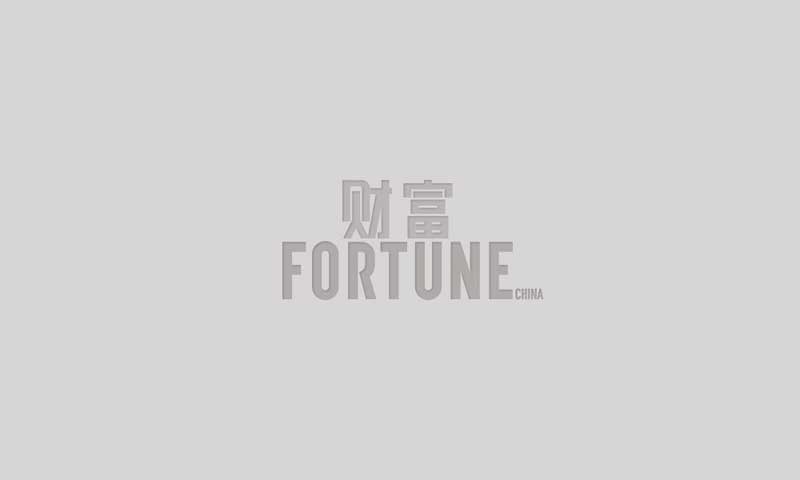
DeepSeek让开源再度流行起来。这家中国初创企业决定使用开源框架来实现复杂推理,这一举动震动了人工智能生态系统:自此之后,百度将文心一言(ERNIE)模型开源,而 OpenAI 首席执行官山姆·奥特曼(Sam Altman)也表示,他认为自己领导的非开源公司可能站在了“历史错误的一边”。
目前人工智能领域存在两种截然不同的范式:一种是由OpenAI和微软(Microsoft)等巨头推动的闭源生态系统,另一种是由Meta和Mistral等公司倡导的开源平台。
这不仅仅是一场技术层面的争论。开源与闭源之争,是关于人工智能未来走向的根本性讨论,也是在万亿美元规模的人工智能行业逐步成型之际,关于谁将掌控这项新技术巨大潜力的讨论。
历史教训
每一场软件革命的核心都是开源系统与闭源系统之间的较量。
在大型机时代,IBM及其闭源系统占据了主导地位,以至于流传着这样一句格言:“选IBM永远不会被炒鱿鱼。”但随着技术的成熟,企业纷纷转向开源系统,从而摆脱了供应商的限制。
这样的循环一次又一次地上演。开源的Linux系统向微软的Windows系统发起了挑战。PostgreSQL和MySQL数据库成为了甲骨文数据库的替代品。
供应商锁定,即更换供应商几乎不可能,会抑制创新、限制灵活性并带来安全隐患。随着人工智能日益深入地融入关键业务流程,这些风险只会进一步加剧。
开源平台则能够降低这些风险,使企业能够更换供应商或将解决方案进行内部化调整,而无需承担高昂的成本。
开源为何重要
消费者可能会体验到闭源平台带来的便利。然而,企业优先考虑的因素则有所不同。企业不能通过它们无法掌控的黑盒应用程序编程接口(API)传输敏感数据和专有信息。
开源人工智能模型具有三个关键优势。
首先,开源模型将敏感信息保存在企业内部的基础设施之中,降低了因与外部服务器交互而导致数据泄露的风险。
其次,企业能够根据自身独特需求对开源模型进行定制化开发,利用专有数据对模型进行微调,而不受闭源系统的限制。
最后,通过在自有基础设施上部署开源模型,企业能够避免向供应商支付因规模扩展而产生的额外费用。
尽管闭源平台可能在操作上更为简便,但它们却无法提供开源模型所具备的安全性、灵活性以及低成本优势。
颇具讽刺意味的是,OpenAI的崛起是建立在开源的基础之上的。2017年谷歌发布的《注意力就是你所需要的一切》为现代语言模型的发展提供了蓝图。然而,尽管有此基础,OpenAI 却从初创时的开源理念转向了更加倾向于闭源的发展模式,这引发了人们对其致力于确保人工智能惠及“全人类”这一承诺的质疑。
微软与OpenAI的合作使这家科技巨头迅速跻身商业人工智能领域的前沿。微软投入了超过130亿美元,将GPT-4集成到其整个生态系统中——从Azure云服务到通过Copilot、GitHub和必应(Bing)集成到Office应用程序中——这对依赖这些工具的企业产生了强大的锁定效应。
从历史上看,闭源人工智能系统一直通过强力策略占据主导地位:扩大数据规模、增加参数数量以及提升算力,从而主导市场并设置进入壁垒。
然而,一种新的模式正在兴起:推理能力革命。像DeepSeek的R1这样的模型表明,复杂推理能力已然能够与依赖规模优势的专有系统相抗衡。推理能力是开源人工智能的特洛伊木马,它证明了算法的进步能够削弱闭源平台的优势,从而对竞争格局构成挑战。
这为小型实验室和初创企业带来了重要机遇。开源人工智能以远低于闭源系统的成本促进集体创新的发展,实现了资源获取的民主化,并鼓励更多参与者做出贡献。
目前,传统的人工智能价值链由少数几家公司主导,包括硬件领域的英伟达(Nvidia)、模型开发领域的OpenAI和Anthropic,以及基础设施领域的亚马逊云科技(Amazon Web Services)、微软Azure和谷歌云平台(Google Cloud Platform)。由于对资金和算力要求较高,形成了极高的进入壁垒。
但是,像优化推理引擎和专用硬件这样的新型创新正在逐步瓦解这一垄断格局。
