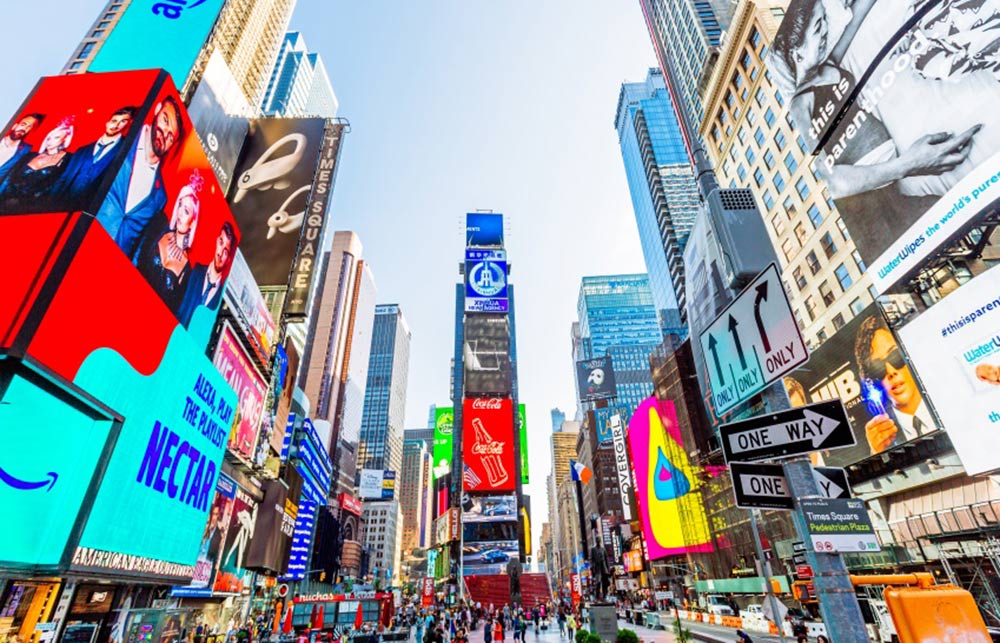
大众媒体一直在警告我们,通过使用最新的跟踪和人工智能技术,那些令人厌恶的营销人员以及其他不良动机者获得了预测甚至左右人们选择的能力。
例如,Netflix 2019年的纪录片《隐私大盗》(The Great Hack)讲述了一个案例:数据分析公司Cambridge Analytica通过搜刮社交媒体信息来获取有关个人想法的深度洞见。Netflix认为,该公司利用这些洞见可以设计有着明确目标的广告,继而操控2016年美国总统选举向有利于唐纳德•特朗普的方向发展。知名科技投资者罗杰•麦克纳米在讨论该纪录片中所描述的事件时断言,科技公司“都有一个数据诅咒人偶,它以数字的形式完整再现了人们的生活。借助这个人偶,这些公司能够左右人们的行为。”
同样,哈佛大学的心理学家肖珊娜•祖波夫最近发出了有关数字营销人员的警告:“他们的目的不仅仅是为了了解你的行为,同时还会塑造行为,从而将预测转变为必然性……如今,他们的目标是让消费者成为自动化工具。”
很明显,这种坏人会做坏事而且擅长做坏事的理念往往会让公众联想到阴谋论,而且也符合公众对阴谋论的认知。然而,我和斯坦福大学的营销学教授伊塔马•西蒙森最近在《消费者心理评论》(Consumer Psychology Review)发表的文章中指出,当我们更进一步研究这个问题时,我们发现上述指控过于夸张。
毫无疑问的是,通过人工智能(基本上是机器学习方法)获得的进步让诸多领域发生了革命性的变化,包括图像识别、语言翻译等等。然而,预测人类的选择(以及人类的普通行为)与人工智能擅长的那些任务有很大的差异。人们对特定产品和属性的喜好并非是可以预测的现实存在,它往往形成于做出决定的过程中,这种喜好有别于人工智能其他任务的目标。
具体来说,尽管人们可能会有普适的产品喜好(例如独特性、便于使用、质量、喜爱的颜色),但对于特定产品来说,或者人们在产品特性之间进行取舍时,这种喜好并非是确切的,难以被精确定义。
例如,人们不大可能在购买吐司机之前就对某个型号或吐司机的参数存在具体的喜好。同样,人们也不大可能对于自己是否愿意花更多的钱购买一个更有吸引力的吐司机有一个明确的概念,除非他们正在决定是否要购买。
这一现象在当前的消费信息环境中尤为普遍,消费者会在首次做出决定时,或即将做出决定之前,越发频繁地遭遇影响其选择的众多决定性因素(例如专家和用户评论、产品推荐、新选项),因此我们无法对其提前预测。例如,在购物过程中,一名消费者可能会看到产品的评论,重点介绍某个看似不怎么重要的特色能够给消费者带来的福利,但消费者此前从未考虑过这个因素。然而,这个介绍可能会对消费者的选择带来重大影响。这类恰逢其时的信息影响让选择预测的难度变得越来越大,而不是越来越小。
诚然,在这些案例中,消费者对于特定产品或属性的喜好并不强烈、精确和稳定。例如,一些人每天早上更愿意买一杯拿铁。在这种场景下,进行预测相对来说比较容易,而且对于数据或方法的要求也不是很高。
同样,在部分案例中,某些变量可以预测出不同消费群体存在的不同喜好。例如,相对于购买PlayStation的消费者,购买Xbox的消费者对Xbox游戏广告的接受度可能更高。在这个范围内,随着我们当前对人类行为(例如购买、“喜好”、访问等)跟踪的越多,此类预测的难度也就越小。
然而,即便拥有广泛的目标消费群体数据,预测谁必然会购买某种产品的能力依然很低。例如在最近Facebook举行的活动中,数亿用户收到了个性化(基于其在Facebook的历史喜好)的美容产品广告,在观看广告的人群中,购买该产品的人数比例平均只有万分之一点五左右。
的确,这个结果要比收看非个性化广告然后购买产品的人群比例高出约50%。换句话说,个性化的推送会让人们在看过广告后购买广告产品的概率从约万分之一升至万分之一点五。从经济角度而言,成功率的上述变化可能难能可贵(取决于广告的成本以及产品的利润率)。然而,这与拥有能够操控消费者或实现其“自动化”的“数据诅咒玩偶”还相距甚远。
在其他环境下,使用异常复杂的机器学习(深度学习)方法在改善人类选择预测方面并没有比使用基本的数据统计法好多少。例如,最近的研究发现,与使用简单的模型相比,使用更加复杂的模型在改善信用卡选择预测能力方面收效甚微,如果考虑成本的话,后者基本上是在浪费精力。
