来划分其员工,而且在我到访期间,公司大部分员工似乎都是白人和亚洲人,尤其是管理层。运营副总裁安妮·马特尔是Element七名高管中唯一的女性,而工业解决方案高级副总裁奥马尔·达拉是唯一的有色人种。与Element相关联的24名学院研究员中,仅有3名是女性。在本吉奥实验室MILA网站上列出的100名学生当中,只有7名是女性。(本吉奥表示,该网站的信息并没有更新,而且他并不知道当前的性别比例。)虽然格布鲁与本吉奥的关系很好,但格布鲁在批评时也是毫不留情。她说:“我对他说,你都签署了反对自主武器的公开信,并希望保持独立,但公司打造人工智能技术的员工大部分都是白人或亚洲男性。你连自家实验室的问题都没解决,如何去解决世界性的问题。” 本吉奥表示,他对这一局面感到羞愧,并将努力解决这一问题,其中一个举措便是扩大招聘,并拨款帮助那些来自于被忽视群体的学生。与此同时,Element已经聘请了一位新人力副总裁安妮·梅泽,主要负责公司的多元化和包容性问题。为了解决产品中可能存在的伦理问题,Element将聘请伦理学家担任研究员,并与开发人员通力合作。公司还在伦敦办事处设立了AI for Good lab,由前谷歌DeepMind的研究员朱莉安·科尼碧斯执掌。在AI for Good lab中,研究人员将以无偿或有偿的方式,围绕能够带来社会福利的人工智能项目,与非营利性和政府等机构开展合作。 然而,伦理挑战依然存在。在早期研究中,Element使用其自有数据来制造某些产品;例如,问题回答工具的部分培训资料便来自于内部共享文件。运营高管马特尔对我说,因为令Element高管感到为难的是,如何在面部识别中使用人工智能技术才算是符合伦理。他们计划在自家雇员身上进行试验。公司将安装摄像头,这些摄像头在经过员工的允许之后捕捉其面部图像,并对人工智能技术进行培训。高管们将对员工进行调查,询问他们对该技术的感受,加深他们对于伦理维度的理解。马特尔说:“我们希望通过公司内部试验把这个问题弄明白。” 当然,这意味着至少在最初的时候,所有的面部识别模型都将基于并不能代表广泛人群的面部图片。马特尔表示,高管们意识到了这一问题:我们对于代表性不充分问题感到非常担忧,而且我们正在寻找对策。 即便是Element产品旨在为高管回答的问题——接下来应该怎么做?——亦充满了伦理挑战。对于能够实现利润最大化的举措,无论商用人工智能技术做出何种推荐,人们也很难对其责难。然而它如何做出这些决定?哪些社会代价是可以容忍的?由谁来决定?正如本吉奥所承认的那样,随着越来越多的机构部署人工智能技术,尽管会有新的岗位涌现出来,但数百万人有可能会因此而失去工作。虽然本吉奥和加格内最初计划向小型机构推销其服务,但他们随后还是将目光投向了排名前2000位的大公司;事实证明,Element对大量数据集的需求远非小型机构可以满足。尤为值得一提的是,他们将目光投向了金融和供应链公司,而其中规模最大的那些公司在这一方面并非就是毫无准备的门外汉。加格内表示,随着技术的改善,Element预计也将向小一点的机构销售其技术。但到了那个时候,Element向全球规模最大的公司提供人工智能优势的计划似乎更适合为现有的大公司锦上添花,而不是面向大众普及人工智能的福利。 本吉奥认为,科学家的工作是继续探索人工智能新成果。他说,各国政府应加大对这一领域的规范力度,同时更加公平地分配财富,并投资教育和社会安全网络,规避人工智能不可避免的负面影响。当然,这些主张的前提是,政府心怀民众的最大利益。与此同时,美国政府正在削减富人的税收,而中国政府,作为人工智能研究最大的资助者,将使用深度学习来监控其民众。华盛顿大学教授多明戈斯表示:“在我看来,约书亚认为人工智能可以符合伦理纲常,而且他的公司也能够成为一家合乎伦理纲常的人工智能技术公司。但是坦率地讲,约书亚有一点天真,众多的技术专家亦是如此。他们的看法过于理想化。” 本吉奥并不赞成这一结论。他说:“作为科学家,我认为我们有责任与社会和政府打交道,从而按照我们所坚信的理念来引导人们的思想和心灵。” 今春一个清冷明朗的早晨,Element的员工齐聚一堂,在一个已改造为活动场地的高屋顶教堂开展协同软件设计场外培训。参加的员工按照圆桌划分为不同的组别,其任务就是设计一款教授人工智能基础知识的游戏。我与其中的6名员工一桌,他们决定将这款人工智能游戏命名为Sophia the Robot(机器人索菲亚)。这个机器人发疯了,自然便需要使用人工智能技术来与之战斗并进行抓捕。新人力副总裁梅泽刚好就在这一桌。她表示:“我很喜欢索菲亚这个名称,因为我们需要更多的女性,但我不喜欢打打杀杀。”不少人在交头接耳时都表示同意这一观点。一位高管助理提出了建议:“可以将游戏的目标设定为改变索菲亚的思考方式,把它变为帮助世界。”新版本的游戏听起来令人更加愉悦,因为它与Element的自我形象结合的更加紧密。一位雇员对我说:“在办公室,公司不允许谈论Skynet(天网)。”后者是源自于《终结者》授权的对抗性人工智能系统。任何不小心谈论这一话题的员工都必须将1美元放到一个特别准备的罐子中。一位同事以非常高兴的口吻补充说:“我们应该持有积极乐观的态度。” 随后,我到访了本吉奥在蒙特利尔大学的实验室,由一个个点着日光灯的监狱式房间组成,房间里到处都是计算机显示器和一摞摞的教科书。在其中一个房间里,有十几个年轻人一边开发其人工智能模型,一边开着数学玩笑,同时还聊了聊自己的职业道路。我在无意中听到:“微软有着各种不俗的待遇,打折机票、酒店。”、“我每周去Element AI一次,然后拿到的是这台电脑。”、“他是一个叛徒。”、“你可以在其他领域说‘叛徒’这个词,但是在深度学习领域不行。”、“为什么?”、“因为在深度学习领域,每个人都是叛徒。”看起来,本吉奥无偿背叛的愿景还没有完全实现。 然而,本吉奥能够通过培养下一代研究人员来影响人工智能的未来,而且这种影响力可能是其他学者无法企及的。(他的一个儿子也成为了人工智能研究员;另一个儿子是一名音乐家。)一天下午,我到访了本吉奥的办公室,在这个面积不大、空旷的房间中,主要摆设是一个白板,上面潦草地写着“襁褓中的人工智能”,还有一个书架,上面摆放的书本包括《老鼠的大脑皮层》。本吉奥承认,尽管作为Element的联合创始人,但由于一直忙于人工智能最前沿的研究工作,自己并没有在公司办公室待过多长时间。这些研究离商业应用还有很长的路要走。 虽然科技公司一直专注于让人工智能能够更好地物尽其用,即发现规律并对其进行总结,但本吉奥希望越过这些最基本的用途,并开始打造深受人类智慧启发的机器。他并不愿意描述这类机器的详情。但人们可以想象的是,在未来,机器不仅仅是用来在仓库搬运产品,而是能够在现实世界中穿梭。它们不仅仅会对命令做出响应,同时还能理解并同情人类。它们不仅仅能够识别图像,还能够创作艺术。为了实现这一目标,本吉奥一直在研究人脑的工作原理。他的一位博士后学生对我说,大脑便是“智能系统可能会成为现实的证据”。本吉奥已将一款游戏作为其重点项目。在游戏中,玩家通过与这位伪装的婴儿讲话、指路等等,告诉一位虚拟儿童——也就是办公室白板上写的“襁褓中的人工智能”——世界如何运转。“我们可以从婴儿如何学习以及父母如何与自己的孩子互动中吸取灵感。”这看起来似乎很牵强,但是别忘了,本吉奥曾经看似荒诞的理念如今是大型科技公司最主流技术的理论支柱。 尽管本吉奥认为人工智能可能会达到与人类相仿的水平,但他对埃隆·马斯克这类人所宣传的影响深远的伦理问题不以为然,因为其前提就是人工智能的智慧水平要高出人类。本吉奥对于人类打造和使用人工智能时所做出的伦理选择更感兴趣。他曾经对一位采访者透露:“最大的危险在于,人们可能会以不负责任的方式或恶意的方式来对待人工智能技术,我是指用于谋取个人私利。”其他的科学家也同意本吉奥的看法。然而,随着人工智能研究的继续向前迈进,它依然由全球最强大的政府、企业和投资者提供资助。本吉奥的大学实验室的运转资金基本上就来自于大型科技公司。 在讨论最大科技公司的会议期间,本吉奥曾一度对我说:“我们希望Element AI能够发展成为这些科技巨头中的一员。”我问本吉奥,到那时,他是否会跟那些公司一样关注他所不齿的财富和权力。他回答说:“我们的理念不仅仅是为了创建一家公司,然后成为全球最有钱的公司。我们的目标是改变世界,改变商业的运行方式,让它不像现在这样集中在少数企业当中,并让它变得更加民主。”尽管我十分敬佩他的姿态,也对他的抱负有信心,但他的话与大型科技公司所奉行的口号没有多大的区别。不干坏事。让世界更加开放和互联。打造一个符合道德规范的企业并不在于创始人的抱负,而是关乎企业所有者在一段时间之后如何在社会公益和企业利润之间进行取舍。接下来应该怎么做?如果计算机仍然难以给出答案,那么多少令其感到安慰的是,人类在这个问题上也没有比计算机睿智多少。(财富中文网) 本文最初刊于2018年7月1日的《财富》杂志。 译者:冯丰 审校:夏林 |