在这个新的生态系统中,人工智能堆栈正在经历拆分。像Groq这样的公司正在硬件领域向英伟达发起挑战(Groq是Race Capital投资组合中的公司之一)。像Mistral这样的小型实验室开发出了极具智能的模型,能够与OpenAI和Anthropic抗衡。像Hugging Face这样的平台致力于实现模型获取的民主化。像Fireworks和Together这样的推理服务正在降低延迟并提高请求处理量。像Lambda Labs和Fluidstack这样的替代云市场,提供了竞争性价格优势(与三大寡头相比)。
平衡开源与闭源
诚然,开源模型也有自身的风险。训练数据存在被滥用的可能性。恶意行为者可能会开发出有害的应用程序,比如恶意软件或深度伪造技术。企业也可能会越过道德底线,擅自使用个人数据,牺牲数据隐私以谋取竞争优势。
采取战略性治理措施有助于缓解上述风险。推迟发布前沿模型能够为安全评估工作留出时间。实施部分权重共享策略也能在带来研究益处的同时,限制被滥用的风险。
人工智能的未来取决于平衡这些相互冲突的利益诉求的能力——这就如同人工智能系统本身为了实现最佳性能而平衡权重和偏差一样。
开源与闭源的抉择,绝非仅仅基于个人喜好,而是决定人工智能革命走向的关键决策。我们必须选择能够鼓励创新、促进包容性且合乎道德的治理框架。开源将是实现这一目标的途径。
庄思浩(Alfred Chuang)是Race Capital的普通合伙人,该公司在人工智能领域进行全面布局,涵盖开源和闭源解决方案。(财富中文网)
译者:中慧言-王芳
DeepSeek让开源再度流行起来。这家中国初创企业决定使用开源框架来实现复杂推理,这一举动震动了人工智能生态系统:自此之后,百度将文心一言(ERNIE)模型开源,而 OpenAI 首席执行官山姆·奥特曼(Sam Altman)也表示,他认为自己领导的非开源公司可能站在了“历史错误的一边”。
目前人工智能领域存在两种截然不同的范式:一种是由OpenAI和微软(Microsoft)等巨头推动的闭源生态系统,另一种是由Meta和Mistral等公司倡导的开源平台。
这不仅仅是一场技术层面的争论。开源与闭源之争,是关于人工智能未来走向的根本性讨论,也是在万亿美元规模的人工智能行业逐步成型之际,关于谁将掌控这项新技术巨大潜力的讨论。
历史教训
每一场软件革命的核心都是开源系统与闭源系统之间的较量。
在大型机时代,IBM及其闭源系统占据了主导地位,以至于流传着这样一句格言:“选IBM永远不会被炒鱿鱼。”但随着技术的成熟,企业纷纷转向开源系统,从而摆脱了供应商的限制。
这样的循环一次又一次地上演。开源的Linux系统向微软的Windows系统发起了挑战。PostgreSQL和MySQL数据库成为了甲骨文数据库的替代品。
供应商锁定,即更换供应商几乎不可能,会抑制创新、限制灵活性并带来安全隐患。随着人工智能日益深入地融入关键业务流程,这些风险只会进一步加剧。
开源平台则能够降低这些风险,使企业能够更换供应商或将解决方案进行内部化调整,而无需承担高昂的成本。
开源为何重要
消费者可能会体验到闭源平台带来的便利。然而,企业优先考虑的因素则有所不同。企业不能通过它们无法掌控的黑盒应用程序编程接口(API)传输敏感数据和专有信息。
开源人工智能模型具有三个关键优势。
首先,开源模型将敏感信息保存在企业内部的基础设施之中,降低了因与外部服务器交互而导致数据泄露的风险。
其次,企业能够根据自身独特需求对开源模型进行定制化开发,利用专有数据对模型进行微调,而不受闭源系统的限制。
最后,通过在自有基础设施上部署开源模型,企业能够避免向供应商支付因规模扩展而产生的额外费用。
尽管闭源平台可能在操作上更为简便,但它们却无法提供开源模型所具备的安全性、灵活性以及低成本优势。
颇具讽刺意味的是,OpenAI的崛起是建立在开源的基础之上的。2017年谷歌发布的《注意力就是你所需要的一切》为现代语言模型的发展提供了蓝图。然而,尽管有此基础,OpenAI 却从初创时的开源理念转向了更加倾向于闭源的发展模式,这引发了人们对其致力于确保人工智能惠及“全人类”这一承诺的质疑。
