电子表格是一种非常巧妙的发明,诞生之初,其使命是实现簿记的数字化,自此而后的50年间,因为它的存在,研究人员与商业人士得以不受行、列数量限制任意输入各种数据,然后再借助计算机对这些信息进行分析。如今,电子表格被广泛应用于工作生活的各个方面,甚至连学童都可以像财务分析师管理预算一样使用这一工具。
不过电子表格没有思考能力,而这则是更新一代、功能也更强大的“神经网络”软件的专长(神经网络是一种复杂的人工智能程序,能够模拟人脑的计算过程)。近年来,由于神经网络的发展,顶尖人工智能研究人员关注的焦点已经从结构化数据(如成行成列的文字、数字)转向了图像。换句话说,功能强大的计算机可以通过浏览数百万张猫咪的照片来了解这种小型猫科动物的特征,但同样的软件却很难在简单的电子表格中直观地做到这一点。
这让医学研究、金融和运营等领域的数据科学家们深感沮丧,因为在这些领域,结构化数据才是真正的“硬通货”。金融公司Capital One的应用型机器学习研究人员巴彦•布鲁斯说:“我们的数据大多是结构化数据,或者至少是对这些数据进行了某种结构化处理。深度学习的进展与我们的数据之间有着很大距离,我们做的很多工作都是为了缩小这种距离。”
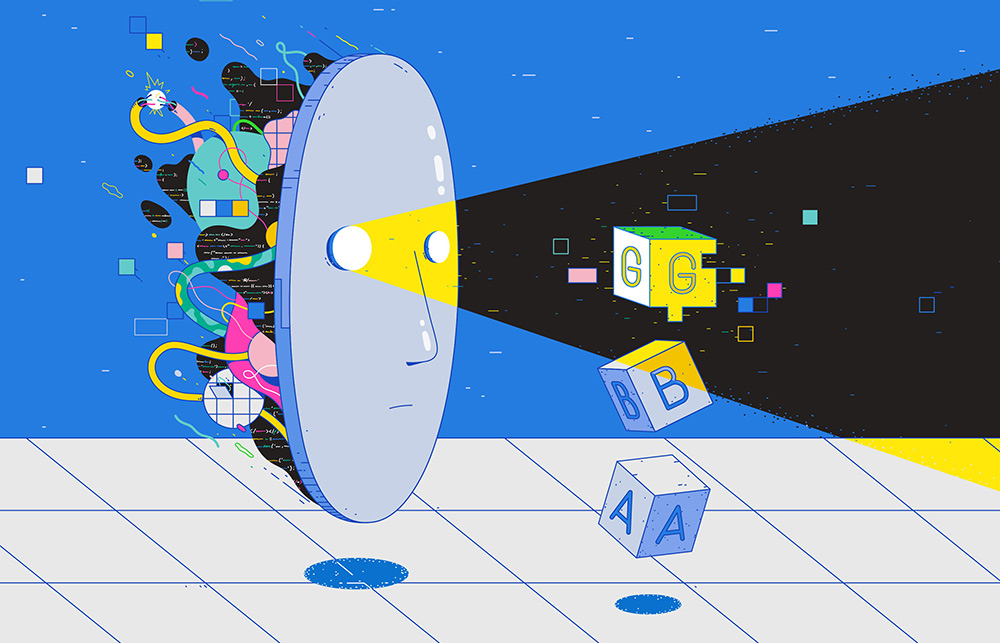
一些公司为解决这一问题也推出了自己的新项目。以生物技术巨头基因泰克为例,该公司的数据科学家最近花费数月时间制作了一个包含55,000名癌症患者健康记录和基因组数据的电子表格,既收录了年龄、胆固醇水平、心率等信息,也收录了一些更为复杂的属性数据,如分子特征和基因异常状况等。基因泰克计划将这些信息输入神经网络,并借此描绘出患者的健康属性,以期开发出突破性药物,针对每位患者的情况对症下药。
问题在于,研究人员现在才刚开始训练神经网络学习使用(像基因泰克制作的电子表格那样的)结构化数据。基因泰克的个性化医疗数据科学分析业务全球主管瑞安•科平表示:“包括临床试验数据和电子病历在内,我们的大多数数据都是结构化数据。”如果计算机网络能够分析并自主认知病人资料中的相似性,“那么我们就可以开始对结果进行观察,并考虑如何针对病人的具体情况选择治疗方案。然而,现在还做不到这一点。”
除医疗行业外,很多其它行业也有机会从中受益。据研究公司IDC估计,今年,商业领域将产生5.8泽字节的销售预测、客户数据等生产力数据。一个泽字节大致相当于全球所有海滩上沙粒的总数。也就是说,这是一个天文数字,IDC全球数据层项目(该项目负责计算全球每年产生的数据量)的负责人约翰•瑞德宁如是说。
这意味着,只要能够将数据压缩成神经网络可以学习的格式,那么各种类型的企业都将有机会从中获益。食品巨头百事公司的首席战略和转型官阿蒂纳•卡尼乌拉认为,预测能力的小幅提升也能够带来巨大的财务回报。她说:“准确度的增加将会带来数百万美元的收益。”
接下来的挑战则是要找到那些对商业活动最有价值的数据供研究人员使用。斯坦福大学教授、硅谷初创公司Sisu Data(该公司的主营业务是为企业开发分析工具)的首席执行官彼得•贝利斯说:“深度网络非常酷炫,在汽车、推文理解等领域都大有可为。但如果只是储存在表格中的数据,那么对我们在认知风险、了解客户满意度等方面的帮助就非常有限了。”如果换成商业人士都可以听懂的话,那么问题依然是:人工智能能否解决自己难以识别Excel内容的问题?
