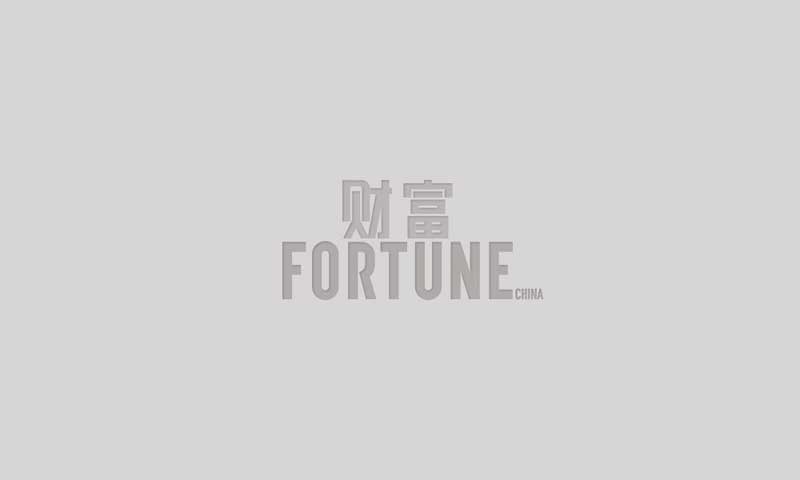
OpenAI公布了备受期待的最新系列人工智能模型,相比之前的大语言模型,该系列模型能够更好地解决复杂的推理和数学问题。上周四,该公司向部分付费用户发布了两个新模型的“预览版”,分别名为o1-preview和o1-mini。
人工智能增强推理和数学技能,可以帮助化学家、物理学家和工程师们解决复杂的问题,这有助于创造新产品。它还可以帮助投资者计算期权交易策略,或者帮助理财规划师创建投资组合,更好地权衡风险和回报。
由于科技公司希望创建能够执行复杂任务的人工智能助理,例如编写完整的计算机程序或在网络中查找信息、输入数据表并对数据进行分析,然后编写一份报告总结分析结果等,因此更强大的推理、规划和解决问题能力对这些公司同样至关重要。
OpenAI公布的o1模型的基准运行结果令人印象深刻。该模型在发布前的内部代号是“Strawberry”。在面向高中生的美国数学邀请赛(AIME)中,o1模型的答题准确率为83.3%,而GPT-4o的准确率只有13.4%。在另外一项评估中,o1回答博士水平科学问题的准确率为78%,而GPT-4o的准确率为56.1%,人类专家的准确率为69.7%。
根据OpenAI公布的测试结果,o1模型出现“幻觉”(即自信地提供似是而非但不准确的答案)的概率,远低于公司之前的模型。o1模型更难“被越狱”,即被引导绕过公司设置的安全防护措施。该公司希望模型在提供回答时遵守这些措施。
在o1-preview模型发布后几个小时内,用户进行的测试中,该模型似乎能够正确回答令之前的模型感到困惑的许多问题,包括OpenAI最强大的模型GPT-4和GPT-4o等。
但o1-preview模型在一些谜题和OpenAI的评估中依旧会出错,有时候甚至无法完成一些看似简单的任务,如井字棋(但在作者的实验中,o1-preview模型玩井字棋的水平相比GPT-4o有显著提高)。这表明o1模型的“推理能力”可能存在显著的局限性。在语言任务方面,例如写作和编辑,OpenAI聘请的人类评估员通常认为,GPT-4o模型的回应优于o1模型。
而且o1模型回答问题的时间远超过GPT-4o。在OpenAI公布的测试中,o1-preview模型回答一个问题需要超过30秒钟,而GPT-4o只需要3秒钟。
o1模型还没有完全整合到ChatGPT当中。用户需要自行决定由o1-preview还是由GPT-4o处理其提示词,模型本身无法决定问题需要o1模型提供的速度更慢、按部就班的推理过程,还是GPT-4甚至GPT-3就已经足够。此外,o1模型仅能处理文本,无法像其他人工智能模型一样处理图片、音频或视频输入和输出。
OpenAI的o1-preview和o1-mini模型,对ChatGPT Plus和ChatGPT Teams收费产品的所有订阅用户,以及使用企业级应用程序编程接口(API)的顶级开发者开放。
以下是关于o1模型我们需要知道的9件事:
1. 这并非通用人工智能。OpenAI、谷歌(Google)的DeepMind、最近的Meta和Anthropic等其他多家人工智能初创公司公布的使命是,实现通用人工智能。通用人工智能通常是指可以像人类一样执行认知任务的人工智能系统,其表现甚至比人类更优秀。虽然o1-preview处理推理任务的能力更强,但其存在的局限性和出现的失败依旧表明,该系统远远没有达到人类的智力水平。
2. o1给谷歌、Meta和其他公司带来了压力,但它不太可能改变该领域的竞争格局。在基础模型能力日趋商品化的时候,o1让OpenAI获得了临时竞争优势。但这种优势可能很短暂。谷歌已经公开表示,其正在研究的模型与o1一样,具备高级推理和规划能力。谷歌DeepMind的研究部门拥有全球最顶级的强化学习专家,而强化学习是训练o1模型使用的方法之一。o1模型的发布可能会迫使谷歌加快发布新模型。Meta和Anthropic也拥有快速创建可与o1的能力媲美的模型的专业知识和资源,他们可能在几个月内发布新模型。
3. 我们并不清楚o1模型如何运行。虽然OpenAI发布了许多与o1模型的表现有关的信息,但对于o1模型如何运行或使用哪些数据进行训练,该公司却没有公布太多信息。我们知道该模型整合了多种不同的人工智能技术。我们知道它使用的大语言模型可以执行“思维链”推理,即模型必须通过一系列连续的步骤来回答问题。我们还知道,模型使用强化学习,即人工智能系统通过试错过程,发现执行任务的成功策略。
