借助“深度学习”人工智能,进一步了解自己的肌肉
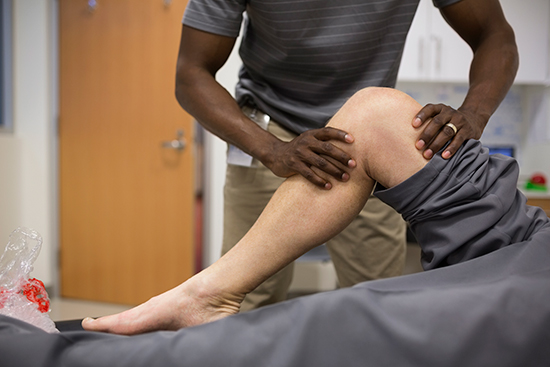
俗话说,眼见为实。慢性病的治愈过程其实很难看见,努力练习跑更快跳更远的进步往往也不明显。 但是,如果有人工智能帮忙呢? 根据医学期刊《IEEE Transactions on Medical Imaging》新发表的一项研究,位于大阪附近的奈良科学技术学院(Nara Institute of Science and Technology)的研究人员称,已经开发出“深度学习”人工智能工具,能够更好地区分单块肌肉,更快速准确搭建个人肌肉骨骼系统的模型。医学专家可以使用该模型研究人体肌肉和骨骼的力量及承受的压力。 主导该项研究的奈良科学技术学院的教授佐藤义雄在一份声明中说:“细分很耗时,而且需要专业知识。我们使用深度学习技术自动细分单独的肌肉,为每位患者生成个性化的肌肉骨骼模型。” “深度学习”是人工智能研究领域的术语,主要利用所谓的神经网络和庞大的计算能力,通过实例学习并模仿人类学习的方式。 在研究过程中,奈良科学技术学院的研究人员利用工具观察人体大腿和臀部的19块肌肉,主要看细分肌肉时能否超过传统的成像方法(包括公认最新技术的分层多图谱分割)。最后新工具成功了,也缩短了外科医生调试及验证系统的时间。 该研究由研究员们与大阪大学医院合作进行。 该工具潜在的应用方式众多。一方面能够让医疗服务提供方开发更有效的康复设备,帮助患有肌萎缩侧索硬化(亦被称为ALS)及其它导致肌肉严重萎缩疾病的患者。另一方面,全球的顶级运动员也可以借此更好地了解自身生物力学。 本次突破只是个性化医疗广阔领域的众多突破之一,由研究人员在名叫Bayesian U-Net的深度学习框架上完成。个性化医疗通常使用个人数据为特定患者量身定制治疗方案,不再选择针对普通人群的常规治疗。 如果利用该工具审查医学图像,将来会不会替代行医经验丰富的骨科医生?可能性不大。但该工具也许可以减少骨科医生看图像的时间,留出更多的时间实施诊治。(财富中文网) 译者:冯丰 审校:夏林 |
As the saying goes, seeing is believing—sometimes in a cure for a chronic disease, sometimes in the opportunity to run faster or jump farther than ever before. But what if artificial intelligence can assist? According to a newly published study in the medical journal IEEE Transactions on Medical Imaging, researchers at the Nara Institute of Science and Technology, known as NAIST and located outside of Osaka, say they have developed a “deep learning” A.I. tool that allows them to better tell apart, or “segment,” individual muscles. The tool ultimately allows for the faster creation of a more accurate model of a person’s musculoskeletal system, which medical professionals then use to study the forces and stresses on muscles and bones. “This segmentation was time consuming and depended on expert-knowledge,” said Yoshinobu Sato, the NAIST professor who led the study, in a statement. “We used deep learning to automate the segmentation of individual muscles to generate a musculoskeletal model that is personalized to the patient.” “Deep learning” is the term for the area of A.I. research that uses so-called neural networks—and a tremendous amount of computational horsepower—to learn by example and mimic the way humans learn. For the study, the NAIST researchers directed the tool at 19 muscles in the thigh and hips to see if it could tell them apart better than conventional imaging methods, including hierarchical multi-atlas segmentation, considered state-of-the-art. It succeeded, even as it reduced the time a surgeon needs to train and validate the system. Researchers conducted the study in collaboration with Osaka University Hospital. The potential applications of the tool are numerous. It can help those who suffer from amyotrophic lateral sclerosis, known as ALS, and other disorders that result in severe muscle atrophy by allowing medical providers to develop more effective rehabilitation devices. It can also help the world’s top athletes who want to better understand their biomechanics. The advancement, which the researchers built on a deep learning framework known as Bayesian U-Net, is just one of many in the broad area of health care known as personalized medicine, which involves using personal data to tailor treatment to the specific patient, rather than opting for conventional treatment that best targets the average population. Will the tool replace the highly skilled orthopedic surgeons once needed to examine such medical images? It’s not likely. But it might just let them spend less time seeing and more time doing. |