神经网络商业应用的推广进度取决于其是否能够像分析图像一样理解单词的含义。为解决这一问题,研究人员将目光转向了一种名为Word2vec的技术。(“vec”代表向量,是神经网络最擅长理解的分析单元类型。)Word2vec由谷歌的一个研究小组于2013年开发,并已作为开源软件项目对外发布,可以帮助计算机理解特定单词之间的联系。Word2vec技术为更强大语言系统的出现铺平了道路,这些新推出的系统已经能够识别出与“汽车”一词关系更密切的企业是宝马、尼桑这样的汽车制造商,而不是卡夫亨氏这样的食品公司。
word2vec之所以具备神奇的计算能力,是因为其可以将单词转换成神经网络能够理解的数字串,进而识别出词语之间的相关性。经过一段时间的训练,通过对更多文本进行学习,神经网络便具备了根据单词共同出现的频率对其进行打分的能力,并能够根据分数对单词进行分组。与更早出现的所谓自然语言处理技术相比,这些较新的系统提升了与人类思维典型相关的模式识别属性。
借助这种计算机辅助的单词联想游戏,计算机将可以理解表格中存储的信息。这个过程相当于为神经网络创建了一套自己的摩尔斯电码:当应用程序在一份有关销售情况的电子表格中遇到一列表示“日期”的数据时,无需获得明确指令,只要借助足够的数据,便能够理解某些假日可能会对特定季节的销售产生影响。旧金山大学应用数据伦理中心的主任、非营利教育机构Fast.ai的联合创始人雷切尔•托马斯表示:“这是底层的核心概念。神经网络通过建模特定形态的模式创造了一种无限灵活的学习架构。”
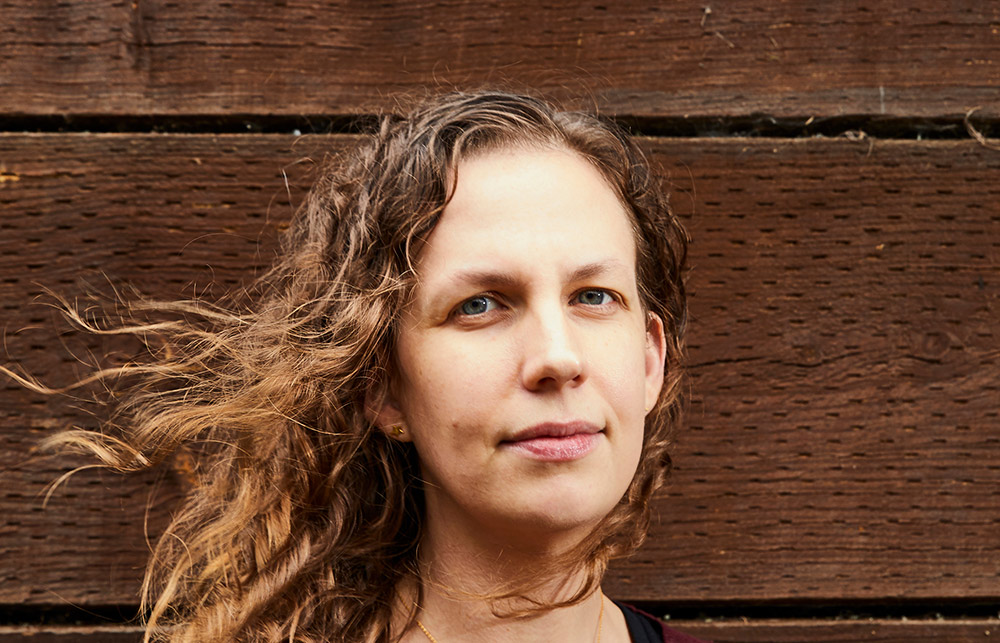
仅在投资领域就有大量通过文字分析创造价值的机会。高盛的一个研究小组正在对神经网络进行训练,使其获得搜寻“家庭房产内部转让”相关词汇的能力。在进行非商业性质的交易时,交易双方很可能不会如实描述房产的真实价值,如果可以教会软件在筛选资料时将相关信息排除在外,自然能够提高银行的分析能力。“为此,我们训练了一个可以识别此类交易、并减少对其关注程度的神经网络。”加州大学圣迭戈分校计算机科学专业的常任教授查尔斯•埃尔坎表示,直到最近,他还在负责领导高盛的机器学习项目。
复杂的词语联想对物流行业也有很大价值。旧金山外卖初创公司Instacart便使用了word2vec的一种变体技术,让自己的算法能够预测顾客的偏好,这一能力在公司无法提供顾客想要的产品时尤其有用。为方便神经网络处理相关信息,该公司使用的程序会将超市库存商品的“单词”转换成“数字形式的数据”,随后,神经网络会对相应物品进行分组,以便理解这些数据的意义:比如,(通过分组,神经网络会发现,)与咖啡相比,什锦干果与干果或坚果的共同点更多。Instacart的机器学习主管沙拉特•拉奥表示,使用这种技术帮助公司节约了时间和资金成本。他说:“不然我们就得思考所有可能的配对,还得留一张(手填)表。”