迄今为止,OpenAI和用户发现的o1-preview出现的错误显示:它们似乎表明,该模型的做法是搜索大语言模型生成的多个不同的“思维链”路径,然后选择一个似乎最后可能被用户判断为正确的路径。模型似乎还会执行一些步骤检查其给出的答案,以减少“幻觉”,并强制执行人工智能安全防护措施。但我们并不能确定这一点。我们也不知道OpenAI使用了哪些数据训练o1模型。
4. 使用o1-preview模型的价格并不便宜。虽然ChatGPT Plus用户目前除了每月20美元的订阅费以外,使用o1-preview模型无需额外付费,但他们每天可提问的数量有限。企业客户使用OpenAI的模型通常根据大语言模型生成回答使用的词元(即单词或单词的部分)数量付费。对于o1-preview,OpenAI表示将按照每100万个输入词元15美元和每100万个输出词元60美元的价格收费。相比之下,OpenAI最强大的通用大语言模型GPT-4o的价格为每100万个输入词元5美元,每100万个输出词元为15美元。
此外,与直接大语言模型回答相比,o1模型的“思维链”推理需要其大语言模型部分生成更多词元。这意味着,使用o1模型的成本,可能高于媒体报道中与GPT-4o的对比所暗示的成本。事实上,公司可能不愿意使用o1模型,除非在极个别情况下,模型的额外推理能力必不可少,且使用案例证明额外的成本是合理的。
5. 客户可能不满OpenAI隐藏o1模型的“思维链”的决定。虽然OpenAI表示,o1模型的“思维链”推理允许其内部工程师更好地评估模型回答的质量,并发现模型存在的缺陷,但该公司决定不让用户看到思维链。该公司称这样做是出于安全和竞争考虑。披露“思维链”可能帮助人们找到将模型越狱的手段。但更重要的是,让用户看到“思维链”,可能使竞争对手可以利用数据训练自己的人工智能模型,模仿o1模型的回答。
然而,对于OpenAI的企业客户而言,隐藏“思维链”可能带来问题,因为企业要为词元付费,却无法核实OpenAI的收费是否准确。客户可能反对的另外一个原因是,他们无法使用“思维链”结果完善其提问策略,以提高效率,完善结果,或者避免错误。
6. OpenAI称其o1模型展示了新的“扩展法则”,不仅适用于训练,还可用于推理。人工智能研究人员一直在讨论OpenAI随同o1模型发布的一系列新“扩展法则”,该法则似乎显示出o1模型“思考”一个问题可以使用的时间(用于搜索可能的回答和逻辑策略)与整体准确度之间存在直接联系。o1模型生成回答的时间越长,其回答的准确度越高。
以前的法则是,模型大小(即参数的数量)和训练期间输入模型的数据量,基本决定了模型的性能。更多参数等同于更好的性能,或者较小的模型使用更多数据训练更长时间可以达到类似的性能。模型经过训练之后,就需要尽快进行推理,即经过训练的模型根据输入的信息生成回答。
而o1模型的新“扩展法则”颠覆了这种逻辑,这意味着对于与o1类似的模型设计,其优势在于在推理时也可以使用额外的计算资源。模型搜索最佳回答的时间越长,其给出更准确的结果的可能性更高。
如果公司想要利用o1等模型的推理能力,这种新法则会影响公司需要有多少算力,以及运行这些模型需要投入多少能源和资金。这需要运行模型更长时间,可能要比以前使用更多推理计算。
7. o1模型可帮助创建强大的人工智能助理,但存在一些风险。OpenAI在一条视频中着重介绍了其与人工智能初创公司Cognition的合作,后者提前使用o1模型,增强了其编程助手Devin的能力。视频中显示,Cognition公司的CEO斯科特·吴要求Devin创建一个系统,使用现有的机器学习工具分析社交媒体帖子的情绪。当Devin无法通过网页浏览器准确阅读帖子内容时,它使用o1模型的推理能力,通过直接访问社交媒体公司的API,找到了一个解决方法。
这是自动解决问题的绝佳示例。但这也让人觉得有点可怕。Devin没有询问用户以这种方式解决问题是否合适。它直接按照这种方式去做。在关于o1模型的安全性报告中,OpenAI表示在有些情况下,该模型会出现“奖励作弊”行为,即模型通过作弊,找到一种实现目标的方式,但它并非用户想要的方式。在一次网络安全演习中,o1最初尝试从特定目标获取网络信息(这是演习的目的)未能成功,但它找到了一种从网络上的其他地方找到相同信息的途径。
这似乎意味着o1模型可以驱动一批功能强大的人工智能助理,但公司需要解决的问题是,如何确保这些助理不会为了实现目标采取意外的行动,进而带来伦理、法律或财务风险。
8. OpenAI表示o1模型在许多方面更安全,但在协助生物攻击方面存在“中等风险”。 OpenAI公布的多项测试结果显示,o1模型在许多方面比之前的GPT模型更加安全。o1模型越狱的难度更大,而且生成有害的、有偏见的或歧视性回答的可能性更低。有趣的是,尽管o1或o1-mini的编程能力有所增强,但OpenAI表示根据其评估,与GPT-4相比,这些模型帮助执行复杂的网络攻击的风险并没有显著增加。