On a warm September afternoon in 2015, Bengio and four of his closest colleagues met at Le Bouthillier’s Montreal home. The gathering was technically a strategy meeting for a technology-transfer company Bengio had cofounded years earlier. But Bengio, harboring serious anxieties about the future of his field, also saw an opportunity to raise some questions he had been dwelling on: Was it possible to create a business that would help a broader ecosystem of startups and universities, rather than hurt it—and maybe even be good for society at large? And if so, could that business compete in a Big Tech–dominated world? Bengio especially wanted to hear from his friend Jean-François Gagné, an energetic serial entrepreneur more than 15 years his junior. Gagné had earlier sold a startup he cofounded to a company now known as JDA Software; after three years working there, Gagné left and became an entrepreneur-inresidence at the Canadian venture capital firm Real Ventures. Bengio was keen on getting involved in Gagné’s next project, provided it aligned with his own goals. Gagné, as it happened, had also been wrestling with how to survive in a Big Tech–dominated world. At the end of the three-hour meeting, as the sun began to set, he told Bengio and the others, “Okay, I’m going to flesh out a business plan.” That winter, Gagné and a colleague, Nicolas Chapados, visited Bengio at his small University of Montreal office. Surrounded by Bengio’s professorial paraphernalia—textbooks, stacks of papers, a whiteboard covered in cat-scratch equations—Gagné announced that with Real Ventures’ blessing he had come up with a plan. He proposed cofounding a startup that would build A.I. technologies for startups and other under-resourced organizations that couldn’t afford to build their own and might be attracted to a non–Big Tech vendor. The startup’s key selling point would be one of the most talented workforces on earth: It would pay researchers from Bengio’s lab, among other top universities, to work for the company several hours a month yet keep their academic positions. That way, the business would get top talent at a bargain, the universities would keep their researchers, and Main Street customers would stand a chance of competing with their richer rivals. Everyone would win, except maybe Big Tech. GOOGLE CEO Sundar Pichai declared earlier this year, “A.I. is one of the most important things humanity is working on. It is more profound than, I dunno, electricity or fire.” Google and the other companies that together constitute the Big Tech threat that occupies Bengio have positioned themselves as forces to democratize A.I., by making it affordable for consumers and businesses of all sizes, and using it to better the world. “A.I. is going to make sweeping changes to the world,” Fei-Fei Li, the chief scientist for Google Cloud, tells me. “It should be a force that makes work, and life, and society, better.” When Bengio and Gagné began their discussions, the largest tech companies hadn’t yet been embroiled in the high-profile A.I. ethics messes—about controversial sales of A.I. for military and predictive policing, as well as the slipping of racial and other biases into products—that would soon consume them. But even then, it was clear to insiders that Big Tech companies were deploying A.I. to compound their considerable power and wealth. Understanding this required knowing that A.I. is different from other software. First of all, there are relatively few A.I. experts in the world, which means they can command salaries well into the six figures; that makes building a large team of A.I. experts too expensive for all but the wealthiest companies. Second, A.I. often requires more computing power than traditional software, which can be expensive, and good data, which can be difficult to get, unless you happen to be a tech giant with nearly limitless access to both. “There’s something about the way A.I. is done these days … that increases the concentration of expertise and wealth and power in the hands of just a few companies,” Bengio says. Better resources attract better researchers, which leads to better innovations, which brings in more revenue, which buys more resources. “It sort of feeds itself,” he adds. Bengio’s earliest encounters with A.I. anticipated the rise of Big Tech. Growing up in Montreal in the 1970s, he was especially taken with science fiction books like Philip K. Dick’s novel Do Androids Dream of Electric Sheep?