虽然在结构化数据领域应用深度学习技术已经是大势所趋,但障碍依然存在。首先,这是一个全新想法,此前并未对其效果进行过验证,没有人知道与更为传统的统计方法相比,这种技术能够有哪些优势。人工智能芯片生产公司英伟达的数据科学家伊文•奥尔德里奇说:“现在我们还不知道这个问题的答案。”
的确,考虑到训练神经网络的费用,对于那些不具备人工智能专长的企业来说,原有的数据分析方法可能已经够用了。百事公司高管、人工智能专家卡尼乌拉表说:“我坚信,这个世界上绝不存在可以解决所有问题的‘锦囊妙计’,对所有公司来说都是如此。”云服务巨头亚马逊、微软和谷歌在推销自己的服务时实际上也隐含着这层意思:与其投入巨资、招揽人才去争取潜在的增量回报,还不如直接从我们这里购买人工智能服务。
与其它以“教会计算机具备‘思考’能力”为目的的项目一样,人类的偏见也会对项目的成功构成威胁。深度学习系统的优劣取决于训练它们所用的数据,数据太多或太少都可能会使软件的预测产生偏差。以基因泰克的数据集为例,该数据集收入了此前15年的临床数据,但只收入了此前8年的基因组测试数据,也就是说,在此之前的患者数据并不像研究人员所希望的那样具有可比性。供职于基因泰克的科平说:“如果我们对这些数据集缺乏了解,那么据此建立起来的模型可能毫无可靠性可言。”
科平表示,尽管如此,对这些电子表格中的内容进行强化分析依然具有很高的潜在价值,其意义完全不亚于获得“预测一个病人在接受某种治疗之后能够存活多久”的能力。对一堆表格来说,可以做到这一点也算是不错的成绩了。
数家公司正在对神经网络进行训练,希望其能够处理自己已有的结构化数据,这些公司包括:
基因泰克
这家生物技术先驱企业制作了一份内含繁杂健康数据、覆盖数百万名患者的电子表格,从常规记录到基因组图谱,不一而足。这一研究具有重要意义:如果人工智能真可以通过正确方式分析这些数据,个体病患未来或将能够获得针其疾病制定的个性化治疗方案。
高盛
人工智能为投资者提供了无限机遇。受高盛聘请,一位机器学习专业的教授开发了一种训练工具,借助这种工具,神经网络能够学会忽略那些可能使金融分析复杂化的词语,如“家庭内部转让”(出现这一词语时,交易中的房产价值可能失真)。神经网络学会识别、忽略此类词语可以提升现有分析模型的效率。
Instacart
这家外卖初创公司拥有一套易于理解的数据集,内含员工需为顾客选取的各种超市商品。该公司正在训练算法进行复杂单词联想的能力,比如在看到什锦干果时,能够联想到坚果和干果,方便在顾客所需商品缺货时为其提供替代选择。(财富中文网)
本文另一版本登载于《财富》杂志2020年10月刊,标题为《是什么让人工智能看起来很蠢》。
译者:梁宇
审校:夏林
电子表格是一种非常巧妙的发明,诞生之初,其使命是实现簿记的数字化,自此而后的50年间,因为它的存在,研究人员与商业人士得以不受行、列数量限制任意输入各种数据,然后再借助计算机对这些信息进行分析。如今,电子表格被广泛应用于工作生活的各个方面,甚至连学童都可以像财务分析师管理预算一样使用这一工具。