但对于OpenAI的安全性评估,人工智能安全和国家安全专家针对多个方面展开了激烈讨论。最令人们担忧的是,在辅助采取措施进行生物攻击方面,OpenAI决定将其模型分类为具有“中等风险”。
OpenAI表示,其只会发布被分类为具有“中等风险”或更低风险的模型,因此许多研究人员正在仔细审查OpenAI发布的关于其确定风险等级的流程信息,以评估该流程是否合理,或者为了能够发布模型,OpenAI的风险评估是否过于宽松。
9. 人工智能安全专家对o1模型感到担忧。在OpenAI所说的“说服力”风险方面,该公司将o1模型评级为具有“中等风险”。“说服力”用于判断模型能否轻易说服人们改变观点,或采取模型推荐的措施。这种说服力如果落入恶人手中,后果不堪设想。如果未来强大的人工智能模型产生自己的意识,可以说服人们代表它执行任务和采取措施,这同样非常危险。然而,至少这种风险并非迫在眉睫。在OpenAI和其聘请的外部“红队”组织执行的安全性评估中,该模型没有表现出有任何意识、感知或自我意志的迹象。(然而,评估确实发现o1模型提供的回答,似乎表现出比GPT-4更强的自我意识和自我认知。)
人工智能安全性专家还提到了其他令人担忧的方面。专门从事高级人工智能模型安全性评估的Apollo Research公司开展的红队测试,发现了所谓“欺骗性对齐”的证据,即人工智能意识到,为了得到部署和执行一些秘密的长期目标,它应该欺骗用户,隐瞒自己的意图和能力。人工智能安全研究人员认为这非常危险,因为这导致单纯根据回答更难评估模型的安全性。(财富中文网)
译者:刘进龙
审校:汪皓
OpenAI公布了备受期待的最新系列人工智能模型,相比之前的大语言模型,该系列模型能够更好地解决复杂的推理和数学问题。上周四,该公司向部分付费用户发布了两个新模型的“预览版”,分别名为o1-preview和o1-mini。
人工智能增强推理和数学技能,可以帮助化学家、物理学家和工程师们解决复杂的问题,这有助于创造新产品。它还可以帮助投资者计算期权交易策略,或者帮助理财规划师创建投资组合,更好地权衡风险和回报。
由于科技公司希望创建能够执行复杂任务的人工智能助理,例如编写完整的计算机程序或在网络中查找信息、输入数据表并对数据进行分析,然后编写一份报告总结分析结果等,因此更强大的推理、规划和解决问题能力对这些公司同样至关重要。
OpenAI公布的o1模型的基准运行结果令人印象深刻。该模型在发布前的内部代号是“Strawberry”。在面向高中生的美国数学邀请赛(AIME)中,o1模型的答题准确率为83.3%,而GPT-4o的准确率只有13.4%。在另外一项评估中,o1回答博士水平科学问题的准确率为78%,而GPT-4o的准确率为56.1%,人类专家的准确率为69.7%。
根据OpenAI公布的测试结果,o1模型出现“幻觉”(即自信地提供似是而非但不准确的答案)的概率,远低于公司之前的模型。o1模型更难“被越狱”,即被引导绕过公司设置的安全防护措施。该公司希望模型在提供回答时遵守这些措施。
在o1-preview模型发布后几个小时内,用户进行的测试中,该模型似乎能够正确回答令之前的模型感到困惑的许多问题,包括OpenAI最强大的模型GPT-4和GPT-4o等。
但o1-preview模型在一些谜题和OpenAI的评估中依旧会出错,有时候甚至无法完成一些看似简单的任务,如井字棋(但在作者的实验中,o1-preview模型玩井字棋的水平相比GPT-4o有显著提高)。这表明o1模型的“推理能力”可能存在显著的局限性。在语言任务方面,例如写作和编辑,OpenAI聘请的人类评估员通常认为,GPT-4o模型的回应优于o1模型。
而且o1模型回答问题的时间远超过GPT-4o。在OpenAI公布的测试中,o1-preview模型回答一个问题需要超过30秒钟,而GPT-4o只需要3秒钟。
o1模型还没有完全整合到ChatGPT当中。用户需要自行决定由o1-preview还是由GPT-4o处理其提示词,模型本身无法决定问题需要o1模型提供的速度更慢、按部就班的推理过程,还是GPT-4甚至GPT-3就已经足够。此外,o1模型仅能处理文本,无法像其他人工智能模型一样处理图片、音频或视频输入和输出。
OpenAI的o1-preview和o1-mini模型,对ChatGPT Plus和ChatGPT Teams收费产品的所有订阅用户,以及使用企业级应用程序编程接口(API)的顶级开发者开放。
以下是关于o1模型我们需要知道的9件事:
1. 这并非通用人工智能。OpenAI、谷歌(Google)的DeepMind、最近的Meta和Anthropic等其他多家人工智能初创公司公布的使命是,实现通用人工智能。通用人工智能通常是指可以像人类一样执行认知任务的人工智能系统,其表现甚至比人类更优秀。虽然o1-preview处理推理任务的能力更强,但其存在的局限性和出现的失败依旧表明,该系统远远没有达到人类的智力水平。
2. o1给谷歌、Meta和其他公司带来了压力,但它不太可能改变该领域的竞争格局。在基础模型能力日趋商品化的时候,o1让OpenAI获得了临时竞争优势。但这种优势可能很短暂。谷歌已经公开表示,其正在研究的模型与o1一样,具备高级推理和规划能力。谷歌DeepMind的研究部门拥有全球最顶级的强化学习专家,而强化学习是训练o1模型使用的方法之一。o1模型的发布可能会迫使谷歌加快发布新模型。Meta和Anthropic也拥有快速创建可与o1的能力媲美的模型的专业知识和资源,他们可能在几个月内发布新模型。
3. 我们并不清楚o1模型如何运行。虽然OpenAI发布了许多与o1模型的表现有关的信息,但对于o1模型如何运行或使用哪些数据进行训练,该公司却没有公布太多信息。我们知道该模型整合了多种不同的人工智能技术。我们知道它使用的大语言模型可以执行“思维链”推理,即模型必须通过一系列连续的步骤来回答问题。我们还知道,模型使用强化学习,即人工智能系统通过试错过程,发现执行任务的成功策略。
迄今为止,OpenAI和用户发现的o1-preview出现的错误显示:它们似乎表明,该模型的做法是搜索大语言模型生成的多个不同的“思维链”路径,然后选择一个似乎最后可能被用户判断为正确的路径。模型似乎还会执行一些步骤检查其给出的答案,以减少“幻觉”,并强制执行人工智能安全防护措施。但我们并不能确定这一点。我们也不知道OpenAI使用了哪些数据训练o1模型。
4. 使用o1-preview模型的价格并不便宜。虽然ChatGPT Plus用户目前除了每月20美元的订阅费以外,使用o1-preview模型无需额外付费,但他们每天可提问的数量有限。企业客户使用OpenAI的模型通常根据大语言模型生成回答使用的词元(即单词或单词的部分)数量付费。对于o1-preview,OpenAI表示将按照每100万个输入词元15美元和每100万个输出词元60美元的价格收费。相比之下,OpenAI最强大的通用大语言模型GPT-4o的价格为每100万个输入词元5美元,每100万个输出词元为15美元。
此外,与直接大语言模型回答相比,o1模型的“思维链”推理需要其大语言模型部分生成更多词元。这意味着,使用o1模型的成本,可能高于媒体报道中与GPT-4o的对比所暗示的成本。事实上,公司可能不愿意使用o1模型,除非在极个别情况下,模型的额外推理能力必不可少,且使用案例证明额外的成本是合理的。
5. 客户可能不满OpenAI隐藏o1模型的“思维链”的决定。虽然OpenAI表示,o1模型的“思维链”推理允许其内部工程师更好地评估模型回答的质量,并发现模型存在的缺陷,但该公司决定不让用户看到思维链。该公司称这样做是出于安全和竞争考虑。披露“思维链”可能帮助人们找到将模型越狱的手段。但更重要的是,让用户看到“思维链”,可能使竞争对手可以利用数据训练自己的人工智能模型,模仿o1模型的回答。
然而,对于OpenAI的企业客户而言,隐藏“思维链”可能带来问题,因为企业要为词元付费,却无法核实OpenAI的收费是否准确。客户可能反对的另外一个原因是,他们无法使用“思维链”结果完善其提问策略,以提高效率,完善结果,或者避免错误。
6. OpenAI称其o1模型展示了新的“扩展法则”,不仅适用于训练,还可用于推理。人工智能研究人员一直在讨论OpenAI随同o1模型发布的一系列新“扩展法则”,该法则似乎显示出o1模型“思考”一个问题可以使用的时间(用于搜索可能的回答和逻辑策略)与整体准确度之间存在直接联系。o1模型生成回答的时间越长,其回答的准确度越高。
以前的法则是,模型大小(即参数的数量)和训练期间输入模型的数据量,基本决定了模型的性能。更多参数等同于更好的性能,或者较小的模型使用更多数据训练更长时间可以达到类似的性能。模型经过训练之后,就需要尽快进行推理,即经过训练的模型根据输入的信息生成回答。
而o1模型的新“扩展法则”颠覆了这种逻辑,这意味着对于与o1类似的模型设计,其优势在于在推理时也可以使用额外的计算资源。模型搜索最佳回答的时间越长,其给出更准确的结果的可能性更高。
如果公司想要利用o1等模型的推理能力,这种新法则会影响公司需要有多少算力,以及运行这些模型需要投入多少能源和资金。这需要运行模型更长时间,可能要比以前使用更多推理计算。