微软与OpenAI的合作使这家科技巨头迅速跻身商业人工智能领域的前沿。微软投入了超过130亿美元,将GPT-4集成到其整个生态系统中——从Azure云服务到通过Copilot、GitHub和必应(Bing)集成到Office应用程序中——这对依赖这些工具的企业产生了强大的锁定效应。
从历史上看,闭源人工智能系统一直通过强力策略占据主导地位:扩大数据规模、增加参数数量以及提升算力,从而主导市场并设置进入壁垒。
然而,一种新的模式正在兴起:推理能力革命。像DeepSeek的R1这样的模型表明,复杂推理能力已然能够与依赖规模优势的专有系统相抗衡。推理能力是开源人工智能的特洛伊木马,它证明了算法的进步能够削弱闭源平台的优势,从而对竞争格局构成挑战。
这为小型实验室和初创企业带来了重要机遇。开源人工智能以远低于闭源系统的成本促进集体创新的发展,实现了资源获取的民主化,并鼓励更多参与者做出贡献。
目前,传统的人工智能价值链由少数几家公司主导,包括硬件领域的英伟达(Nvidia)、模型开发领域的OpenAI和Anthropic,以及基础设施领域的亚马逊云科技(Amazon Web Services)、微软Azure和谷歌云平台(Google Cloud Platform)。由于对资金和算力要求较高,形成了极高的进入壁垒。
但是,像优化推理引擎和专用硬件这样的新型创新正在逐步瓦解这一垄断格局。
在这个新的生态系统中,人工智能堆栈正在经历拆分。像Groq这样的公司正在硬件领域向英伟达发起挑战(Groq是Race Capital投资组合中的公司之一)。像Mistral这样的小型实验室开发出了极具智能的模型,能够与OpenAI和Anthropic抗衡。像Hugging Face这样的平台致力于实现模型获取的民主化。像Fireworks和Together这样的推理服务正在降低延迟并提高请求处理量。像Lambda Labs和Fluidstack这样的替代云市场,提供了竞争性价格优势(与三大寡头相比)。
平衡开源与闭源
诚然,开源模型也有自身的风险。训练数据存在被滥用的可能性。恶意行为者可能会开发出有害的应用程序,比如恶意软件或深度伪造技术。企业也可能会越过道德底线,擅自使用个人数据,牺牲数据隐私以谋取竞争优势。
采取战略性治理措施有助于缓解上述风险。推迟发布前沿模型能够为安全评估工作留出时间。实施部分权重共享策略也能在带来研究益处的同时,限制被滥用的风险。
人工智能的未来取决于平衡这些相互冲突的利益诉求的能力——这就如同人工智能系统本身为了实现最佳性能而平衡权重和偏差一样。
开源与闭源的抉择,绝非仅仅基于个人喜好,而是决定人工智能革命走向的关键决策。我们必须选择能够鼓励创新、促进包容性且合乎道德的治理框架。开源将是实现这一目标的途径。
庄思浩(Alfred Chuang)是Race Capital的普通合伙人,该公司在人工智能领域进行全面布局,涵盖开源和闭源解决方案。(财富中文网)
译者:中慧言-王芳
DeepSeek has made open-source cool again. The Chinese startup’s decision to use open-source frameworks to achieve sophisticated reasoning has shaken up the AI ecosystem: Since then, Baidu has made its ERNIE model open-source, while OpenAI CEO Sam Altman has said he thinks his non-open source company may be on the “wrong side of history.”
There are now two distinct paradigms in the AI sector: the closed ecosystems promoted by giants like OpenAI and Microsoft, versus the open-source platforms championed by companies like Meta and Mistral.