不过电子表格没有思考能力,而这则是更新一代、功能也更强大的“神经网络”软件的专长(神经网络是一种复杂的人工智能程序,能够模拟人脑的计算过程)。近年来,由于神经网络的发展,顶尖人工智能研究人员关注的焦点已经从结构化数据(如成行成列的文字、数字)转向了图像。换句话说,功能强大的计算机可以通过浏览数百万张猫咪的照片来了解这种小型猫科动物的特征,但同样的软件却很难在简单的电子表格中直观地做到这一点。
这让医学研究、金融和运营等领域的数据科学家们深感沮丧,因为在这些领域,结构化数据才是真正的“硬通货”。金融公司Capital One的应用型机器学习研究人员巴彦•布鲁斯说:“我们的数据大多是结构化数据,或者至少是对这些数据进行了某种结构化处理。深度学习的进展与我们的数据之间有着很大距离,我们做的很多工作都是为了缩小这种距离。”
一些公司为解决这一问题也推出了自己的新项目。以生物技术巨头基因泰克为例,该公司的数据科学家最近花费数月时间制作了一个包含55,000名癌症患者健康记录和基因组数据的电子表格,既收录了年龄、胆固醇水平、心率等信息,也收录了一些更为复杂的属性数据,如分子特征和基因异常状况等。基因泰克计划将这些信息输入神经网络,并借此描绘出患者的健康属性,以期开发出突破性药物,针对每位患者的情况对症下药。
问题在于,研究人员现在才刚开始训练神经网络学习使用(像基因泰克制作的电子表格那样的)结构化数据。基因泰克的个性化医疗数据科学分析业务全球主管瑞安•科平表示:“包括临床试验数据和电子病历在内,我们的大多数数据都是结构化数据。”如果计算机网络能够分析并自主认知病人资料中的相似性,“那么我们就可以开始对结果进行观察,并考虑如何针对病人的具体情况选择治疗方案。然而,现在还做不到这一点。”
除医疗行业外,很多其它行业也有机会从中受益。据研究公司IDC估计,今年,商业领域将产生5.8泽字节的销售预测、客户数据等生产力数据。一个泽字节大致相当于全球所有海滩上沙粒的总数。也就是说,这是一个天文数字,IDC全球数据层项目(该项目负责计算全球每年产生的数据量)的负责人约翰•瑞德宁如是说。
这意味着,只要能够将数据压缩成神经网络可以学习的格式,那么各种类型的企业都将有机会从中获益。食品巨头百事公司的首席战略和转型官阿蒂纳•卡尼乌拉认为,预测能力的小幅提升也能够带来巨大的财务回报。她说:“准确度的增加将会带来数百万美元的收益。”
接下来的挑战则是要找到那些对商业活动最有价值的数据供研究人员使用。斯坦福大学教授、硅谷初创公司Sisu Data(该公司的主营业务是为企业开发分析工具)的首席执行官彼得•贝利斯说:“深度网络非常酷炫,在汽车、推文理解等领域都大有可为。但如果只是储存在表格中的数据,那么对我们在认知风险、了解客户满意度等方面的帮助就非常有限了。”如果换成商业人士都可以听懂的话,那么问题依然是:人工智能能否解决自己难以识别Excel内容的问题?