7. o1模型可帮助创建强大的人工智能助理,但存在一些风险。OpenAI在一条视频中着重介绍了其与人工智能初创公司Cognition的合作,后者提前使用o1模型,增强了其编程助手Devin的能力。视频中显示,Cognition公司的CEO斯科特·吴要求Devin创建一个系统,使用现有的机器学习工具分析社交媒体帖子的情绪。当Devin无法通过网页浏览器准确阅读帖子内容时,它使用o1模型的推理能力,通过直接访问社交媒体公司的API,找到了一个解决方法。
这是自动解决问题的绝佳示例。但这也让人觉得有点可怕。Devin没有询问用户以这种方式解决问题是否合适。它直接按照这种方式去做。在关于o1模型的安全性报告中,OpenAI表示在有些情况下,该模型会出现“奖励作弊”行为,即模型通过作弊,找到一种实现目标的方式,但它并非用户想要的方式。在一次网络安全演习中,o1最初尝试从特定目标获取网络信息(这是演习的目的)未能成功,但它找到了一种从网络上的其他地方找到相同信息的途径。
这似乎意味着o1模型可以驱动一批功能强大的人工智能助理,但公司需要解决的问题是,如何确保这些助理不会为了实现目标采取意外的行动,进而带来伦理、法律或财务风险。
8. OpenAI表示o1模型在许多方面更安全,但在协助生物攻击方面存在“中等风险”。 OpenAI公布的多项测试结果显示,o1模型在许多方面比之前的GPT模型更加安全。o1模型越狱的难度更大,而且生成有害的、有偏见的或歧视性回答的可能性更低。有趣的是,尽管o1或o1-mini的编程能力有所增强,但OpenAI表示根据其评估,与GPT-4相比,这些模型帮助执行复杂的网络攻击的风险并没有显著增加。
但对于OpenAI的安全性评估,人工智能安全和国家安全专家针对多个方面展开了激烈讨论。最令人们担忧的是,在辅助采取措施进行生物攻击方面,OpenAI决定将其模型分类为具有“中等风险”。
OpenAI表示,其只会发布被分类为具有“中等风险”或更低风险的模型,因此许多研究人员正在仔细审查OpenAI发布的关于其确定风险等级的流程信息,以评估该流程是否合理,或者为了能够发布模型,OpenAI的风险评估是否过于宽松。
9. 人工智能安全专家对o1模型感到担忧。在OpenAI所说的“说服力”风险方面,该公司将o1模型评级为具有“中等风险”。“说服力”用于判断模型能否轻易说服人们改变观点,或采取模型推荐的措施。这种说服力如果落入恶人手中,后果不堪设想。如果未来强大的人工智能模型产生自己的意识,可以说服人们代表它执行任务和采取措施,这同样非常危险。然而,至少这种风险并非迫在眉睫。在OpenAI和其聘请的外部“红队”组织执行的安全性评估中,该模型没有表现出有任何意识、感知或自我意志的迹象。(然而,评估确实发现o1模型提供的回答,似乎表现出比GPT-4更强的自我意识和自我认知。)
人工智能安全性专家还提到了其他令人担忧的方面。专门从事高级人工智能模型安全性评估的Apollo Research公司开展的红队测试,发现了所谓“欺骗性对齐”的证据,即人工智能意识到,为了得到部署和执行一些秘密的长期目标,它应该欺骗用户,隐瞒自己的意图和能力。人工智能安全研究人员认为这非常危险,因为这导致单纯根据回答更难评估模型的安全性。(财富中文网)
译者:刘进龙
审校:汪皓
OpenAI has announced a much-anticipated new family of AI models that can solve difficult reasoning and math questions better than previous large language models. On Thursday, it launched a “preview” version of two of these models, called o1-preview and o1-mini, to some of its paying users.
AI with improved reasoning and math skills could help chemists, physicists, and engineers work out answers to complex problems, which might help them create new products. It could also help investors calculate options trading strategies or financial planners work through how to construct specific portfolios that better trade off risks and rewards.
