反智主义盛行,美国可能会输掉全球人工智能竞赛
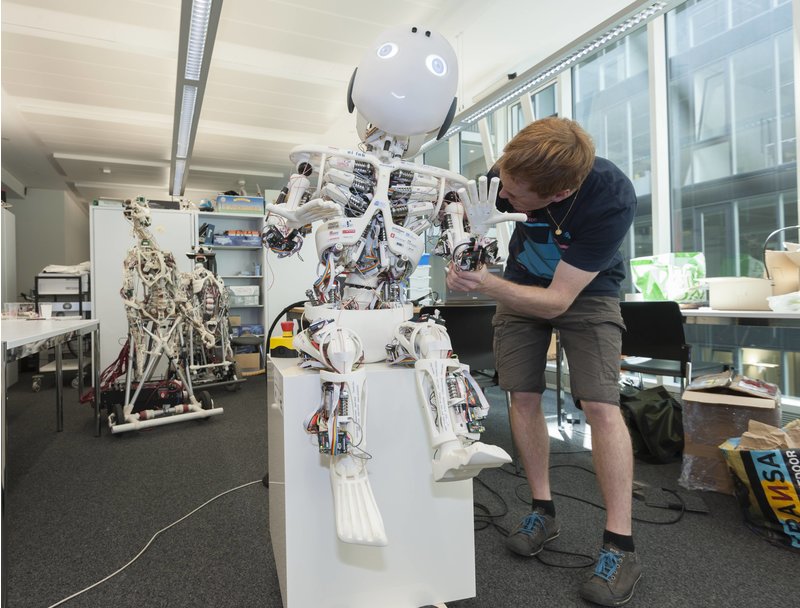
一个国家徜若在人工智能领域里赢得了全球的主导权,则必将获得巨大的经济利益,其经济增长率在2035年前甚至可能提高一倍。可惜美国在如何开展竞争上却没有得到很好的建议。 过去一年里,中、日、英、法、印、加等国都启动了由政府支持的大规模人工智能项目,以在该领域里拔得头筹。虽然特朗普政府也开始关注人工智能技术的发展,但在国家层面上,美国国内并未形成一个有凝聚力的战略与中日等国抗衡。美国政界反而兴起了一股反智主义,决策者们更多担心人工智能的潜在危害,呼吁给这项技术念“紧箍咒”的多,支持其发展的少。 人工智能确实给社会带来了一些独特的挑战——比如它有可能加剧刑事司法系统中的种族偏见,而自动驾驶汽车技术也产生了一些伦理问题。至于如何解决这些挑战,也有人给出了一些解决方案,目前最流行的观点,是要确立人工智能算法的透明性原则或可解释性原则,或者建立一个最高层级的人工智能监管机构。然而这些措施不仅可能无助于解决问题,反而会严重拖慢美国人工智能技术的发展和应用速度。 透明性原则的支持者们认为,应该要求人工智能公司公开源代码,让监管机构、记者和有责任心的公民可以对这些代码进行审查,从而发现任何不法行为的迹象。不过以人工智能系统的复杂性,我们很难相信这种方法会有什么效果,反而会使那些奉行“拿来主义”的国家的企业更容易偷到美国的原代码。这种做法显然会阻碍美国在全球人工智能竞赛中的竞争,使美国企业更不愿意投资这项技术。 可解释性原则的支持者则认为,政府应要求公司采取必要措施,使终端用户有能力解读他们的算法——比如描述算法的工作原理,或者只允许使用那些能够解释清楚其决策机制的算法。比如欧盟就把算法可解释性作为评估人工智能潜在风险的一个主要指标。欧盟的《通用数据保护条例》(GDPR)规定,一个自然人有权获得关于算法决策机制的“有意义的信息”。 可解释性原则可能是个合理的要求,而且它已经成为了刑事司法或消费金融等很多领域的标准。但是在某些领域里,你甚至无法要求一个自然人去解释他的决策机制,你又怎能将这个要求硬套在人工智能身上呢?非要这样的话,企业只得继续依赖真人进行决策,以避免监管压力,而这则会不可避免地造成效率和创新的迟滞。 另外,可解释性与准确性是鱼与熊掌的关系。一个算法越复杂,其准确性一般越高;但一个算法越复杂,它就越难以解释清楚。这种矛盾是始终存在的。两个变量的线性回归,肯定要比200个变量的线性回归容易解释。算法使用的数学模型越先进,这种矛盾就愈发尖锐。因此,可解释性原则只有在可以牺牲准确性的条件下才能实现,而这种条件显然太少了。比如对于自动驾驶汽车技术,为了可解释性而牺牲准确性,后果无疑是灾难性的。导航精度哪怕稍稍损失一点,或者计算机某一次不小心将行人和广告牌上的人像搞混了,都会造成巨大的危险。 另一个颇为流行的馊主意,是建立一个全国性的类似于美国食品与药品管理局(FDA)和美国国家运输交通安全委员会(NTSB)的人工智能监管机构。埃隆·马斯克就坚决支持这一倡议。持这种观点的人好像把搞人工智能当成了卖衣服和快餐,似乎认为所有人工智能算法都对社会有同样的危险性。然而人工智能系统的决策机制也跟人类一样,是受一系列行业法律法规约束的,其危险性有高有低,主要取决于它的应用场景。你不能只因为它是一个人工智能算法,就对一个低风险的人工智能产品搞监管,这必然会显著阻碍这项技术的研发,进而限制美国企业采用人工智能技术的能力。 好在政策制定者们还是有一种可行的办法,既能解决人工智能的潜在威胁,又不会阻碍它的发展——那就是算法责任原则。它是一种低干涉的监管模式,企业只需通过一系列控制机制,验证他们的人工智能系统是不是按照设计意图运行,能不能识别和纠正有害结果。它既不会像透明性原则那样危害知识产权,也不会像可解释性原则那样阻碍技术发展,企业仍然可以部署先进的创新人工智能系统。但在某些特殊情况下,根据实际需要,也可以要求企业解释其决策机制,不管人工智能系统在这些决策中有没有被使用。另外根据算法责任原则,各个行业的监管机构也将能够理解各自领域内的人工智能技术,而不需要建立一个全国性的最高监管机构。这样也就大大降低了人工智能部署的壁垒。 如果美国真的想领跑全球人工智能竞赛,那么政策制定者最应该避免的,就是用一套低效的监管制度扼杀人工智能的发展潜力。如果政策制定者担心人工智能的安全问题或是公平问题,他们完全可以采用算法责任原则来化解他们的担忧,而不是当美国刚站在人工智能竞赛的起跑线上,就去一棍子打断他的腿。(财富中文网) 本文作者乔舒亚·纽是智库机构数据创新中心(Center for Data Innovation)的高级政策研究分析师,该机构主要研究数据、科技与公共政策的交集。 译者:朴成奎 |
The country that wins the global race for dominance in artificial intelligence stands to capture enormous economic benefits, including potentially doubling its economic growth rates by 2035. Unfortunately, the United States is getting bad advice about how to compete. Over the past year, Canada, China, France, India, Japan, and the United Kingdom have all launched major government-backed initiatives to compete in AI. While the Trump administration has begun to focus on how to advance the technology, it has not developed a cohesive national strategy to match that of other countries. This has allowed the conversation about how policymakers in the United States should support AI to be dominated by proposals from advocates primarily concerned with staving off potential harms of AI by imposing restrictive regulations on the technology, rather than supporting its growth. AI does pose unique challenges—from potentially exacerbating racial bias in the criminal justice system to raising ethical concerns with self-driving cars—and the leading ideas to address these challenges are to mandate the principle of algorithmic transparency or algorithmic explainability, or to form an overarching AI regulator. However, not only would these measures likely be ineffective at addressing potential challenges, they would significantly slow the development and adoption of AI in the United States. Proponents of algorithmic transparency contend that requiring companies to disclose the source code of their algorithms would allow regulators, journalists, and concerned citizens to scrutinize the code and identify any signs of wrongdoing. While the complexity of AI systems leaves little reason to believethat this would actually be effective, it would make it significantly easier for bad actors in countries that routinely flout intellectual property protections, to steal U.S. source code. This would simultaneously give a leg up to the United States’ main competition in the global AI race and reduce incentives for U.S. firms to invest in developing AI. Others have