神经网络商业应用的推广进度取决于其是否能够像分析图像一样理解单词的含义。为解决这一问题,研究人员将目光转向了一种名为Word2vec的技术。(“vec”代表向量,是神经网络最擅长理解的分析单元类型。)Word2vec由谷歌的一个研究小组于2013年开发,并已作为开源软件项目对外发布,可以帮助计算机理解特定单词之间的联系。Word2vec技术为更强大语言系统的出现铺平了道路,这些新推出的系统已经能够识别出与“汽车”一词关系更密切的企业是宝马、尼桑这样的汽车制造商,而不是卡夫亨氏这样的食品公司。
word2vec之所以具备神奇的计算能力,是因为其可以将单词转换成神经网络能够理解的数字串,进而识别出词语之间的相关性。经过一段时间的训练,通过对更多文本进行学习,神经网络便具备了根据单词共同出现的频率对其进行打分的能力,并能够根据分数对单词进行分组。与更早出现的所谓自然语言处理技术相比,这些较新的系统提升了与人类思维典型相关的模式识别属性。
借助这种计算机辅助的单词联想游戏,计算机将可以理解表格中存储的信息。这个过程相当于为神经网络创建了一套自己的摩尔斯电码:当应用程序在一份有关销售情况的电子表格中遇到一列表示“日期”的数据时,无需获得明确指令,只要借助足够的数据,便能够理解某些假日可能会对特定季节的销售产生影响。旧金山大学应用数据伦理中心的主任、非营利教育机构Fast.ai的联合创始人雷切尔•托马斯表示:“这是底层的核心概念。神经网络通过建模特定形态的模式创造了一种无限灵活的学习架构。”
仅在投资领域就有大量通过文字分析创造价值的机会。高盛的一个研究小组正在对神经网络进行训练,使其获得搜寻“家庭房产内部转让”相关词汇的能力。在进行非商业性质的交易时,交易双方很可能不会如实描述房产的真实价值,如果可以教会软件在筛选资料时将相关信息排除在外,自然能够提高银行的分析能力。“为此,我们训练了一个可以识别此类交易、并减少对其关注程度的神经网络。”加州大学圣迭戈分校计算机科学专业的常任教授查尔斯•埃尔坎表示,直到最近,他还在负责领导高盛的机器学习项目。
复杂的词语联想对物流行业也有很大价值。旧金山外卖初创公司Instacart便使用了word2vec的一种变体技术,让自己的算法能够预测顾客的偏好,这一能力在公司无法提供顾客想要的产品时尤其有用。为方便神经网络处理相关信息,该公司使用的程序会将超市库存商品的“单词”转换成“数字形式的数据”,随后,神经网络会对相应物品进行分组,以便理解这些数据的意义:比如,(通过分组,神经网络会发现,)与咖啡相比,什锦干果与干果或坚果的共同点更多。Instacart的机器学习主管沙拉特•拉奥表示,使用这种技术帮助公司节约了时间和资金成本。他说:“不然我们就得思考所有可能的配对,还得留一张(手填)表。”
虽然在结构化数据领域应用深度学习技术已经是大势所趋,但障碍依然存在。首先,这是一个全新想法,此前并未对其效果进行过验证,没有人知道与更为传统的统计方法相比,这种技术能够有哪些优势。人工智能芯片生产公司英伟达的数据科学家伊文•奥尔德里奇说:“现在我们还不知道这个问题的答案。”
的确,考虑到训练神经网络的费用,对于那些不具备人工智能专长的企业来说,原有的数据分析方法可能已经够用了。百事公司高管、人工智能专家卡尼乌拉表说:“我坚信,这个世界上绝不存在可以解决所有问题的‘锦囊妙计’,对所有公司来说都是如此。”云服务巨头亚马逊、微软和谷歌在推销自己的服务时实际上也隐含着这层意思:与其投入巨资、招揽人才去争取潜在的增量回报,还不如直接从我们这里购买人工智能服务。