消费者喜好预测能力有限的另一个案例在于,我们不妨看看(缺乏)推荐引擎取得的进步,例如Netflix或亚马逊(Amazon)根据观众观看或购买习惯引导观众查看新节目或产品的引擎。最近发表的两篇评估报告认为,大多数通过愈发复杂的方法实现的所谓预测精准度改善都是“莫须有的进展”。他们发现,简单的方法所发挥的作用往往与更加复杂的方法没有什么区别,其中一篇报告总结说:“尽管模型的计算复杂度在不断增加,但进步似乎依然很有限……”
对于消费者和决策者来说,有限的预测能力以及对个人选择有限的影响在某种程度上应该让人感到欣慰。另一方面,在当前的信息环境下,消费者和决策者在构建其喜好并做出选择之前,有必要对评论操纵以及他们日渐依赖的其他信息保持警惕。
换句话说,我们不用太担心营销人员会知道我们到底想要什么(或者通过按哪个按钮来实现操控我们的目的),而且应该去关注自己在做出选择时所愈发依赖的信息的完整性。(财富中文网)
大卫•盖尔是伊利诺伊大学芝加哥分校的营销学教授。
译者:冯丰
审校:夏林
大众媒体一直在警告我们,通过使用最新的跟踪和人工智能技术,那些令人厌恶的营销人员以及其他不良动机者获得了预测甚至左右人们选择的能力。
例如,Netflix 2019年的纪录片《隐私大盗》(The Great Hack)讲述了一个案例:数据分析公司Cambridge Analytica通过搜刮社交媒体信息来获取有关个人想法的深度洞见。Netflix认为,该公司利用这些洞见可以设计有着明确目标的广告,继而操控2016年美国总统选举向有利于唐纳德•特朗普的方向发展。知名科技投资者罗杰•麦克纳米在讨论该纪录片中所描述的事件时断言,科技公司“都有一个数据诅咒人偶,它以数字的形式完整再现了人们的生活。借助这个人偶,这些公司能够左右人们的行为。”
同样,哈佛大学的心理学家肖珊娜•祖波夫最近发出了有关数字营销人员的警告:“他们的目的不仅仅是为了了解你的行为,同时还会塑造行为,从而将预测转变为必然性……如今,他们的目标是让消费者成为自动化工具。”
很明显,这种坏人会做坏事而且擅长做坏事的理念往往会让公众联想到阴谋论,而且也符合公众对阴谋论的认知。然而,我和斯坦福大学的营销学教授伊塔马•西蒙森最近在《消费者心理评论》(Consumer Psychology Review)发表的文章中指出,当我们更进一步研究这个问题时,我们发现上述指控过于夸张。
毫无疑问的是,通过人工智能(基本上是机器学习方法)获得的进步让诸多领域发生了革命性的变化,包括图像识别、语言翻译等等。然而,预测人类的选择(以及人类的普通行为)与人工智能擅长的那些任务有很大的差异。人们对特定产品和属性的喜好并非是可以预测的现实存在,它往往形成于做出决定的过程中,这种喜好有别于人工智能其他任务的目标。
具体来说,尽管人们可能会有普适的产品喜好(例如独特性、便于使用、质量、喜爱的颜色),但对于特定产品来说,或者人们在产品特性之间进行取舍时,这种喜好并非是确切的,难以被精确定义。
例如,人们不大可能在购买吐司机之前就对某个型号或吐司机的参数存在具体的喜好。同样,人们也不大可能对于自己是否愿意花更多的钱购买一个更有吸引力的吐司机有一个明确的概念,除非他们正在决定是否要购买。
这一现象在当前的消费信息环境中尤为普遍,消费者会在首次做出决定时,或即将做出决定之前,越发频繁地遭遇影响其选择的众多决定性因素(例如专家和用户评论、产品推荐、新选项),因此我们无法对其提前预测。例如,在购物过程中,一名消费者可能会看到产品的评论,重点介绍某个看似不怎么重要的特色能够给消费者带来的福利,但消费者此前从未考虑过这个因素。然而,这个介绍可能会对消费者的选择带来重大影响。这类恰逢其时的信息影响让选择预测的难度变得越来越大,而不是越来越小。
诚然,在这些案例中,消费者对于特定产品或属性的喜好并不强烈、精确和稳定。例如,一些人每天早上更愿意买一杯拿铁。在这种场景下,进行预测相对来说比较容易,而且对于数据或方法的要求也不是很高。