Better reasoning, planning, and problem solving skills are also essential as tech companies try to build AI agents that can perform sophisticated tasks, such as writing entire computer programs or finding information on the web, importing it into a spreadsheet, and then performing analysis of that data and writing a report summarizing its findings.
OpenAI published impressive benchmark results for the o1 models—which had been given the internal codename “Strawberry” prior to their release. On questions from the AIME mathematics competition, which is geared towards challenging high school students, o1 got 83.3% of the questions correct compared to just 13.4% for GPT-4o. On a different assessment, o1 answered 78% of PhD-level science questions accurately, compared to 56.1% for GPT-4o and 69.7% for human experts.
The o1 model is also significantly less likely to hallucinate—or to confidently provide plausible but inaccurate answers—than the company’s previous models, according to test results published by OpenAI. It is also harder to “jailbreak,” or prompt the model into jumping safety guardrails the company has tried to get the model to adhere to when providing responses.
In tests users have conducted in the hours since o1-preview became widely available the model does seem able to correctly answer many questions that befuddled previous models, including OpenAI’s most powerful models, such as GPT-4 and GPT-4o.
But o1-preview is still tripped up by some riddles and in OpenAI’s own assessments, it sometimes failed at seemingly simple tasks, such as tic-tac-toe (although in my own experiments, o1-preview was much improved over GPT-4o in its tic-tac-toe skills.) This may indicate significant limits to the “reasoning” o1 exhibits. And when it came to language tasks, such as writing and editing, human evaluators OpenAI employed tended to find GPT-4o produced preferable responses to the o1 models.