与其它以“教会计算机具备‘思考’能力”为目的的项目一样,人类的偏见也会对项目的成功构成威胁。深度学习系统的优劣取决于训练它们所用的数据,数据太多或太少都可能会使软件的预测产生偏差。以基因泰克的数据集为例,该数据集收入了此前15年的临床数据,但只收入了此前8年的基因组测试数据,也就是说,在此之前的患者数据并不像研究人员所希望的那样具有可比性。供职于基因泰克的科平说:“如果我们对这些数据集缺乏了解,那么据此建立起来的模型可能毫无可靠性可言。”
科平表示,尽管如此,对这些电子表格中的内容进行强化分析依然具有很高的潜在价值,其意义完全不亚于获得“预测一个病人在接受某种治疗之后能够存活多久”的能力。对一堆表格来说,可以做到这一点也算是不错的成绩了。
数家公司正在对神经网络进行训练,希望其能够处理自己已有的结构化数据,这些公司包括:
基因泰克
这家生物技术先驱企业制作了一份内含繁杂健康数据、覆盖数百万名患者的电子表格,从常规记录到基因组图谱,不一而足。这一研究具有重要意义:如果人工智能真可以通过正确方式分析这些数据,个体病患未来或将能够获得针其疾病制定的个性化治疗方案。
高盛
人工智能为投资者提供了无限机遇。受高盛聘请,一位机器学习专业的教授开发了一种训练工具,借助这种工具,神经网络能够学会忽略那些可能使金融分析复杂化的词语,如“家庭内部转让”(出现这一词语时,交易中的房产价值可能失真)。神经网络学会识别、忽略此类词语可以提升现有分析模型的效率。
Instacart
这家外卖初创公司拥有一套易于理解的数据集,内含员工需为顾客选取的各种超市商品。该公司正在训练算法进行复杂单词联想的能力,比如在看到什锦干果时,能够联想到坚果和干果,方便在顾客所需商品缺货时为其提供替代选择。(财富中文网)
本文另一版本登载于《财富》杂志2020年10月刊,标题为《是什么让人工智能看起来很蠢》。
译者:梁宇
审校:夏林
The electronic spreadsheet has been around for about 50 years. An ingenious invention originally meant to digitize bookkeeping, the software has enabled researchers and businesspeople to input infinite rows and columns of disparate data and then analyze the information with the aid of a computer. It is such standard fare today that schoolchildren are as likely to use free spreadsheet programs as financial analysts are to manage budgets.