—in which sentient robots created by a megacorporation have gone rogue. In college, Bengio majored in computer engineering; he was in graduate school at McGill University when he came across a paper by Geoff Hinton and was lightning-struck, finding echoes of the sci-fi stories he had loved so much as a child. “I was like, ‘Oh my God. This is the thing I want to do,’ ” he recalls later. In time, Bengio, along with Hinton and LeCun, would become an important figure in a field known as deep learning, involving computer models called neural networks. But their research was littered with false starts and confounded ambitions. Deep learning was alluring in theory, but no one could make it work well in practice. “For years, at the machine-learning conferences, neural networks were out of favor, and Yoshua would be there cranking away on his neural net,” recalls Mozer, the University of Colorado professor, “and I’d be like, ‘Poor Yoshua, he’s so out of it.’ ” In the late 2000s it dawned on researchers why deep learning hadn’t worked well. Training neural networks at a high level required more computing power than had been available. Further, neural networks need good digital information in order to learn, and before the rise of the consumer Internet there hadn’t been enough of it for them to learn from. By the late 2000s, all that had changed, and soon large tech companies were applying the techniques of Bengio and his colleagues to achieve commercial milestones: translating languages, understanding speech, recognizing faces. By that time, Bengio’s brother Samy, also an A.I. researcher, was working at Google. Bengio was tempted to follow his brother and colleagues to Silicon Valley, but instead, in October 2016, he, Gagné, Chapados, and Real Ventures launched their own startup: Element AI. “Yoshua had no material ownership in any A.I. platform, despite being hounded over the last five years to do so, other than Element AI,” says Matt Ocko, a managing partner at DCVC, which invested in the company. “He had voted with his reputation.” To win customers, Element relied on the star power of its researchers, the reputational glitz of its funding, and a promise of more personalized service than Big Tech could provide. But its executives also worked another angle: In an age in which Google was competing to sell A.I. to the military, Facebook had played host to rogue actors who influence elections, and Amazon was gobbling up the global economy, Element could position itself as a less predaceous, more ethical A.I. outfit. This spring, I visited Element’s headquarters in Montreal’s Plateau District. The headcount had expanded dramatically, to 300, and judging from the colorful Post-it notes columned on the walls, so had the workload. In one meeting, a dozen Elementals, as employees call themselves, watched a demo of a product in development, in which a worker could enter questions on a Google-like screen—“What’s our hiring forecast?”