proposed algorithmic explainability, where the government would require companies to make their algorithms interpretable to end users, such as by describing how their algorithms work or by only using algorithms that can articulate rationales for their decisions. For example, the European Union has made explainability a primary check on the potential dangers of AI, guaranteeing in its General Data Protection Regulation (GDPR) a person’s right to obtain “meaningful information” about certain decisions made by an algorithm. Requiring explainability can be appropriate, and it is already the standard in many domains, such as criminal justice or consumer finance. But extending this requirement to AI decision-making in circumstances where the same standard doesn’t apply for human decisions would be a mistake. It would incentivize businesses to rely on humans to make decisions so they can avoid this regulatory burden, which would come at the expense of productivity and innovation. Additionally, there can be inescapable trade-offs between explainability and accuracy. An algorithm’s accuracy typically increases with its complexity, but the more complex an algorithm is, the more difficult it is to explain. This trade-off has always existed—a simple linear regression with two variables is easier to explain than one with 200 variables—but the tradeoffs become more acute when using more advanced data science methods. Thus, explainability requirements would only make sense in situations where it is appropriate to sacrifice accuracy—and these cases are rare. For example, it would be a terrible idea to prioritize explainability over accuracy in autonomous vehicles, as even slight reductions in navigational accuracy or to a vehicle’s ability to differentiate between a pedestrian on the road and a picture of a person on a billboard could be enormously dangerous. A third popular, but bad idea, championed most notably by Elon Musk, is to create the equivalent of the Food and Drug Administration or National Transportation Safety Board to serve as an overarching AI regulatory body. The problem is that establishing an AI regulator falsely implies that all algorithms pose the same level of risk and need for regulatory oversight. However, an AI system’s decisions, like a human’s decisions, are still subject to a wide variety of industry-specific laws and regulation and pose a wide variety of risk depending on their application. Subjecting low-risk decisions to regulatory oversight simply because they use an algorithm would be a considerable barrier to deploying AI, limiting the ability of U.S. firms to adopt the technology. Fortunately, there is a viable way for policymakers to address the potential risks of AI without sabotaging it: Adopt the principle of algorithmic accountability, a light-touch regulatory approach that incentivizes businesses deploying algorithms to use a variety of controls to verify that their AI systems act as intended, and to identify and rectify harmful outcomes. Unlike algorithmic transparency, it would not threaten intellectual property. Unlike algorithmic explainability, it would allow companies to deploy advanced, innovative AI systems, yet still require that they be able to explain certain decisions when context demands it, regardless of whether AI was used in those decisions. And unlike a master AI regulator, algorithmic accountability would ensure regulators could understand AI within their sector-specific domains while limiting the barriers to AI deployment. If the United States is to be a serious contender in the global AI race, the last thing policymakers should do is shackle AI with ineffective, economically damaging regulation. Policymakers who want to focus now on unfair or unsafe AI should instead pursue the principle of algorithmic accountability as a means of addressing their concerns without kneecapping the United States as it enters the global AI race. Joshua New is a senior policy analyst at the Center for Data Innovation, a think tank studying the intersection of data, technology, and public policy. |