What spreadsheets cannot do is think. That’s the preserve of newer, more powerful types of software called neural networks, complex artificial intelligence programs designed to mimic the computational processes of the human brain. And for reasons unique to the development of neural networks in recent years, images—rather than so-called structured data, columns and rows of text and numbers, for example—have been the preoccupation of top A.I. researchers. In other words, powerful computers can sift through millions of photos of cats to understand minute feline characteristics. But the same software struggles to intuit fields in a humble spreadsheet.
This has been deeply frustrating to data scientists in fields like medical research, finance, and operations, where structured data is the coin of the realm. The problem, researchers say, is one of emphasis as well as capabilities. “Most of data we deal with is structured, or we have imposed some kind of structure on it,” says Bayan Bruss, an applied machine learning researcher at the financial firm Capital One. “There’s this big gap between the advances in deep learning and the data that we have. A lot of what we do is try to close that gap.”
Fledgling projects at a handful of companies are trying to bridge the divide. At biotech powerhouse Genentech, for example, data scientists recently spent months building a spreadsheet with the health records and genomic data of 55,000 cancer patients. The fields contain nuggets such as age, cholesterol levels, and heart rates, as well as more sophisticated attributes like molecular profiles and genetic abnormalities. Genentech’s plan is to feed this information into a neural network that can map a patient’s health attributes. The hoped-for outcome is a breakthrough drug that is potentially unique to each patient.
The problem is that researchers are just now beginning to teach neural networks how to consume structured data like the spreadsheets Genentech is building. “The majority of our data is structured data, whether it’s from clinical trials or electronic health records,” says Ryan Copping, global head of analytics for personalized health care data science at Genentech. If computer networks can analyze and make their own realizations about similarities among patient profiles, he says, “then you could start looking at outcomes and thinking about which patients we can target with which therapies. That’s the unmet need.”
The opportunities extend far beyond health care. Research firm IDC estimates the commercial sector will generate 5.8 zettabytes of productivity data—sales forecasts, customer data, and the like—this year. A zettabyte of information corresponds roughly to the number of grains of sand on all the world’s beaches. A lot, in other words, says John Rydning, head of IDC’s Global DataSphere program, which measures the amount of data created each year.
This means that businesses of all types, if they can corral the data into a form neural networks can learn from, have a lucrative opportunity. Even slight improvements in predictive capabilities can lead to enormous financial gains, says Athina Kanioura, chief strategy and transformation officer for food giant PepsiCo. “The additional level of accuracy translates to millions of dollars,” she says.
The challenge, then, is getting researchers to work with the kind of data that can be most helpful to business. “The deep networks that are so cool can really do amazing things for our cars and for understanding sentiment from tweets online,” says Peter Bailis, a Stanford professor and also CEO of a Silicon Valley startup called Sisu Data that builds analytical tools for businesses. “But they don’t help us with understanding things like risk or customer satisfaction if our data is stored in tables.” In terms any businessperson can relate to, the question remains: Can A.I. conquer its Excel problem?
*****
Progress in promoting business applications for neural networks rests on getting the programs to understand words as well as they have been able to analyze images. For that, researchers have turned to a technique called word2vec. (The “vec” stands for vector, the type of analytical unit best understood by a neural network.) Word2vec, invented in 2013 by a team of Google researchers and published as an open-source software project, helps computers map the relationships among certain words. It has led to more powerful language systems that recognize, for example, that the word “car” is more closely related to automakers like BMW or Nissan than a food company like Kraft Heinz.
The computational magic of word2vec is its ability to discover those correlations by converting words into a string of numbers that neural networks can understand. Over time, as a neural network is trained on additional text, it groups words according to numerical scores measuring how frequently the words appear near each other. Compared with older so-called natural language processing technologies, these newer systems improve on the pattern recognition attributes typically associated with human thought.