—and get up-to-date answers. The answers would be based not just on existing information but also on the A.I.’s predictions about the future based on its understanding of business goals. As is typical at fast-growing startups, the employees I met seemed simultaneously energized and utterly exhausted. A persistent challenge for Element is the dearth of good data. The simplest way to train A.I. models is to feed them lots of well-labeled examples—thousands of cat images, or translated texts. Big Tech has access to so much consumer-oriented data that it’s all but impossible for anyone else to compete at building large-scale consumer products. But businesses, governments, and other institutions own huge amounts of private information. Even if a corporation uses Google for email, or Amazon for cloud computing, it doesn’t typically let those vendors access its internal databases about equipment malfunctions, or sales trends, or processing times. That’s where Element sees an opening. If it can access several companies’ databases of, say, product images, it can then—with customers’ permission—use all of that information to build a better product-recommendation engine. Big Tech companies are also selling A.I. products and services to businesses—IBM is squarely focused on it—but no one has cornered the market. Element’s bet is that if it can embed itself in these organizations, it can secure a corporate data advantage similar to the one Big Tech has in consumer products. Not that it has gotten anywhere close to that point. Element has signed up some prominent Canadian firms, including the Port of Montreal and Radio Canada, and counts more than 10 of the world’s 1,000 biggest companies as customers, but executives wouldn’t quantify their customers or name any non-Canadian ones. Products, too, are still in early stages of development. During the demo of the question-answering product, the project manager, François Maillet, who is not a native English speaker, requested information about “how many time” employees had spent on a certain product. The A.I. was stumped, until Maillet revised the question to ask “how much time” had been spent. Maillet acknowledges the product has a long way to go. But he says Element wants it to become so intelligent that it can answer the deepest strategic questions. The example he offers—“What should we be doing?”—seemed to go beyond the strategic. It sounded quite nearly prayerful. LOOK NO FURTHER than Google’s employee revolt over its decision to provide A.I. to the Pentagon as evidence that tech companies’ stances on military use of A.I. have become an ethical litmus test. Bengio and his cofounders vowed early on to never build A.I. for offensive military purposes. But earlier this year, the Korea Advanced Institute of Science and Technology, a research university, announced it would partner with the defense unit of the South Korean conglomerate Hanwha, a major Element investor, to build military systems. Despite Element’s ties with Hanwha, Bengio signed an open letter boycotting the Korean institute until it promised not to “develop autonomous weapons lacking meaningful human control.” Gagné, more discreetly, wrote to Hanwha emphasizing that Element wouldn’t partner with companies building autonomous weapons. Soon Gagné and the scientists received assurances: The university and Hanwha wouldn’t be doing so. Autonomous weapons are neither the only ethical challenge facing A.I. nor the most serious one. Kate Crawford, a New York University professor who studies the societal implications of A.I., has written that all the “hand-wringing” over A.I. as a future existential threat distracts from existing problems, as “sexism, racism, and other forms of discrimination are being built into the machinelearning algorithms.” Since A.I. models are trained on the data that engineers feed it, any biases in the data will poison a given model. Tay, an A.I. chatbot deployed to Twitter by Microsoft to learn how humans talk, soon started spewing racist comments, like “Hitler was right.” Microsoft apologized, took Tay off-line, and said it is working to address data bias. Google’s A.I.