同样,在部分案例中,某些变量可以预测出不同消费群体存在的不同喜好。例如,相对于购买PlayStation的消费者,购买Xbox的消费者对Xbox游戏广告的接受度可能更高。在这个范围内,随着我们当前对人类行为(例如购买、“喜好”、访问等)跟踪的越多,此类预测的难度也就越小。
然而,即便拥有广泛的目标消费群体数据,预测谁必然会购买某种产品的能力依然很低。例如在最近Facebook举行的活动中,数亿用户收到了个性化(基于其在Facebook的历史喜好)的美容产品广告,在观看广告的人群中,购买该产品的人数比例平均只有万分之一点五左右。
的确,这个结果要比收看非个性化广告然后购买产品的人群比例高出约50%。换句话说,个性化的推送会让人们在看过广告后购买广告产品的概率从约万分之一升至万分之一点五。从经济角度而言,成功率的上述变化可能难能可贵(取决于广告的成本以及产品的利润率)。然而,这与拥有能够操控消费者或实现其“自动化”的“数据诅咒玩偶”还相距甚远。
在其他环境下,使用异常复杂的机器学习(深度学习)方法在改善人类选择预测方面并没有比使用基本的数据统计法好多少。例如,最近的研究发现,与使用简单的模型相比,使用更加复杂的模型在改善信用卡选择预测能力方面收效甚微,如果考虑成本的话,后者基本上是在浪费精力。
消费者喜好预测能力有限的另一个案例在于,我们不妨看看(缺乏)推荐引擎取得的进步,例如Netflix或亚马逊(Amazon)根据观众观看或购买习惯引导观众查看新节目或产品的引擎。最近发表的两篇评估报告认为,大多数通过愈发复杂的方法实现的所谓预测精准度改善都是“莫须有的进展”。他们发现,简单的方法所发挥的作用往往与更加复杂的方法没有什么区别,其中一篇报告总结说:“尽管模型的计算复杂度在不断增加,但进步似乎依然很有限……”
对于消费者和决策者来说,有限的预测能力以及对个人选择有限的影响在某种程度上应该让人感到欣慰。另一方面,在当前的信息环境下,消费者和决策者在构建其喜好并做出选择之前,有必要对评论操纵以及他们日渐依赖的其他信息保持警惕。
换句话说,我们不用太担心营销人员会知道我们到底想要什么(或者通过按哪个按钮来实现操控我们的目的),而且应该去关注自己在做出选择时所愈发依赖的信息的完整性。(财富中文网)
大卫•盖尔是伊利诺伊大学芝加哥分校的营销学教授。
译者:冯丰
审校:夏林
Popular media has been warning us about the ability of unsavory marketers and other bad actors to predict and even control our choices using the latest in tracking and artificial intelligence technologies.
In the 2019 Netflix documentary The Great Hack, for instance, the case is made that the data analytics firm Cambridge Analytica scraped social media to gain deep insights into individuals’ psyches. Using these insights, the filmmakers argue, that firm was able to design carefully targeted ads to manipulate the 2016 U.S. Presidential election in favor of Donald Trump. In discussing the events depicted in the film, the well-known technology investor Roger McNamee averred that technology companies “have a data voodoo doll, which is a complete digital representation of our lives. With it, they can manipulate our behavior.”