From this computer-assisted word-association game comes an ability to make sense of what is stored in the rows and columns, for instance, of a spreadsheet. This process creates a type of Morse code for a neural network: If the program comes across a sales spreadsheet with a column indicating “days,” it can learn with enough data that certain holidays could impact sales during a particular season without being explicitly told to do so. “It’s kind of the core idea,” says Rachel Thomas, director of the University of San Francisco’s Center for Applied Data Ethics and cofounder of an educational nonprofit called Fast.ai. “Neural networks are providing this infinitely flexible architecture for learning by modeling a particular shape of patterns.”
The investment world alone is rife with opportunities for analyzing words. At Goldman Sachs, a team of researchers trained a neural network to look for words associated with intra-family home transfers. Such noncommercial transactions likely won’t describe the true value of a house, and teaching a software program to factor them out can improve the bank’s analysis. “So we trained a neural network so it learns to pay less attention to a transaction that has that label,” says Charles Elkan, a longtime professor of computer science at the University of California at San Diego who until recently led machine learning projects for Goldman.
Sophisticated word association is also invaluable for logistics operators. The San Francisco grocery-delivery startup Instacart uses a variant of word2vec to teach its algorithms to anticipate customer preferences, particularly when requested items are unavailable. The program converts the words for supermarket inventory items into numerical data so neural networks can process them. The network then groups items together so it can understand, for example, that trail mix has more in common with dried fruit or nuts than it does with coffee. The result is a time and money saver, says Sharath Rao, a machine learning director for Instacart. “Otherwise you would have to think of all the possible pairs and keep a [manual] table,” he says.
*****
For all the momentum behind using deep learning on structured data, hurdles remain. For one, the idea is so new that there’s no tried-and-true way to evaluate how good these techniques are compared with more conventional statistical methods. “It’s a bit of an open question right now,” says Even Oldridge, a data scientist for Nvidia, which makes chips that power A.I. software.
Indeed, given the expense of training neural networks, older data analytics methods may be sufficient for companies that don’t have the right A.I. expertise in-house. “I’m a firm believer that for every company, there isn’t a magic solution that can solve every problem,” says A.I. expert Kanioura, the PepsiCo executive. This is in fact behind the pitch that cloud-services giants Amazon, Microsoft, and Google make: Buy A.I. services from us rather than making large expenditures on talent for potentially incremental returns.
And as with any project where humans aim to teach computers how to “think,” the biases of the living organisms threaten the project. Deep learning systems are only as good as the software’s predictions. Genentech’s data set, for instance, has clinical data on cancer patients dating back 15 years. However, the genomic testing data it uses in its spreadsheet is eight years old, meaning that patient data from before then isn’t as comparable as researchers might like. “If we don’t understand these data sets, we could build models that are totally unreliable,” says Genentech’s Copping.
Still, the potential value of supercharging the analysis of all those spreadsheet fields is nothing less than being able to “predict how long a patient can survive” with a certain treatment, says Copping. Not bad for a bunch of rows and columns.
*****
A handful of corporations are teaching neural networks to work with the kind of structured data that already exists within their walls. A few examples:
Genentech
The biotech pioneer has built a spreadsheet with complex health data from routine records to genomic profiles—from tens of thousands of patients. The stakes are high: If artificial intelligence can properly analyze the data, the result could be medical treatments targeting the disease of iindividual patients.
Goldman Sachs
A. I. presents untold opportunities for investors. The bank hired a machine learning professor to build a tool to teach networks to ignore phrases that could complicate a financial analysis. Example: “Intra-family transfers” likely don’t reflect the accurate value of a home. Teaching a network to find them can improve the model.
Instacart
The grocery-delivery startup has an understandable data set in the inventory of supermarket items its workers pick for customers. The company is teaching its algorithms to do sophisticated word association like matching trail mix with nuts and dried fruit—in order to offer customers alternatives when their choices are out of stock.
A version of this article appears in the October 2020 issue of Fortune with the headline "What makes artificial intelligence look dumb."