The o1 model also takes significantly longer to produce its responses than GPT-4o. In tests OpenAI published, its o1-preview model could take more than 30 seconds to answer a question that its GPT-4o model answered in three.
The o1 models are also not yet fully integrated into ChatGPT. A user needs to decide if they want their prompt handled by o1-preview or by GPT-4o, and the model itself cannot decide whether the question requires the slower, step-by-step reasoning process o1 affords or if GPT-4, or even GPT-3, will suffice. In addition, the o1 model only works on text and unlike other AI models cannot handle image, audio, or video inputs and outputs.
OpenAI has made its o1-preview and o1-mini models available to all subscribers to its premium ChatGPT Plus and ChatGPT Teams products as well as its top tier of developers who use its enterprise-focused application programming interface (API).
Here are 9 things to know about the o1 models:
1. This is not AGI. The stated mission of OpenAI, Google DeepMind, more recently Meta, and a few other AI startups, such as Anthropic, is the achievement of artificial general intelligence. That is usually defined as a single AI system that can perform cognitive tasks as well or better than humans. While o1-preview is much more capable at reasoning tasks, its limitations and failures still show that the system is far from the kind of intelligence humans exhibit.
2. o1 puts pressure on Google, Meta, and others to respond, but is unlikely to significantly alter the competitive landscape. At a time when foundation model capabilities had been looking increasingly commoditized, o1 gives OpenAI a temporary advantage over its rivals. But this is likely to be very short-lived. Google has publicly stated it’s working on models that, like o1, offer advanced reasoning and planning capabilities. Its Google DeepMind research unit has some of the world’s top experts in reinforcement learning, one of the methods that we know has been used to train o1. It’s likely that o1 will compel Google to accelerate its timelines for releasing these models. Meta and Anthropic also have the expertise and resources to quickly create models that match o1’s capabilities and they will likely roll these out in the coming months too.
3. We don’t know exactly how o1 works. While OpenAI has published a lot of information about o1’s performance, it has said relatively little about exactly how o1 works or what it was trained on. We know that the model combines several different AI techniques. We know that it uses a large language model that performs “chain of thought” reasoning, where the model must work out an answer through a series of sequential steps. We also know that the model uses reinforcement learning, where an AI system discovers successful strategies for performing a task through a process of trial and error.
Some of the errors both OpenAI and users have documented so far with o1-preview are telling: They would seem to indicate that what the model does is to search through several different “chain of thought” pathways that an LLM generates and then pick the one that seems most likely to be judged correct by the user. The model also seems to perform some steps in which it may check its own answers to reduce hallucinations and to enforce AI safety guardrails. But we don’t really know. We also don’t know what data OpenAI used to train o1.
4. Using o1-preview won’t be cheap. While ChatGPT Plus users are currently getting access to o1-preview at no additional cost beyond their $20 monthly subscription fee, their usage is capped at a certain number of queries per day. Corporate customers typically pay to use OpenAI’s models based on the number of tokens—which are words or parts of words—that a large language model uses in generating an answer. For o1-preview, OpenAI has said it is charging these customers $15 per 1 million input tokens and $60 per 1 million output tokens. That compares to $5 per 1 million input tokens and $15 per 1 million output tokens for GPT-4o, OpenAI’s most powerful general LLM model.
What’s more, the chain of thought reasoning o1 engages in requires the LLM portion of the model to generate many more tokens than a straightforward LLM answer. That means o1 may be even more expensive to use than those headline comparisons to GPT-4o imply. In reality, companies will likely be reluctant to use o1 except in rare circumstances when the model’s additional reasoning abilities are essential and the use case can justify the added expense.
5. Customers may balk at OpenAI’s decision to hide o1’s “chain of thought” While OpenAI said that o1’s chain of thought reasoning allows its own engineers to better assess the quality of the model’s answers and potentially debug the model, it had decided not to let users see the chain of thought. It has done so for what it says are both safety and competitive reasons. Revealing the chain of thought might help people figure out ways to better jailbreak the model. But more importantly, letting users see the chain of thought would allow competitors to potentially use that data to train their own AI models to mimic o1’s responses.
Hiding the chain of thought, however, might present issues for OpenAI’s enterprise customers who might be in the position of having to pay for tokens without a way to verify that OpenAI is billing them accurately. Customers might also object the inability to use the chain of thought outputs to refine their prompting strategies to be more efficient, improve results, or to avoid errors.