This is more than just a technical debate. Open vs. closed is a fundamental debate about AI’s future and who will control the new technology’s vast potential as a trillion-dollar industry takes shape.
Lessons from history
Every software revolution has been, at its heart, a struggle between open and closed systems.
In the mainframe era, IBM and its closed system dominated, prompting the aphorism: “Nobody ever got fired for choosing IBM.” But as technology matured, businesses turned to open systems that freed them from vendor constraints.
This cycle happened again and again. Open-source Linux challenged Microsoft Windows. PostgreSQL and MySQL became an alternative to Oracle’s databases.
Vendor lock-in, where switching providers becomes nearly impossible, stifles innovation, limits agility, and creates vulnerability. Those same risks will only increase as AI is increasingly integrated into critical business processes.
Open platforms mitigate those risks, allowing organizations to change vendors or bring solutions in-house without incurring crippling costs.
Why open source matters
Consumers may enjoy the convenience of a closed platform. Yet enterprises have different priorities. Organizations can’t send sensitive data and proprietary information through black box APIs that they don’t control.
Open-source AI models offer three critical advantages.
First, open models keep sensitive information within an organization’s infrastructure, reducing the risk of data breaches from interactions with an external server.
Second, enterprises can tailor open-source models to their unique needs, fine-tuning models with their proprietary data without being constrained by a closed system.
Finally, organizations can avoid scaling fees charged by vendors by deploying open-source models on their own infrastructure.
Closed platforms may be simple, but they don’t provide the safety, flexibility and low costs of an open-source model.
Ironically, OpenAI’s rise was built on open-source foundations. The “Attention Is All You Need” paper released by Google in 2017 provided the blueprint for modern language models. Yet, despite this foundation, OpenAI has shifted from its initial open-source ethos to a more closed model, raising questions about its commitment to ensuring that AI benefits “all of humanity.”
Microsoft’s partnership with OpenAI has rapidly positioned the tech giant at the forefront of the commercial AI landscape. With over $13 billion invested, Microsoft has integrated GPT-4 across its ecosystem—from Azure to Office applications via Copilot, GitHub, and Bing—creating a powerful lock-in effect for businesses that rely on these tools.
Historically, closed AI systems have dominated through brute-force strategies: Scaling data, parameters, and computing power to dominate the market and create barriers to entry.
Yet, a new paradigm is emerging: the reasoning revolution. Models like DeepSeek’s R1 demonstrate that sophisticated reasoning capabilities can rival proprietary systems that depend on sheer scale. Reasoning is a Trojan horse for open-source AI, challenging the competitive landscape by proving that algorithmic advancements can diminish the advantages held by closed platforms.
This opens up a crucial opportunity for smaller labs and startups. Open-source AI fosters collective innovation at a fraction of the cost associated with closed systems, democratizing access and encouraging contributions from a wider range of participants.
Currently, the traditional AI value chain is dominated by a few players in hardware (Nvidia), model development (OpenAI, Anthropic) and infrastructure (Amazon Web Services, Microsoft Azure, Google Cloud Platform). This has created significant barriers to entry, due to high capital and compute requirements.
But new innovations, like optimized inference engines and specialized hardware, are dismantling this monolithic structure.
The AI stack is becoming unbundled in this new ecosystem. Companies like Groq are challenging Nvidia in hardware. (Groq is one of Race Capital’s portfolio companies.) Smaller labs like Mistral have built creative models that can compete with OpenAI and Anthropic. Platforms like Hugging Face are democratizing access to models. Inference services like Fireworks and Together are reducing latency and increasing throughput of requests. Alternative cloud marketplaces, such as Lambda Labs and Fluidstack, offer competitive pricing with the Big Three oligopoly.
Balancing open vs. closed
Of course, open-source models bring their own risks. Training data could be misappropriated. Malicious actors could develop harmful applications, like malware or deepfakes. Companies, too, may cross ethical boundaries by using personal data without authorization, sacrificing data privacy in pursuit of competitive advantage.
Strategic governance measures can help mitigate these risks. Delaying releases of frontier models could give time for security assessments. Partial weight sharing could also limit the potential for misuse, while still providing research benefits.
The future of AI rests on the ability to balance these competing interests—much like how AI systems themselves balance weights and biases for optimal performance.
The choice between going open or closed represents more than just preference. It’s a pivotal decision that will determine the trajectory of the AI revolution. We must choose frameworks that encourage innovation, inclusivity, and ethical governance. Going open-source will be the way to achieve that.
Alfred Chuang is general partner at Race Capital, which invests across the AI spectrum, including both open-source and closed-source solutions.