-powered feature that uses selfies to help users find their doppelgängers in art matched African-Americans with stereotypical depictions of slaves and Asian-Americans with slant-eyed geishas, perhaps because of an overreliance on Western art. I am an Indian-American woman, and when I used the app, Google delivered me a portrait of a copperfaced, beleaguered-looking Native American chief. I also felt beleaguered, so Google got that part right. (A spokesman apologized and said Google is “committed to reducing unfair bias” in A.I.) Problems like these result from bias in the world at large, but it doesn’t help that the field of A.I. is believed to be even less diverse than the broader computer science community, which is dominated by white and Asian men. “The homogeneity of the field is driving all of these issues that are huge,” says Timnit Gebru, a researcher who has worked for Microsoft and others and is an Ethiopian-American woman. “They’re in this bubble, and they think they’re so liberal and enlightened, but they’re not able to see that they’re contributing to the problem.” Women make up 33% of Element’s workforce, 35% of its leadership, and 23% of technical roles—higher percentages than at many big tech companies. Its employees come from more than 25 countries: I met one researcher from Senegal who had joined in part because he couldn’t get a visa to stay in the U.S. after studying there on a Fulbright. But the company doesn’t break down its workforce by race, and during my visit, it appeared predominantly white and Asian, especially in the upper ranks. Anne Martel, the senior vice president of operations, is the only woman among Element’s seven top executives, and Omar Dhalla, the senior vice president of industry solutions, is the only person of color. Of the 24 academic fellows affiliated with Element, just three are female. Of 100 students listed on the website of Bengio’s lab, MILA, seven are women. (Bengio said the website is out of date and he doesn’t know the current gender breakdown.) Gebru is close with Bengio but does not exempt him from her criticisms. “I tell him that he’s signing letters against autonomous weapons and wants to stay independent, but he’s supplying the world with a mostly white or Asian group of males to create A.I.,” she said. “How can you think about world hunger without fixing your issue in your lab?” Bengio said he is “ashamed” about the situation and trying to address it, partly by widening recruitment and earmarking funding for students from underrepresented groups. Element, meanwhile, has hired a new vice president for people, Anne Mezei, who set diversity and inclusion as a top priority. To address possible ethical problems with its products, Element is hiring ethicists as fellows, to work alongside developers. It has also opened an AI for Good lab, in a London office directed by Julien Cornebise, a former researcher at Google DeepMind, where researchers are working, for free or at cost, with nonprofits, government organizations, and others on A.I. projects with social benefit. Still, ethical challenges persist. In early research, Element is basing some products on its own data; the question-answering tool, for example, is being trained partly on shared internal documents. Martel, the operations executive, tells me that because Element executives aren’t sure from an ethics standpoint how they might use A.I. for facial recognition, they plan to experiment with it on their own employees by installing video cameras that will, with employees’ permission, capture their faces to train the A.I. Executives will poll employees on their feelings about this, to refine their understanding of the ethical dimensions. “We want to figure it out through eating our own dog food,” Martel says. That means, of course, that any facial-recognition model will be based, at least at first, on faces that are not representative of the broader population. Martel says executives are aware of the issue: “We’re really concerned about not having the right level of representativeness, and we’re looking into solutions for that.” Even the question that Element’s product aims to answer for executives—What should we be doing?