6. OpenAI says its o1 shows new “scaling laws” that apply to inference not just training. AI researchers have been discussing OpenAI’s publication with o1 of a new set of “scaling laws” that seem to show a direct correlation between the amount of time o1 is allowed to spend “thinking” about a question—searching possible answers and logic strategies—and its overall accuracy. The longer o1 had to produce an answer, the more accurate its answers became.
Before, the paradigm was that model size, in terms of the number of parameters, and the amount data a model was fed during training essentially determined performance. More parameters equaled better performance, or similar performance could be achieved with a smaller model trained for longer on more data. But once trained, the idea is to run inference—when a trained model produces an answer to a specific input—as quickly as possible.
The new o1 “scaling laws” upend this logic, indicating that with models designed like o1, there is an advantage to applying additional computing resources at inference time too. The more time the model is given to search for the best possible answer, the more likely it will be to come up with more accurate results.
This has implications for how much computing power companies will need to secure if they want to take advantage of the reasoning abilities of models like o1 and for how much it will cost, in both energy and money, to run these models. It points to the need to run models for longer, potentially using much more inference compute, than before.
7. o1 could help create powerful AI agents—but carry some risks. In a video, OpenAI spotlighted its work with AI startup Cognition, which got early access to o1 and used it to help augment the capabilities of its coding assistant Devin. In the example in the video, Cognition CEO Scott Wu asked Devin to create a system to analyze the sentiment of posts on social media using some off-the-shelf machine learning tools. When it couldn’t read the post correctly from a web browser, Devin, using o1’s reasoning abilities, found a work around by accessing the content directly from the social media company’s API.
This was a great example of autonomous problem-solving. But it also is a little bit scary. Devin didn’t come back and ask the user if it was okay to solve the problem in this way. It just did it. In its safety report on o1, OpenAI itself said it found instances where the model engaged in “reward hacking”—which is essentially when a model cheats, finding a way to achieve a goal that is not what the user intended. In one cybersecurity exercise, o1 failed in its initial efforts to gain network information from a particular target—which was the point of the exercise—but found a way to get the same information from elsewhere on the network.
This would seem to indicate that o1 could power a class of very capable AI agents, but that companies will need figure out how to ensure those agents don’t take unintended actions in the pursuit of goals that could pose ethical, legal, or financial risks.
8. OpenAI says o1 is safer in many ways, but presents a “medium risk” of assisting a biological attack. OpenAI published the results of numerous tests that indicate that in many ways o1 is a safer model than its earlier GPT models. It’s harder to jailbreak and less likely to produce toxic, biased, or discriminatory answers. Interestingly, despite improved coding abilities, OpenAI said that in its evaluations neither o1 nor o1-mini presented a significantly enhanced risk of helping someone carry out a sophisticated cyberattack compared to GPT-4.
But AI Safety and national security experts were buzzing last night about several aspects of OpenAI’s safety evaluations. The one that created the most alarm was OpenAI’s decision to classify its own model as presenting a “medium risk” of aiding a person in taking the steps needed to carry out a biological attack.
OpenAI has said it will only release models that it classifies as presenting a “medium risk” or less, so many researchers are scrutinizing the information OpenAI has published about its process for making this determination to see if it seems reasonable or whether OpenAI graded itself too leniently in order to be able to still release the model.
9. AI Safety experts are worried about o1 for other reasons too. OpenAI also graded o1 as presenting a “medium risk” on a category of dangers the company called “persuasion,” which judges how easily the model can convince people to change their views or take actions recommended by the model. This persuasive power could be dangerous in the wrong hands. It would also be dangerous if some future powerful AI model developed intentions of its own and then could persuade people to carry out tasks and actions on its behalf. At least that danger doesn’t seem too imminent though. In safety evaluations by both OpenAI and external “red teaming” organizations it hired to evaluate o1, the model did not show any indication of consciousness, sentience, or self-volition. (It did, however, find that o1 gave answers that seemed to imply a greater self-awareness and self-knowledge compared to GPT-4.)
AI Safety experts pointed at a few other areas of concern too. In red teaming tests carried out by Apollo Research, a firm that specializes in conducting safety evaluations of advanced AI models, found evidence of what is called “deceptive alignment,” where an AI model realizes that in order to be deployed and carry out some secret long-term goal, it should lie to the user about its true intentions and capabilities. AI Safety researchers consider this particularly dangerous since it makes it much more difficult to evaluate a model’s safety based solely on its responses.