Likewise, the Harvard psychologist Shoshana Zuboff recently warned of digital marketers, “The idea is not only to know our behavior but also to shape it in ways that can turn predictions into guarantees… the goal now is to automate us.”
Clearly, the idea that bad people do bad things and are adept at it resonates and is consistent with the public’s inclination to conspiracy theories. But as Stanford marketing professor Itamar Simonson and I discuss in a recent articlein Consumer Psychology Review, a closer examination suggests that the claims are grossly exaggerated.
There is no question that advances that go under the label of A.I. (mostly machine learning methods) are enabling revolutions in many domains, including image recognition, language translation, and many others. However, predicting people’s choices (and human behavior generally) is quite unlike the tasks where A.I. shines. Unlike the targets of these other tasks, preferences for specific products and attributes do not exist to be predicted but tend to be formed at the time decisions are made.
To elaborate, while people are likely to have general product preferences (for uniqueness, for ease-of-use, for quality, for a favorite color), people usually do not have precise, well-defined preferences for specific products, or for how they would trade off one product attribute for another.
For example, people are unlikely to have a preference in advance of buying a toaster for a particular model or configuration of toaster. Likewise, they are unlikely to have a clear preference for how much extra they would be willing to pay for a somewhat more attractive toaster, until they are in the process of making a purchase decision. That is, such preferences do not exist to be predicted but are “constructed” in the process of making a decision on the basis of many, largely unpredictable factors.
This is particularly the case in the current consumer information environment, where many of the key determinants of choice (e.g., expert and user reviews, product recommendations, new options) are increasingly encountered by the consumer for the first time at or near the time when a decision is being made, and therefore cannot be anticipated ahead of time. For example, in the process of shopping, a consumer might encounter a product review that highlights the benefits of a seemingly insignificant feature the consumer previously had not considered, and this might substantially affect the consumer’s choice. The influence of such just-in-time information makes our choices increasingly more difficult, not easier, to predict.
To be sure, in some cases consumers do have strong, precise, stable preferences for particular products or attributes. For instance, some people prefer to buy a latte every morning. In such a case making a prediction is relatively easy, and requires little sophistication in data or methods.
Likewise, in some cases, certain variables will predict differences in preferences between consumer groups. For instance, consumers who bought an Xbox are likely to be much more receptive to ads for Xbox games than consumers who bought a PlayStation. Insofar as more of what we do (purchases, “likes,” visits, etc.) is tracked today, more such “easy” predictions can be made.
However, even with extensive consumer data for targeting, the ability to predict who is likely to buy a product in an absolute sense remains low. In a recent Facebook campaign, for instance, where millions of users were shown ads for a beauty product that were targeted to their personalities (based on their history of Facebook likes), on average only about 1.5 in 10,000 people that viewed the ads bought the product.
Granted, this result was about 50% higher than for people who saw the ad but were not targeted based on their personality. In other words, targeting based on personality increased the likelihood that someone who saw an ad would buy the advertised product from about 1 in 10,000 to about 1.5 in 10,000. Such a change in the success rate might be economically meaningful (depending on the cost of the ads and the product’s profit margins), but it is a far cry from having a “data voodoo doll” to manipulate consumers or to “automate” them.
In other contexts, the use of highly sophisticated machine learning (deep learning) methods has shown limited ability to improve predictions of people’s choices over basic statistical methods. For instance, recent research found that the use of more sophisticated models yielded only very slight improvements over a simple model in the ability to predict people’s credit card choices– so slight that, given the cost involved, it was likely a waste of effort.
As another example of the limited ability to predict consumer preferences, consider (the lack of) advances in recommendation engines, like those used by Netflix or Amazon to steer viewers toward new shows or products based on what they’ve already watched or purchased. Two recent reviews referred to most of the claimed gains in predictive accuracy from increasingly sophisticated methods as “phantom progress.” Simple methods, they found, tended to perform as well as more sophisticated ones, with one review concluding that, “progress seems to be still limited… despite the increasing computational complexity of the models.”
For consumers and policymakers, the limited ability to predict and thereby influence individual choices should be somewhat comforting. On the other hand, consumers and policymakers need to be vigilant regarding the manipulation of the reviews and other information that consumers increasingly depend on in the current information environment to construct their preferences and make choices.
In other words, we should be less concerned that marketers will know exactly what we want (or exactly what buttons to push to manipulate us) and more concerned about the integrity of the information we increasingly rely on to make choices.
David Gal is professor of marketing at the University of Illinois at Chicago.