—is loaded with ethical quandaries. One could hardly fault a business-oriented A.I. for recommending whatever course of action maximizes profit. But how should it make those decisions? What social costs are tolerable? Who decides? As Bengio has acknowledged, as more organizations deploy A.I., millions of humans are likely to lose their jobs, though new ones will be created. Though Bengio and Gagné originally planned to pitch their services to small organizations, they have since pivoted to target the 2,000 largest companies in the world; Element’s need for large data sets turned out to be prohibitive for small organizations. In particular, they are targeting finance and supply-chain companies—the biggest of which aren’t exactly defenseless underdogs. Gagné says that as the technology improves, Element expects to sell it to smaller organizations as well. But until that happens, its plan to give an A.I. advantage to the world’s biggest companies would seem better-equipped to enrich powerful incumbent corporations than to spread A.I.’s benefits among the masses. Bengio believes the job of scientists is to keep pursuing A.I. discoveries. Governments should more aggressively regulate the field, he says, while distributing wealth more equally and investing in education and the social safety net, to mitigate A.I.’s inevitable negative effects. Of course, these positions assume governments have their citizens’ best interests in mind. Meanwhile, the U.S. government is cutting taxes for the rich, and the Chinese government, one of the world’s biggest funders of A.I. research, is using deep learning to monitor citizens. “I do think Yoshua believes that A.I. can be ethical, and that his can be the ethical A.I. company,” says Domingos, the University of Washington professor. “But to put it bluntly, Yoshua is a little naive. A lot of technologists are a little naive. They have this utopian view.” Bengio rejects the characterization. “As scientists, I believe that we have a responsibility to engage with both civil society and governments,” he says, “in order to influence minds and hearts in the direction we believe in.” ONE COLD, BRIGHT MORNING this spring, Element’s staff gathered for an off-site training in collaborative software design, in a high-ceilinged church that had been converted into an event space. The attendees, working in groups at round tables, had been assigned to invent a game to teach the fundamentals of A.I. I sat with some halfdozen employees, who had decided on a game about an A.I. named Sophia the Robot who had gone rogue and would need to be fought and captured, using, naturally, A.I. techniques. Mezei, the new VP for people, happened to be at this table. “I like the fact that it’s Sophia, because we need more women,” she interjected. “But I don’t like fighting.” There were murmurs of assent all around. An executive assistant suggested, “Maybe the goal is changing Sophia’s mindset so it’s about helping the world.” This was a more palatable version of the game, one better aligned with Element’s self-image. One employee told me, “At the office, we’re not allowed to talk about Skynet”—the antagonistic A.I. system from the Terminator franchise. Anyone who slips up has to put a dollar into a special jar. A colleague added, in a tone of great cheer, “We’re supposed to be positive and optimistic.” Later I visited Bengio’s lab at the University of Montreal, a warren of carceral, fluorescent-lit rooms filled with computer monitors and piled-up textbooks. In one room, some dozen young men were working on their A.I. models, exchanging math jokes, and contemplating their career paths. Overheard: “Microsoft has all these nice perks—you get cheaper airline tickets, cheap hotels.” “I go to Element AI once a week, and I get this computer.” “He’s a sellout.” “You can scream, ‘Sellout!’ in other fields, but not deep learning.” “Why not?” “Because in deep learning, everyone’s a sellout.” Bengio’s sellout-free vision, it seemed, had not quite been realized. Still, perhaps more than any other academic, Bengio has influence over A.I.’s future, by virtue of training the next generation of researchers. (One of his sons has become an A.I. researcher too; the other is a musician.) One afternoon I went to see Bengio in his office, a small, sparse room whose main features were a whiteboard across which someone had scrawled the phrase “Baby A.I.,” and a bookcase featuring such titles as The Cerebral Cortex of the Rat. Despite being an Element cofounder, Bengio acknowledged that he hadn’t been spending a lot of time at the offices; he had been preoccupied with frontiers in A.I. research that are far from commercial application. While tech companies have been focused on making A.I. better at what it does—recognizing patterns and drawing conclusions from them—Bengio wants to leapfrog those basics and start building machines that are more deeply inspired by human intelligence. He hesitated to describe what that might look like. But one can imagine a future in which machines wouldn’t just move products around a warehouse but navigate the real world. They wouldn’t just respond to commands but understand, and empathize with, humans. They wouldn’t just identify images; they’d create art. To that end, Bengio has been studying how the human brain operates. As one of his postdocs told me, brains “are proof that intelligent systems are possible.” One of Bengio’s pet projects is a game in which players teach a virtual child—the “Baby A.I.” from his whiteboard—about how the world operates by talking to the pretend infant, pointing, and so on: “We can use inspiration from how babies learn and how parents interact with their babies.” It seems far-fetched until you remember that Bengio’s once-outlandish notions now underpin some of Big Tech’s most mainstream technologies. While Bengio believes human-like A.I. is possible, he evinces impatience with the far-reaching ethical worries popularized by people like Elon Musk, premised on A.I.s outsmarting humans. Bengio is more interested in the ethical choices of the humans building and using A.I. “One of the greatest dangers is that people either deal with A.I. in an irresponsible way or maliciously—I mean for their personal gain,” he once told an interviewer. Other scientists share Bengio’s feelings, and yet, as A.I. research continues apace, it remains funded by the world’s most powerful governments, corporations, and investors. Bengio’s university lab is largely funded by Big Tech. At one point, during a discussion of the biggest tech companies, Bengio told me, “We want Element AI to become as large as one of these giants.” When I questioned whether he would then be perpetuating the same sort of concentration of wealth and power that he has decried, he replied, “The idea isn’t just to create one company and be the richest in the world. It’s to change the world, to change the way that business is done, to make it not as concentrated, to make it more democratic.” As much as I admired his position and believed in his intentions, his words didn’t sound much different from the corporate slogans once chosen by Big Tech. Don’t be evil. Make the world more open and connected. Creating an ethical business is less about founders’ intentions than about how, over time, business owners measure societal good against profit. What should we be doing? If computers are still struggling to answer that question, they should take some solace in knowing that we humans are not much better. This article originally appeared in the July 1, 2018 issue of Fortune. |